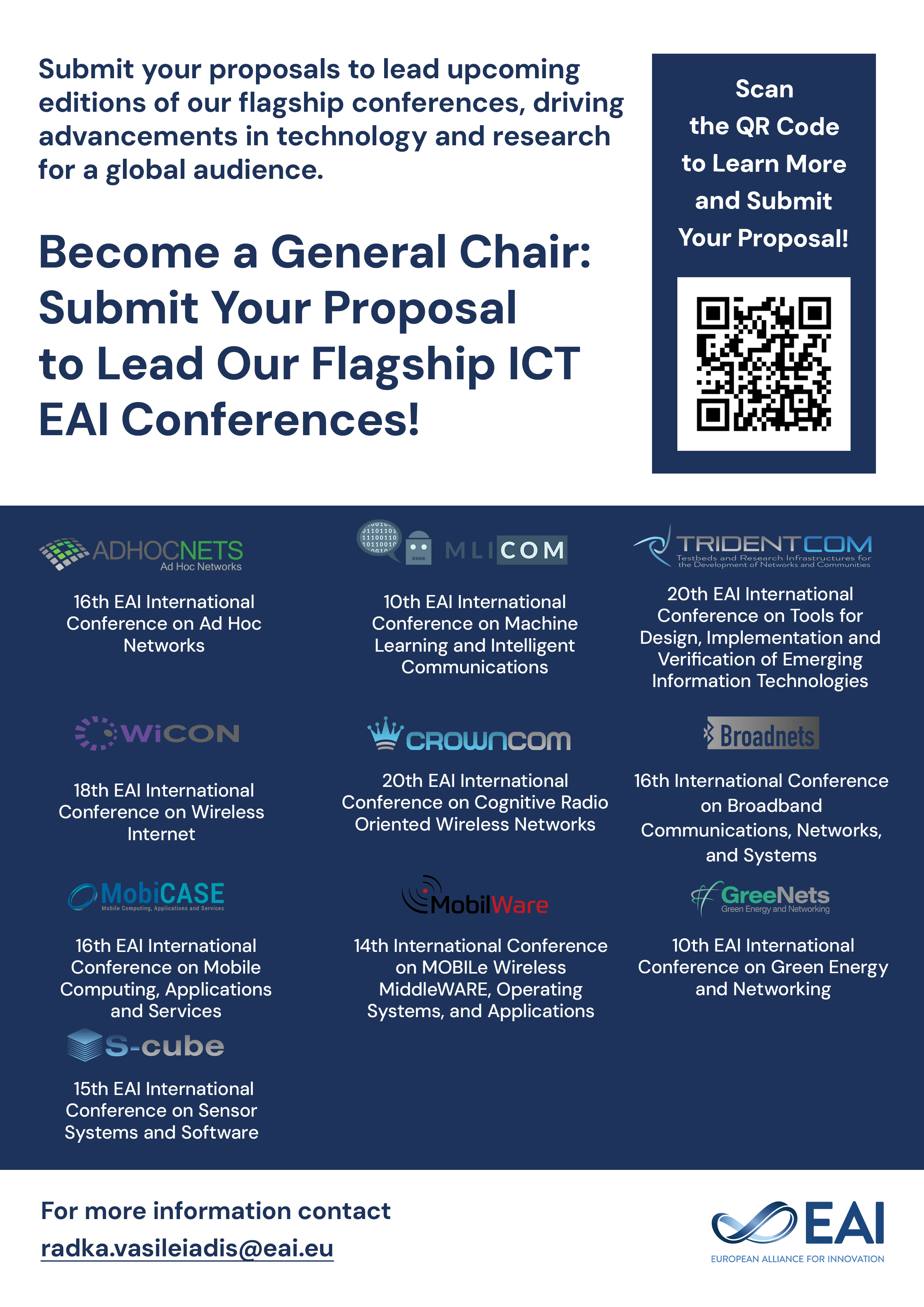
Research Article
Research on the Design of Oral English System Based on Content Recommendation
@INPROCEEDINGS{10.4108/eai.2-12-2022.2328066, author={Hui Li}, title={Research on the Design of Oral English System Based on Content Recommendation}, proceedings={Proceedings of the 2nd International Conference on Information, Control and Automation, ICICA 2022, December 2-4, 2022, Chongqing, China}, publisher={EAI}, proceedings_a={ICICA}, year={2023}, month={3}, keywords={word network; content recommendation; recommendation technology; spoken english system; recommendation principle}, doi={10.4108/eai.2-12-2022.2328066} }
- Hui Li
Year: 2023
Research on the Design of Oral English System Based on Content Recommendation
ICICA
EAI
DOI: 10.4108/eai.2-12-2022.2328066
Abstract
The rapid development of artificial intelligence has made changes in all walks of life. Most of the current English learning platforms use traditional collaborative filtering algorithms to make recommendations. However, students' knowledge is dynamic. Their knowledge is constantly increasing, and traditional collaborative filtering algorithms cannot capture students' knowledge status in time. Therefore, in order to solve this problem, this paper proposes a comprehensive spoken English recommendation system that combines word network and recommendation algorithm. The recommendation of content to users in this system is based on the content recommendation algorithm, which is analyzed in detail in the second part of this paper. The content-based recommendation focuses on calculating the similarity between the product feature vectors, reading the user interest vectors and the product feature vectors, and calculating them based on the similarity calculation formula. The system can dynamically adjust the recommended content as the user's knowledge increases. It can not only improve the learning effect, but also improve the students' learning interest and enthusiasm.