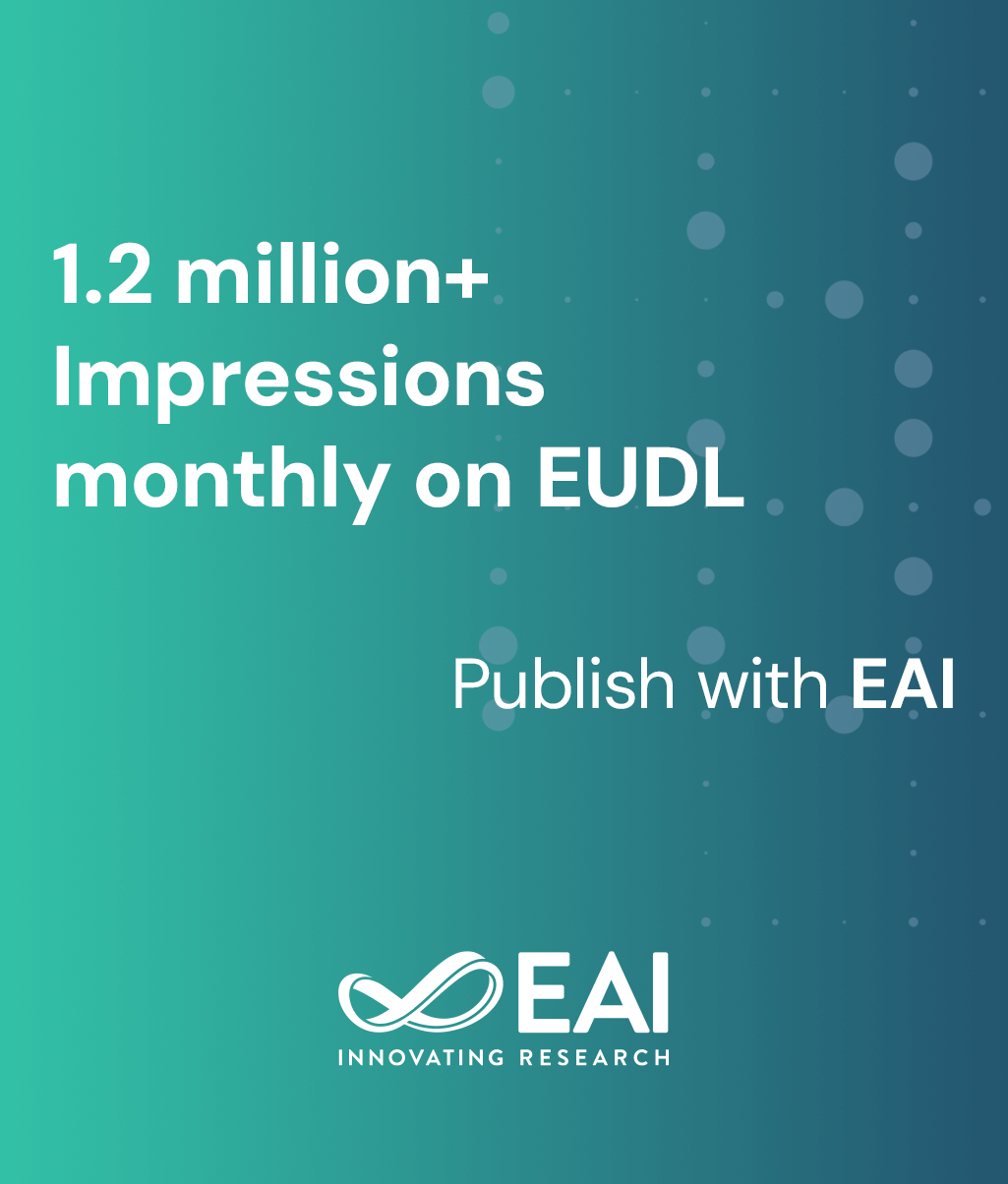
Research Article
Study of Random Forest and XGBoost Quantitative Stock Selection Strategies
@INPROCEEDINGS{10.4108/eai.19-5-2023.2334374, author={Youen Xu and Yang Luo}, title={Study of Random Forest and XGBoost Quantitative Stock Selection Strategies}, proceedings={Proceedings of the 2nd International Conference on Bigdata Blockchain and Economy Management, ICBBEM 2023, May 19--21, 2023, Hangzhou, China}, publisher={EAI}, proceedings_a={ICBBEM}, year={2023}, month={7}, keywords={quantitative stock selection; machine learning; strategy portfolio}, doi={10.4108/eai.19-5-2023.2334374} }
- Youen Xu
Yang Luo
Year: 2023
Study of Random Forest and XGBoost Quantitative Stock Selection Strategies
ICBBEM
EAI
DOI: 10.4108/eai.19-5-2023.2334374
Abstract
The data of all A-share stocks in the Chinese stock market with regular trading in the sample period from 01/01/2015 to 12/31/2021 were analyzed. Factor IC and other methods were used to identify factors that significantly affected the next period return of stocks, and random forest and XGBoost stock selection models were constructed based on the results of factor selection and back-tested on historical data. The two strategies were combined and back-tested using the equal-weighted portfolio and 60/40 portfolio idea methods, respectively, several tests such as annualized return, Sharpe ratio, and maximum retracement rate were compared and analyzed between the combined strategy and the single strategy. The results showed that the two portfolio strategies were more effective than the single strategy in risk control and stable returns, that had some reference value for theoretical research on quantitative stock selection methods.