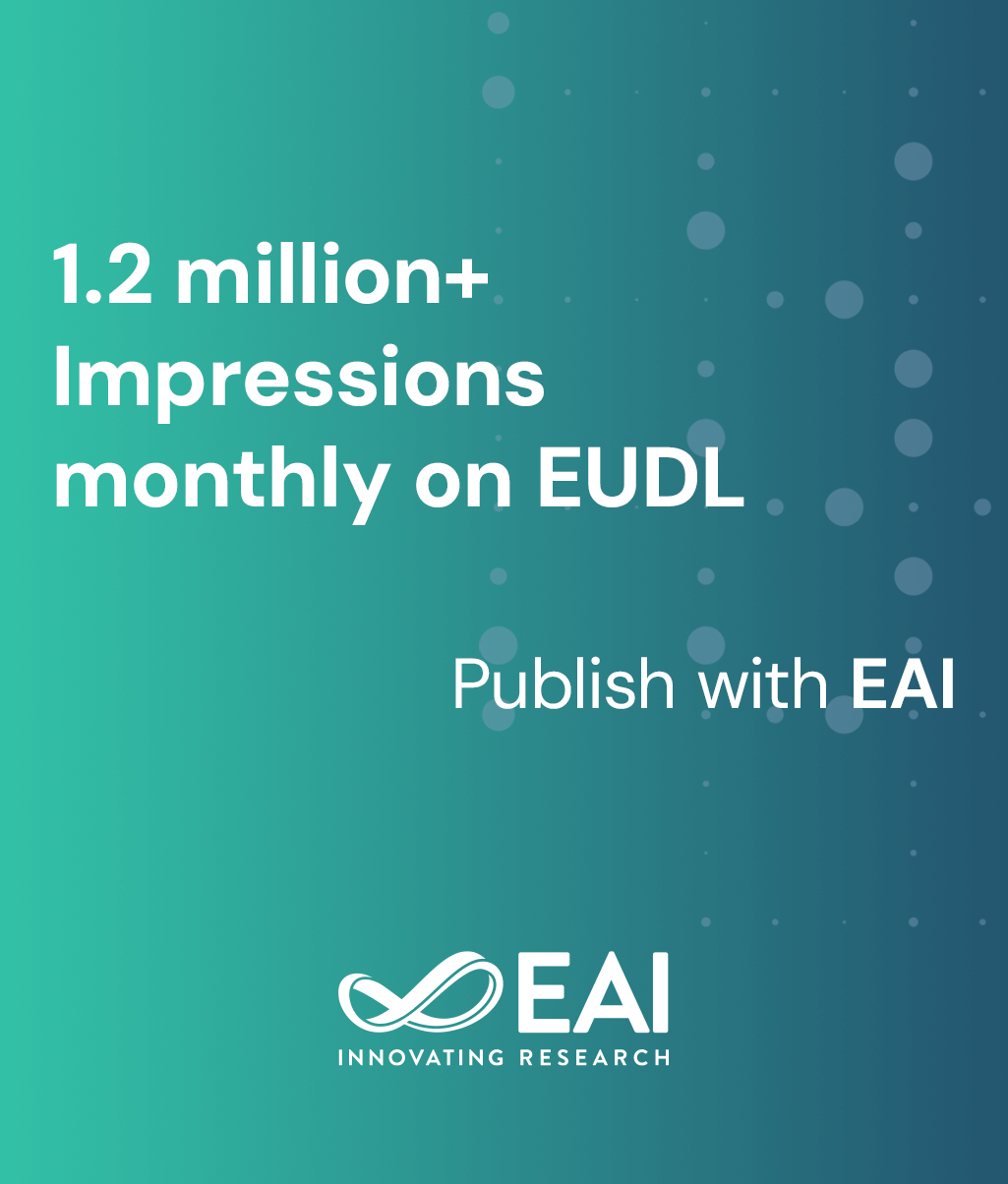
Research Article
Extraction and Reuse of Visual Elements of Tourism Cultural and Creative Products Based on Deep Learning
@INPROCEEDINGS{10.4108/eai.2-12-2022.2328751, author={Zhijie Lin and Xiaobo Lian}, title={Extraction and Reuse of Visual Elements of Tourism Cultural and Creative Products Based on Deep Learning}, proceedings={Proceedings of the 3rd International Conference on Big Data Economy and Information Management, BDEIM 2022, December 2-3, 2022, Zhengzhou, China}, publisher={EAI}, proceedings_a={BDEIM}, year={2023}, month={6}, keywords={deep learning; cultural and creative products; visual elements; paper cutting pattern}, doi={10.4108/eai.2-12-2022.2328751} }
- Zhijie Lin
Xiaobo Lian
Year: 2023
Extraction and Reuse of Visual Elements of Tourism Cultural and Creative Products Based on Deep Learning
BDEIM
EAI
DOI: 10.4108/eai.2-12-2022.2328751
Abstract
With the development of urbanization, people's demand for products continues to extend outward, and the demand for tourism CCP (Cultural and Creative Products) is also increasing. As an important part of traditional culture, traditional handicraft can make products with special artistic value and charm by hand. If the paper-cut patterns can be classified by computer instead of manual, the automation of computer pattern generation can be greatly improved. This paper studies the extraction and re-application of tourism CCP visual ele-ments based on deep learning. The extraction model of visual elements of arbitrary shape paper-cut patterns proposed in this paper consists of two parts: encoding and decoding. The feature sequence is obtained through CNN (Convolutional Neural Network), and then the feature sequence is sent to the bidirectional GRU (gatedcurrent unit) network to extract image features; The decoding part first allocates the structural attributes of the image feature sequence through the attention model, and then outputs the recognized paper-cut patterns through the GRU network. The results show that the geometric features of different types of patterns are quite different, and these features can better distinguish different types of patterns.