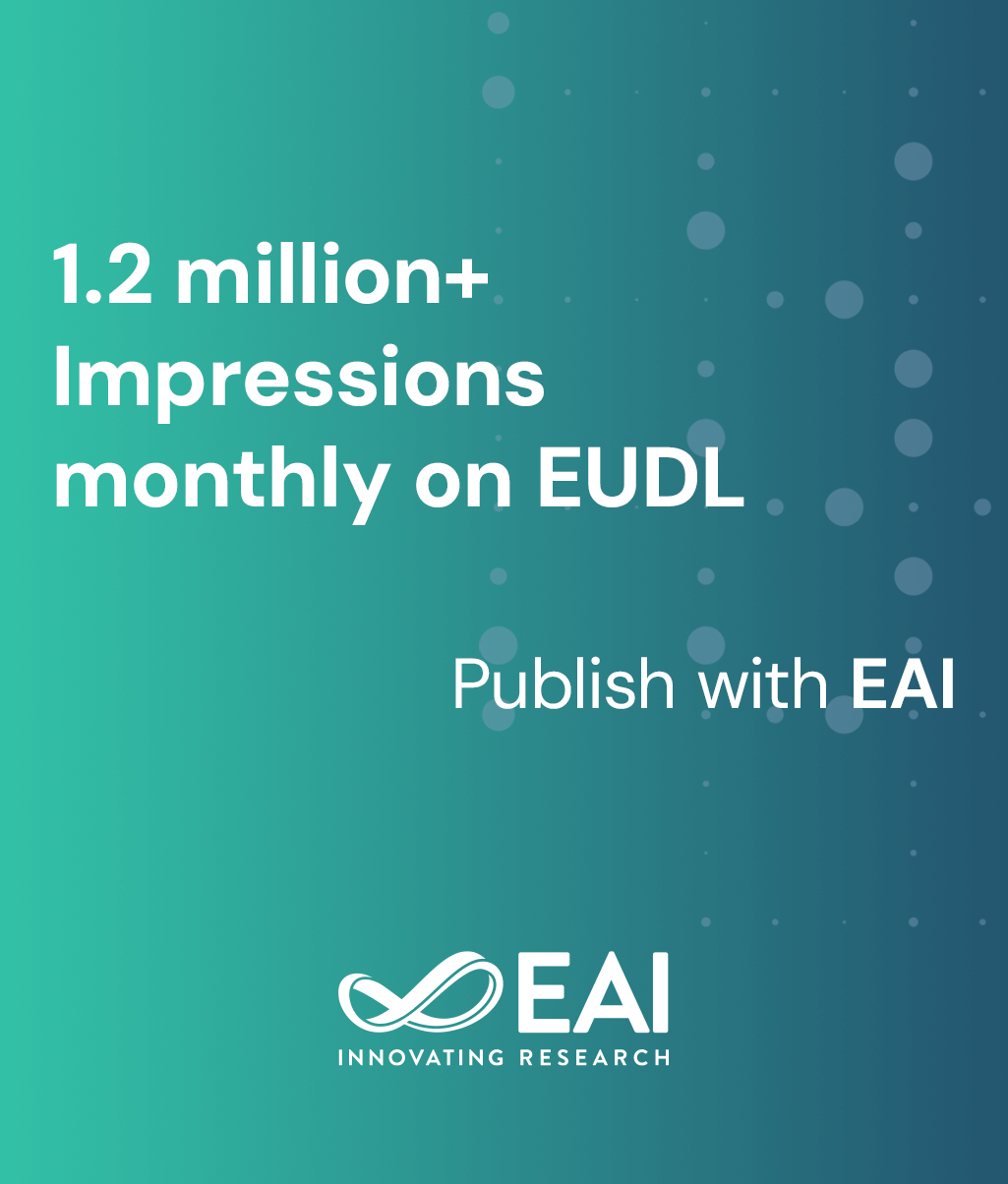
Research Article
Design of Leaf Detection Application using Deep Learning Convolutional Neural Network (CNN) Case Study Lambosir (Blok Leuweung Buah) Mountain Ciremai National Park
@INPROCEEDINGS{10.4108/eai.2-12-2021.2320309, author={Iwan Lesmana and Toni Khalimi and Rachmat Ismaya and Hegar Paningkat Bagja}, title={Design of Leaf Detection Application using Deep Learning Convolutional Neural Network (CNN) Case Study Lambosir (Blok Leuweung Buah) Mountain Ciremai National Park}, proceedings={Proceedings of the 2nd Universitas Kuningan International Conference on System, Engineering, and Technology, UNISET 2021, 2 December 2021, Kuningan, West Java, Indonesia}, publisher={EAI}, proceedings_a={UNISET}, year={2022}, month={8}, keywords={leaf detection; deep learning; cnn}, doi={10.4108/eai.2-12-2021.2320309} }
- Iwan Lesmana
Toni Khalimi
Rachmat Ismaya
Hegar Paningkat Bagja
Year: 2022
Design of Leaf Detection Application using Deep Learning Convolutional Neural Network (CNN) Case Study Lambosir (Blok Leuweung Buah) Mountain Ciremai National Park
UNISET
EAI
DOI: 10.4108/eai.2-12-2021.2320309
Abstract
The Leuweung Buah Lambosir area of Mount Ciremai National Park is included in the rehabilitation zone, the plant diversity index in Lambosir is in the medium category. Increased knowledge and skills regarding the introduction of plant species for officers in Mount Ciremai National Park is often carried out, but for some officers there are some difficulties in identifying plants quickly and accurately. Deep learning is a branch of machine learning (ML) that uses deep neural networks to solve problems in the ML domain. This study aims to create a leaf detection application using a deep learning Convolutional Neural Network (CNN) approach. The types of leaves used in this study were 6 types of leaves including Sonokeling (Dalbergia latifolia Roxb.), Kuray (Trema orientalis), Bungur (Lagerstroemia sp) , Kibeusi (Rhodamnia cinerea), Guava Rivet (Syzygium densiflorum), and Huni (Antidesma Bunius). Tests were carried out with a total of 600 images: 400 images as training data and 200 images as testing data. Testing of each object produces an accuracy rate above 80%.