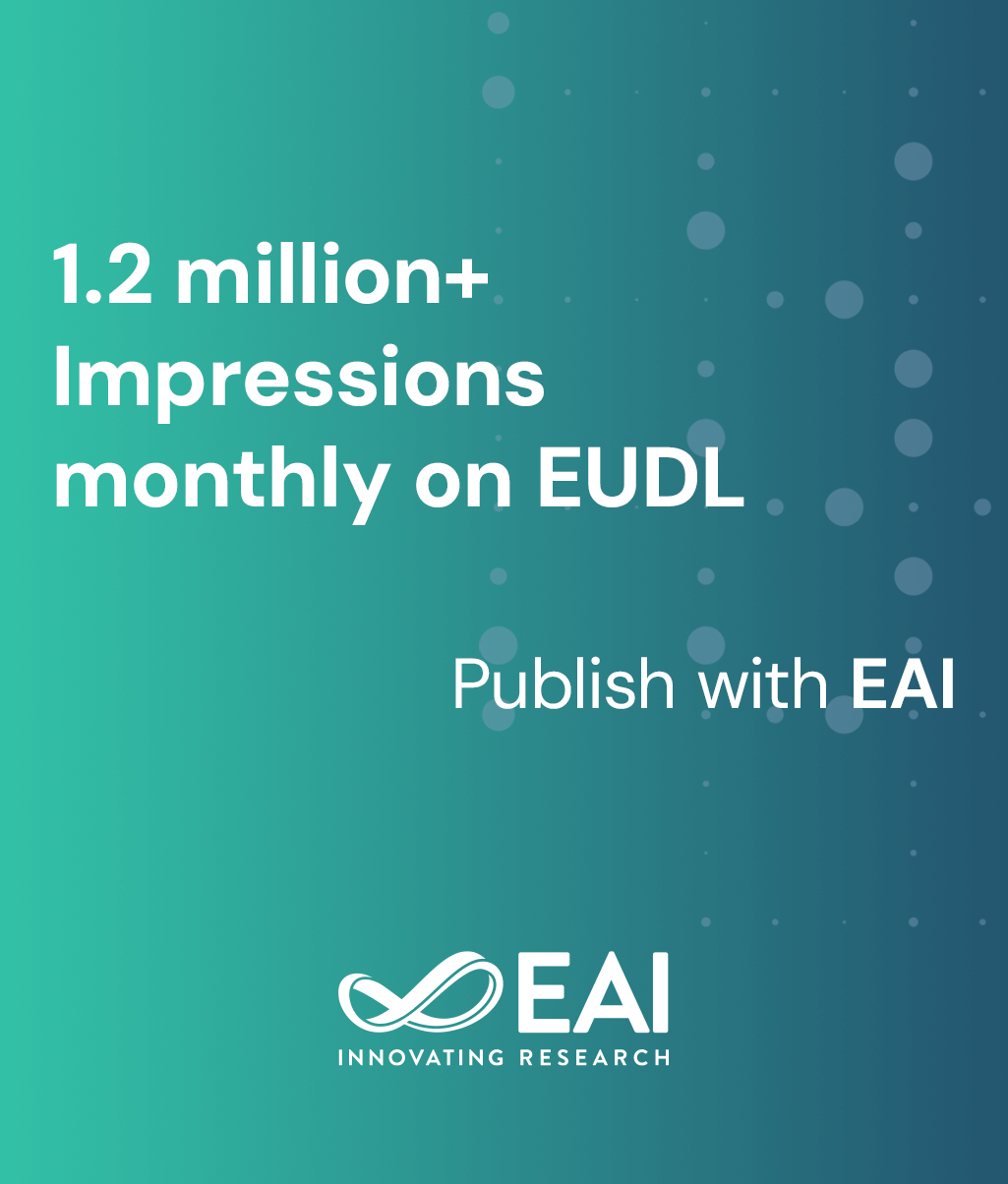
Research Article
A Study of Daily K-Level Quantitative Trading Based on Deep Learning
@INPROCEEDINGS{10.4108/eai.19-5-2023.2334298, author={Hao Cheng}, title={A Study of Daily K-Level Quantitative Trading Based on Deep Learning}, proceedings={Proceedings of the 2nd International Conference on Bigdata Blockchain and Economy Management, ICBBEM 2023, May 19--21, 2023, Hangzhou, China}, publisher={EAI}, proceedings_a={ICBBEM}, year={2023}, month={7}, keywords={quantitative trading lstm daily k-line quantitative backtesting}, doi={10.4108/eai.19-5-2023.2334298} }
- Hao Cheng
Year: 2023
A Study of Daily K-Level Quantitative Trading Based on Deep Learning
ICBBEM
EAI
DOI: 10.4108/eai.19-5-2023.2334298
Abstract
This paper proposes a Daily K-Level Quantitative Trading Strategy (DKTS) based on deep learning, which aims to predict the future stock price trends in the daily K-level and conduct corresponding quantitative trading using historical data and LSTM networks. We use Long Short-Term Memory (LSTM) for feature extraction and predictive modeling, transforming historical data into multidimensional time series data to adapt to the input format of deep learning models. Through the analysis of experimental results on various indexes in the A-share market, this method shows good prediction accuracy and stability. To verify the practical effect of the model, we conducted trading backtests in the actual stock market. The experimental results show that using a deep learning model for trading yields significant returns on multiple indexes and individual stocks, while also demonstrating better risk control and drawdown rates. The research results of this paper indicate that deep learning has broad application prospects in the field of quantitative trading. In the future, we will continue to explore and optimize deep learning models to improve their reliability and stability in practical trading.