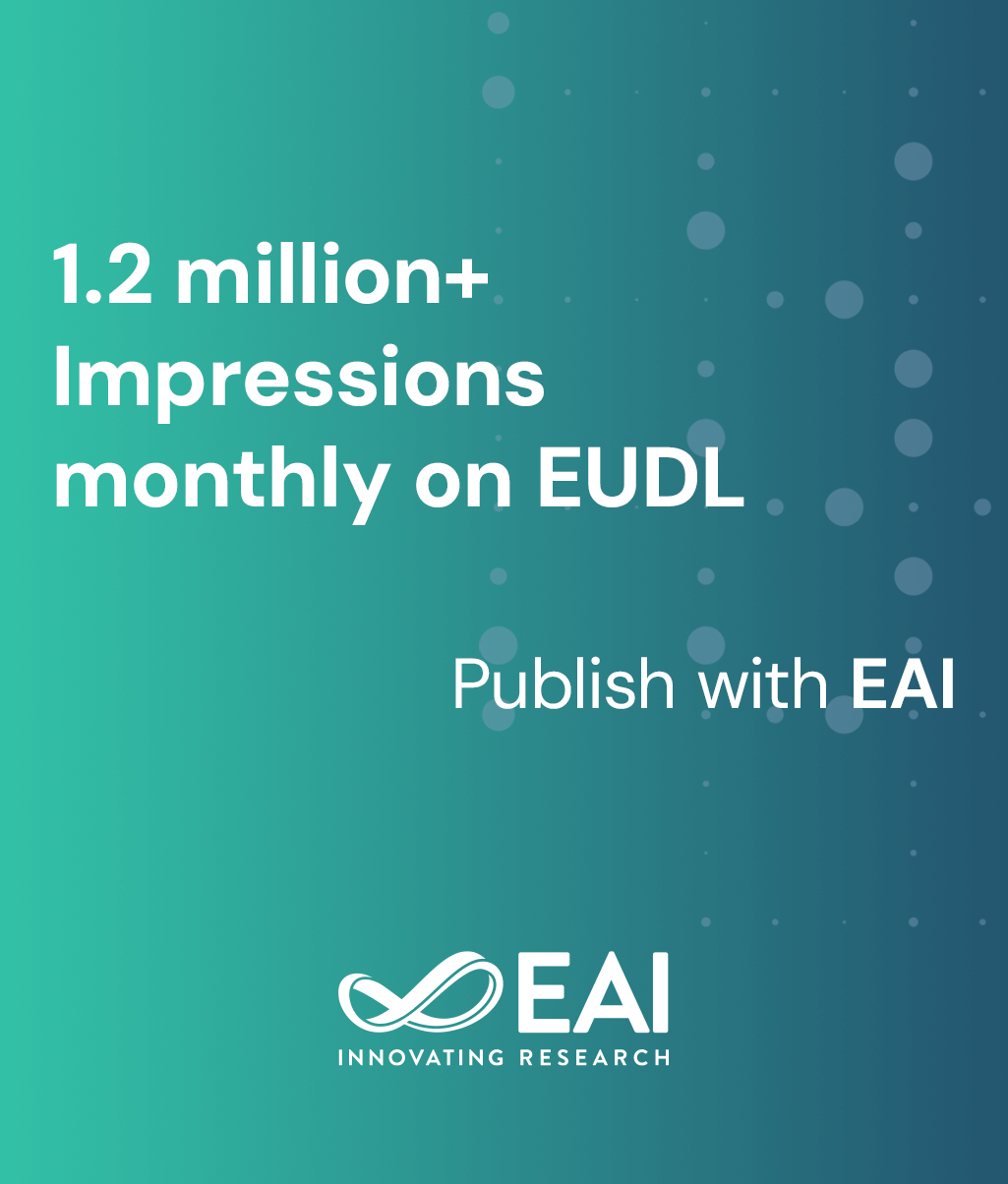
Research Article
Impact of Air Quality on Housing Prices: A Machine Learning Approach
@INPROCEEDINGS{10.4108/eai.2-12-2022.2328696, author={Zulin Feng and Xinlong Li}, title={Impact of Air Quality on Housing Prices: A Machine Learning Approach}, proceedings={Proceedings of the 3rd International Conference on Big Data Economy and Information Management, BDEIM 2022, December 2-3, 2022, Zhengzhou, China}, publisher={EAI}, proceedings_a={BDEIM}, year={2023}, month={6}, keywords={aqi housing price prediction ols regression random forest lasso ridge}, doi={10.4108/eai.2-12-2022.2328696} }
- Zulin Feng
Xinlong Li
Year: 2023
Impact of Air Quality on Housing Prices: A Machine Learning Approach
BDEIM
EAI
DOI: 10.4108/eai.2-12-2022.2328696
Abstract
This paper investigates whether air quality could impact people’s willingness to pay in the housing market. Leveraging OLS regression, Random Forest, Lasso, and Ridge regression, we find a negative correlation between housing prices and air pollution. We use Air Quali-ty Index to measure air quality in our study. Higher AQI represents a higher pollution lev-el. Specifically, one unit increase in AQI reduces the total price of houses by 21370 yuan. Furthermore, our findings reveal that the height of buildings is positively related to resi-dents’ concern about air quality when they make housing purchase decisions. In particular, the higher level the resident’s house locates, the more attention the buyers put pay to air quality. Also, we notice that the buyers from the high total price group are more willing to pay for clean air. As for housing price prediction, we confirm that adding AQI as one of the predictors can increase the accuracy of Beijing housing price prediction even though its effect is relatively small. The findings of this paper may contribute to practical implica-tions in environmental policies, residential satisfaction, and housing market regulations.