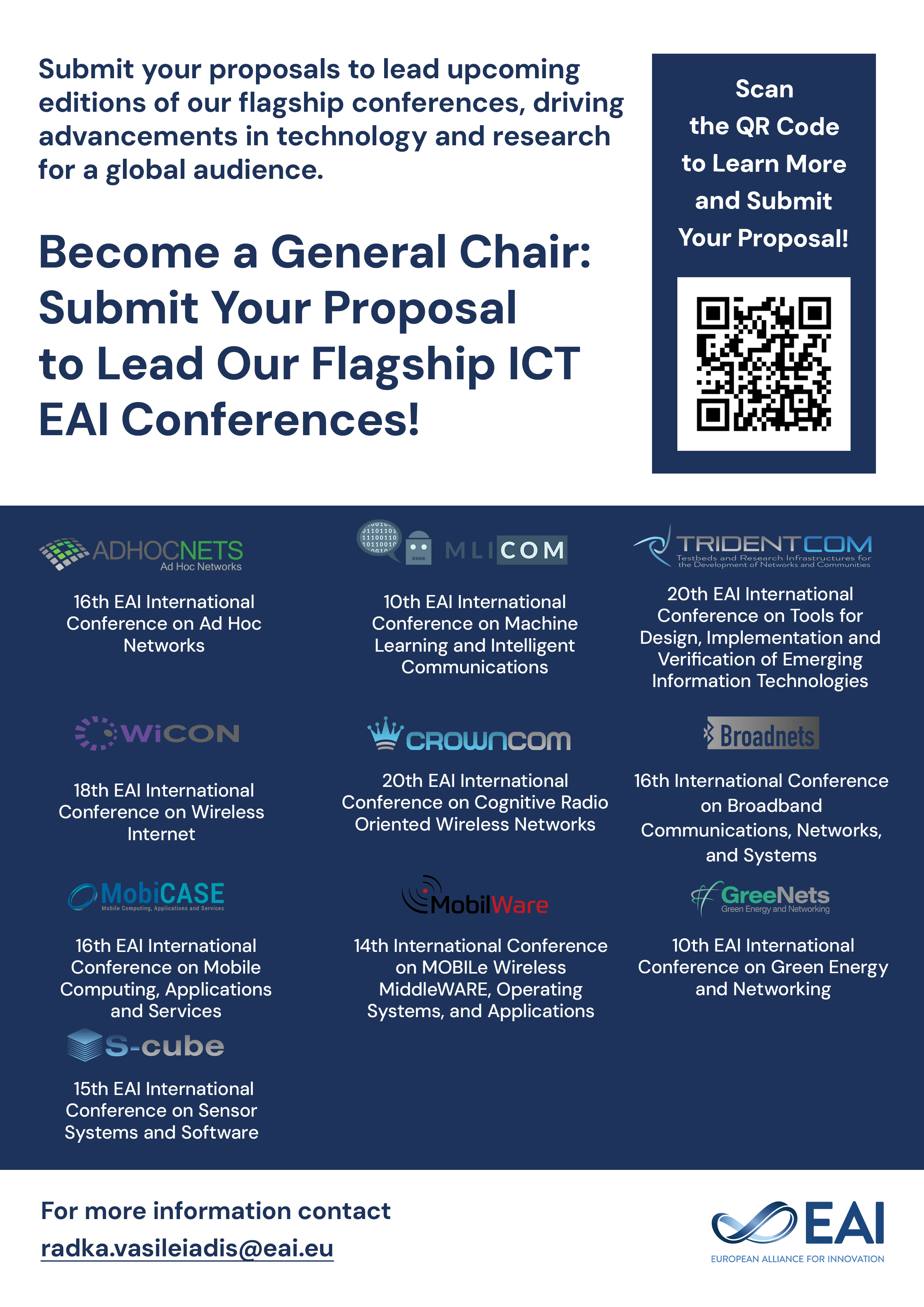
Research Article
Loan Default Prediction Based on Machine Learning Methods
@INPROCEEDINGS{10.4108/eai.2-12-2022.2328740, author={Yuran Zhou}, title={Loan Default Prediction Based on Machine Learning Methods}, proceedings={Proceedings of the 3rd International Conference on Big Data Economy and Information Management, BDEIM 2022, December 2-3, 2022, Zhengzhou, China}, publisher={EAI}, proceedings_a={BDEIM}, year={2023}, month={6}, keywords={loan default prediction; machine learning; extreme gradient boosting tree}, doi={10.4108/eai.2-12-2022.2328740} }
- Yuran Zhou
Year: 2023
Loan Default Prediction Based on Machine Learning Methods
BDEIM
EAI
DOI: 10.4108/eai.2-12-2022.2328740
Abstract
Loan default prediction helps institutions predict whether a borrower will default on a loan and decide whether to lend, thereby reducing losses. We investigate the performance of different machine learning models in predicting customers' loan defaults. Four machine learning models: Logistic Regression, Decision Tree, Random Forest and XGBoost, are used to predict the loan default, considering dependent variables such as the value of all the assets, living status and yearly income. Our results show that XGBoost is the best model with the highest Recall of 0.35 and AUC of 0.832. This study is expected to help lending institutions identify potential default users, and then decide on who to accept or refuse for a loan.
Copyright © 2022–2024 EAI