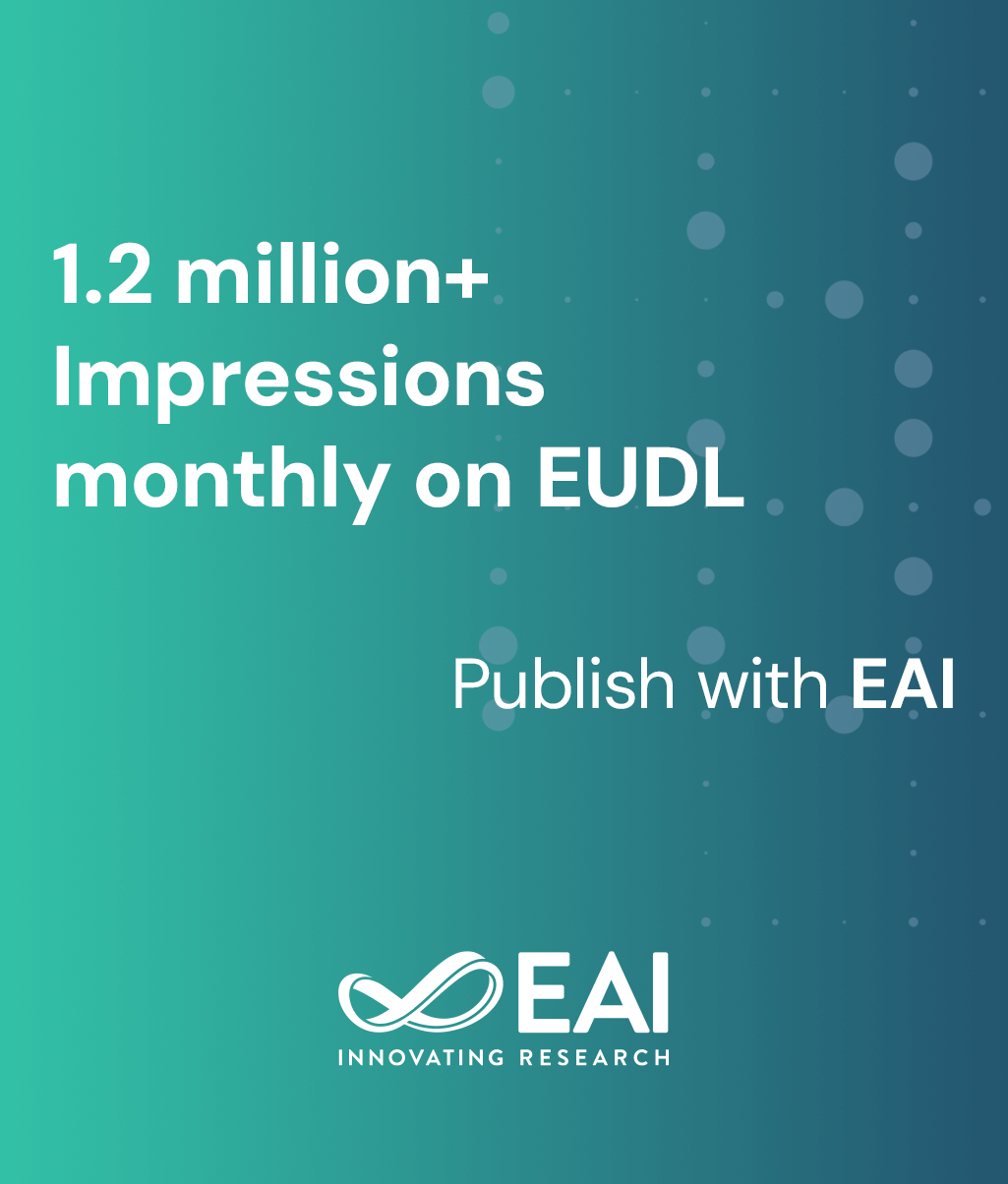
Research Article
Bank Customer Churn Prediction
@INPROCEEDINGS{10.4108/eai.2-12-2022.2328745, author={Hao Tan}, title={Bank Customer Churn Prediction}, proceedings={Proceedings of the 3rd International Conference on Big Data Economy and Information Management, BDEIM 2022, December 2-3, 2022, Zhengzhou, China}, publisher={EAI}, proceedings_a={BDEIM}, year={2023}, month={6}, keywords={random forest logistic regression grid search roc\&auc}, doi={10.4108/eai.2-12-2022.2328745} }
- Hao Tan
Year: 2023
Bank Customer Churn Prediction
BDEIM
EAI
DOI: 10.4108/eai.2-12-2022.2328745
Abstract
Nowadays, with the rapid development of Internet finance, the competition in the banking industry is getting fiercer and fiercer. How to prevent the loss of customers and retain old customers has gradually become an important issue of concern for major banks. In this paper, descriptive statistical analysis of each feature is carried out according to the customer data set of a bank. After a preliminary understanding of the data, data preprocessing is carried out, including data cleaning, feature selection, data transformation, etc. Then, Random Forest and Logistic Regression supervised learning models are used for training and Grid Search is used for reference adjustment. The trained model was evaluated by ROC & AUC, and finally, suggestions were made for the bank to retain customers based on descriptive statistics and the importance of features. Finally, this paper finds that Random Forest is the best model for predicting. At the same time, according to the importance of the model’s influence on bank customer churn, it is concluded that the top three factors with the greatest influence are: age Estimate Salary and Credit Score.