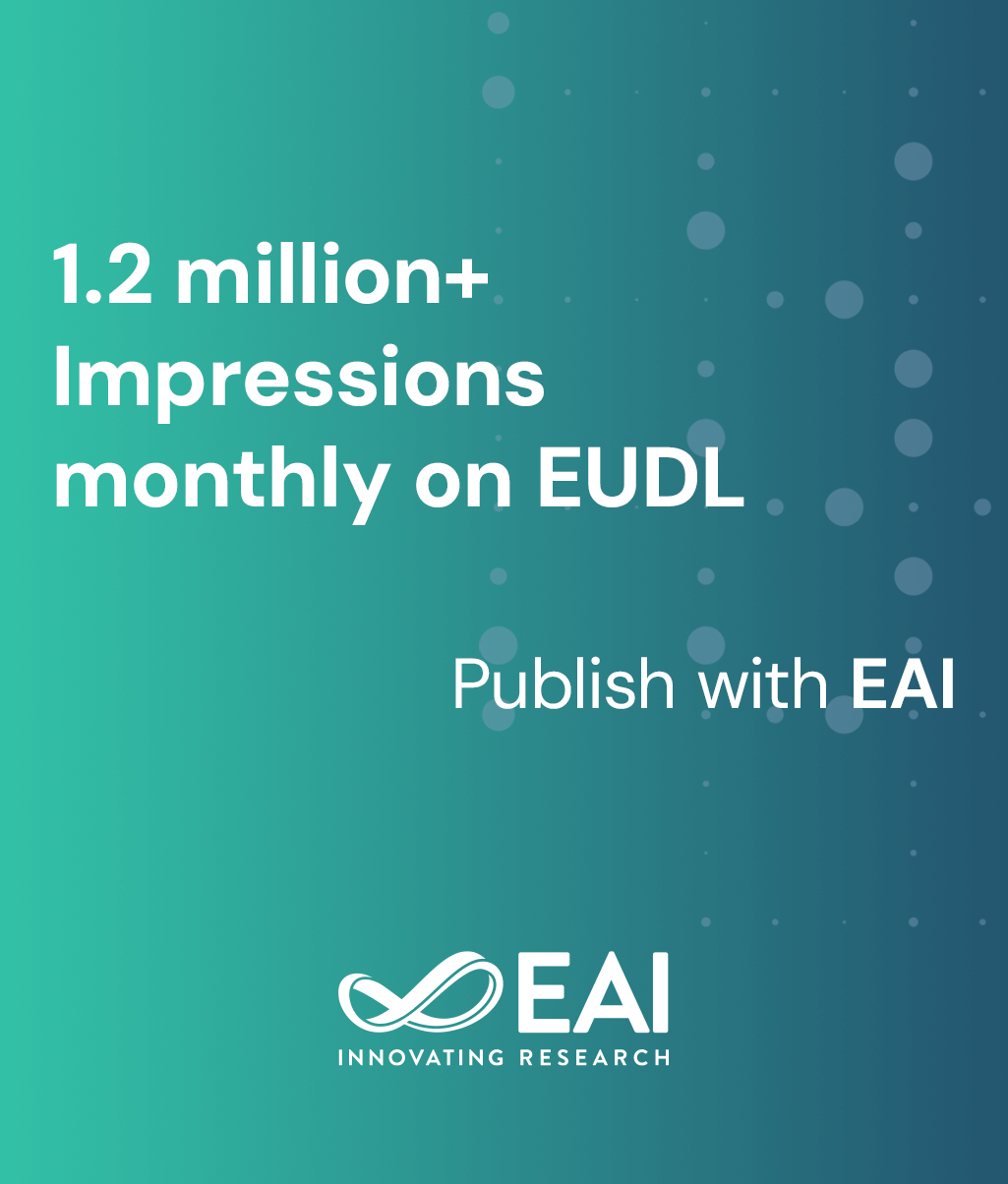
Research Article
Big Data Analysis Method based on Statistical Machine Learning: A Case Study of Financial Data Modeling
@INPROCEEDINGS{10.4108/eai.2-12-2022.2327928, author={Jiaqi Pang}, title={Big Data Analysis Method based on Statistical Machine Learning: A Case Study of Financial Data Modeling}, proceedings={Proceedings of the 2nd International Conference on Information, Control and Automation, ICICA 2022, December 2-4, 2022, Chongqing, China}, publisher={EAI}, proceedings_a={ICICA}, year={2023}, month={3}, keywords={machine learning financial data modeling data analysis modeling}, doi={10.4108/eai.2-12-2022.2327928} }
- Jiaqi Pang
Year: 2023
Big Data Analysis Method based on Statistical Machine Learning: A Case Study of Financial Data Modeling
ICICA
EAI
DOI: 10.4108/eai.2-12-2022.2327928
Abstract
The combination of statistics and machine learning algorithms for big data analysis modeling is an integrated analysis method, which is widely used in data analysis scenarios such as the Internet, finance, etc. It is a hotspot in the current analysis and modeling methodology research. We propose an analytical modeling framework that can integrate statistical models and machine learning models, and applies statistical analysis modeling methods of big data to financial data analysis. We analyzed real financial loan data. The experimental results show that in the application of financial loan default analysis, the random forest algorithm in statistical machine learning works very well. We propose an improved random forest algorithm to accurately and efficiently identify key variables to better judge loan default or not, thus allowing financial institutions to more accurately assess loan risk.