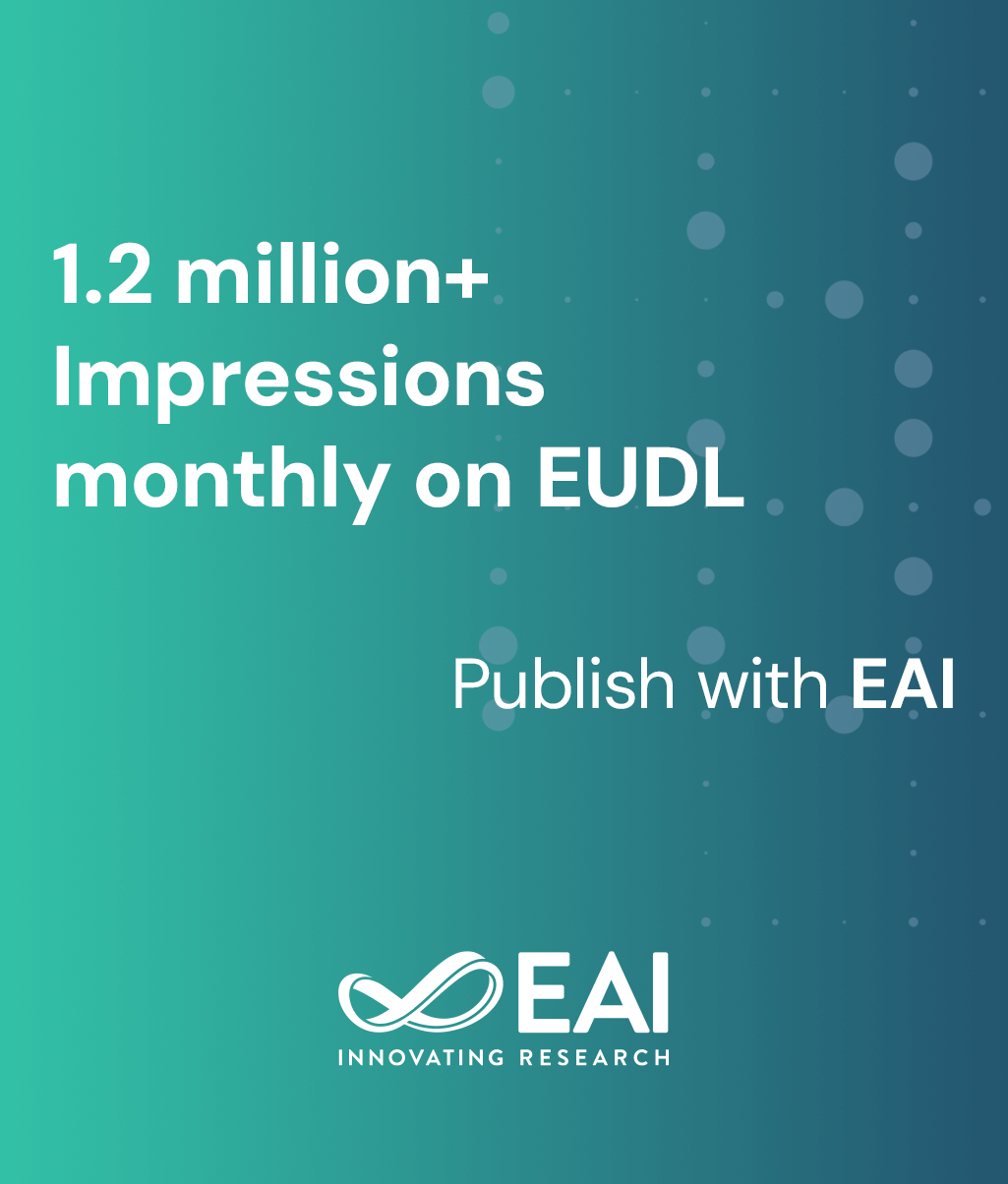
Research Article
Content Linking for UGC based on Word Embedding Model
@ARTICLE{10.4108/eai.19-8-2015.2259690, author={Zhiqiao Gao and Lei Li and Liyuan Mao and Dezhu He and Chao Xue}, title={Content Linking for UGC based on Word Embedding Model}, journal={EAI Endorsed Transactions on Wireless Spectrum}, volume={1}, number={5}, publisher={EAI}, journal_a={WS}, year={2015}, month={9}, keywords={content linking; word embedding model; ugc}, doi={10.4108/eai.19-8-2015.2259690} }
- Zhiqiao Gao
Lei Li
Liyuan Mao
Dezhu He
Chao Xue
Year: 2015
Content Linking for UGC based on Word Embedding Model
WS
EAI
DOI: 10.4108/eai.19-8-2015.2259690
Abstract
There are huge amounts of User Generated Contents (UGCs) consisting of authors’ articles of different themes and readers’ on-line comments on social networks every day. Generally, an article often gives rise to thousands of readers’ comments, which are related to specific points of the originally published article or previous comments. Hence it has suggested the urgent need for automated methods to implement the content linking task, which can also help other related applications, such as information retrieval, summarization and content management. So far content linking is still a relatively new issue. Because of the unsatisfactory of traditional ways based on feature extraction, we look forward to using deeper textual semantic analysis. The Word Embedding model based on deep learning has performed well in Natural Language Processing (NLP), especially in mining deep semantic information recently. Therefore, we study further on the Word Embedding model trained by different neural network models from which we can learn the structure, principles and training ways of the neural network language model in more depth to complete deep semantic feature extraction. With the aid of the semantic features, we expect to do further research on content linking between comments and their original articles from social networks, and finally verify the validity of the proposed method by comparison with traditional ways based on feature extraction.
Copyright © 2015 Z. Gao et al., licensed to EAI. This is an open access article distributed under the terms of the Creative Commons Attribution licence (http://creativecommons.org/licenses/by/3.0/), which permits unlimited use, distribution and reproduction in any medium so long as the original work is properly cited.