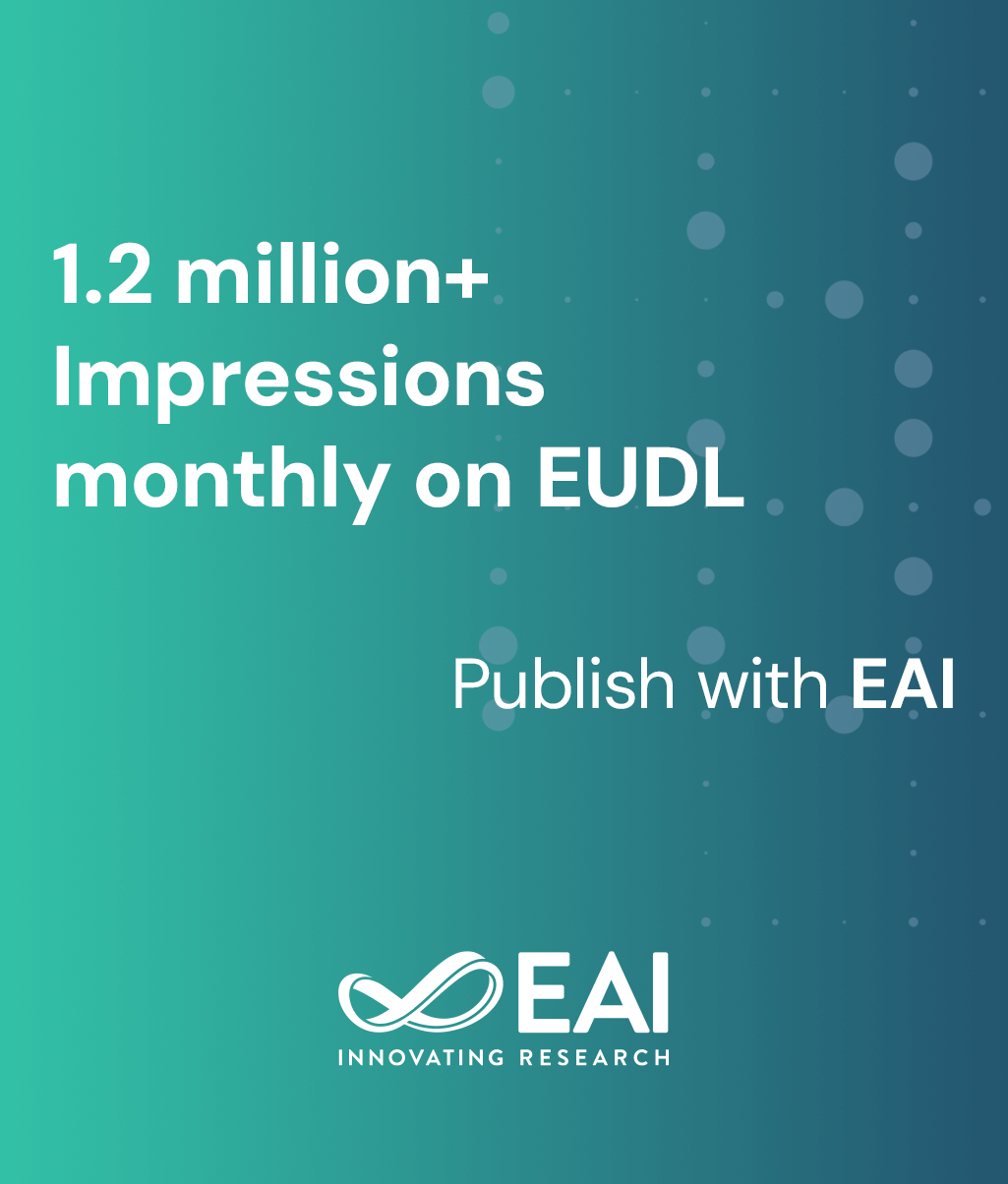
Research Article
Leveraging Self-Attention-Based Deep Learning Networks in Language Processing for Real Crisis Detection on the Web
@INPROCEEDINGS{10.4108/eai.19-5-2023.2334399, author={Haohuan Li}, title={Leveraging Self-Attention-Based Deep Learning Networks in Language Processing for Real Crisis Detection on the Web}, proceedings={Proceedings of the 2nd International Conference on Bigdata Blockchain and Economy Management, ICBBEM 2023, May 19--21, 2023, Hangzhou, China}, publisher={EAI}, proceedings_a={ICBBEM}, year={2023}, month={7}, keywords={lstm self-attention natural language processing classification}, doi={10.4108/eai.19-5-2023.2334399} }
- Haohuan Li
Year: 2023
Leveraging Self-Attention-Based Deep Learning Networks in Language Processing for Real Crisis Detection on the Web
ICBBEM
EAI
DOI: 10.4108/eai.19-5-2023.2334399
Abstract
With the proliferation of social media platforms, there has been a marked surge in the spread of information during crisis events. The identification of authentic crisis-related content on these platforms is essential for effective emergency management and response. In this paper, we introduce a novel approach for predicting the authenticity of crisis-related content using a self-attention-based deep learning network for Natural Language Processing (NLP). In this paper, various self-attention-based layers, Long Short Term Memory(LSTM), Multi Layer Perception(MLP) are explored. The proposed model is trained and evaluated on a dataset of labeled crisis-related posts from various social media platforms. The model demonstrates superior performance in distinguishing between authentic and non-authentic crisis-related content compared to baseline methods. The results suggest that self-attention-based deep learning networks can be effectively utilized for real-time detection of authentic crisis events on social media platforms.