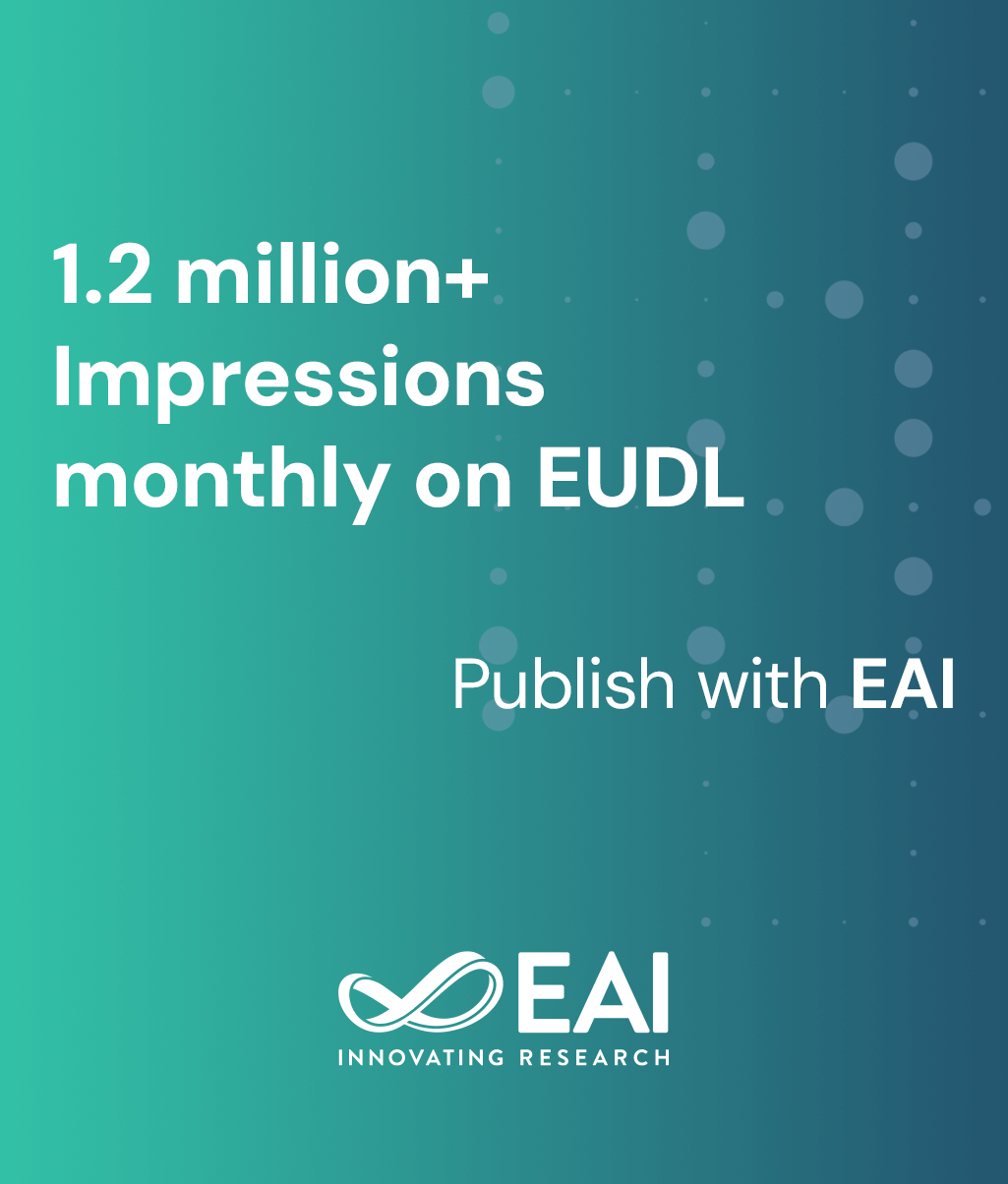
Research Article
Comprehensive Learning Particle Swarm Optimization with Tabu Operator Based on Ripple Neighborhood for Global Optimization
@INPROCEEDINGS{10.4108/eai.19-8-2015.2260857, author={Jin Qi and Bin Xu and Kung Wang and Xi Yin and Xiaoxuan Hu and Yanfei Sun}, title={Comprehensive Learning Particle Swarm Optimization with Tabu Operator Based on Ripple Neighborhood for Global Optimization}, proceedings={11th EAI International Conference on Heterogeneous Networking for Quality, Reliability, Security and Robustness}, publisher={IEEE}, proceedings_a={QSHINE}, year={2015}, month={9}, keywords={comprehensive learning particle swarm optimizer (clpso); tabu search; gaussian distribution; parameter adaptive}, doi={10.4108/eai.19-8-2015.2260857} }
- Jin Qi
Bin Xu
Kung Wang
Xi Yin
Xiaoxuan Hu
Yanfei Sun
Year: 2015
Comprehensive Learning Particle Swarm Optimization with Tabu Operator Based on Ripple Neighborhood for Global Optimization
QSHINE
IEEE
DOI: 10.4108/eai.19-8-2015.2260857
Abstract
For the weak convergence at the latter stage of the comprehensive learning particle swarm optimizer (CLPSO), we put forward a new CLPSO based on Tabu search to enhance the performance. Inspired by the phenomenon of water waves, a Ripple Neighborhood (RP) structure based on the Gaussian distribution is proposed to construct a new adaptive neighborhood structure to guide the selection of candidate solutions in Tabu search, which solves the problem of low convergence and improves the quality of the solution in CLPSO. Experimental results on the standard 26 test functions show that the proposed algorithm achieves a better performance compared with CLPSO.
Copyright © 2015–2025 ICST