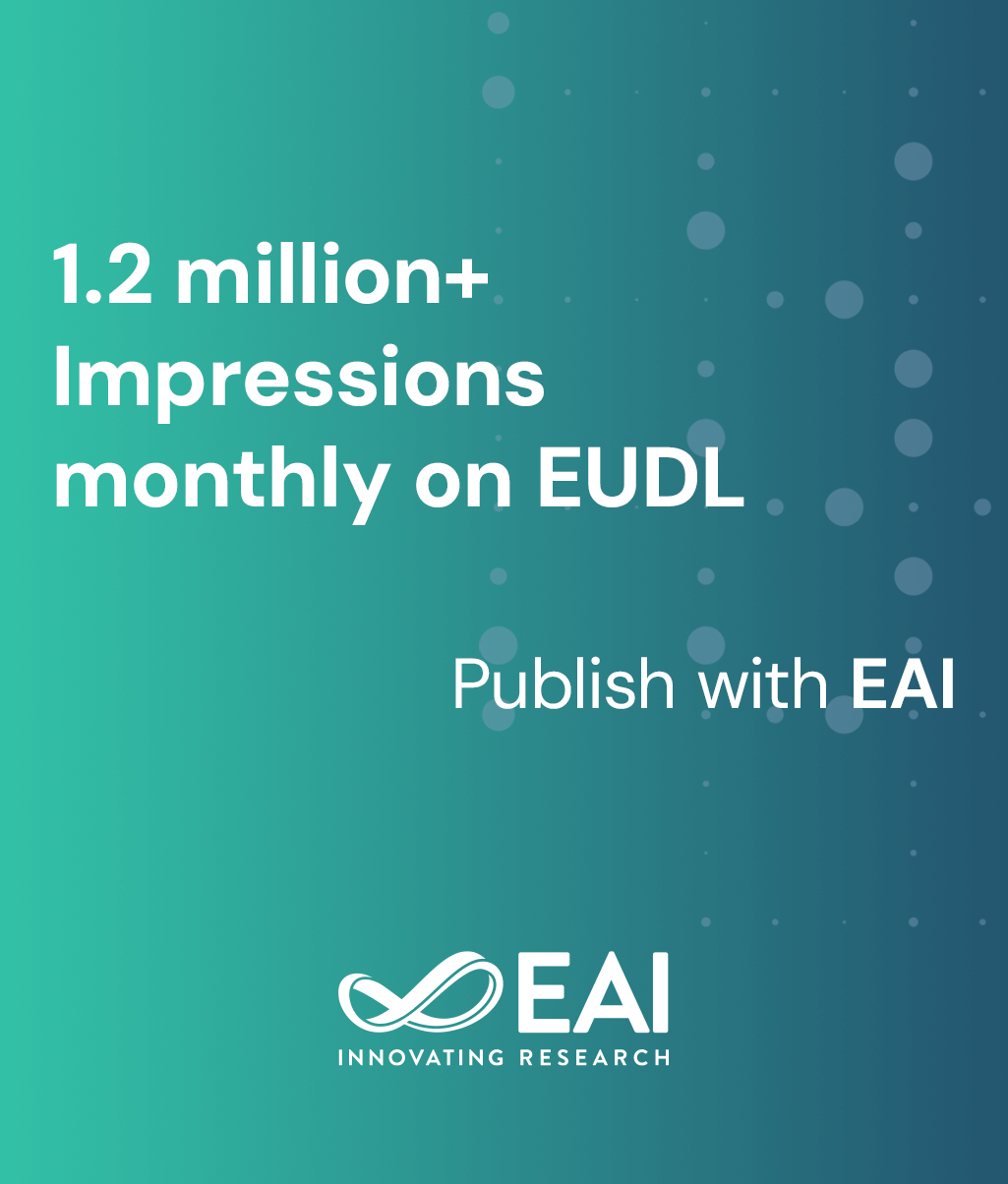
Research Article
BR+ for Addressing Imbalanced Multilabel Data Classification Combined with Resampling Technique
@INPROCEEDINGS{10.4108/eai.19-12-2020.2309179, author={Nilam Novita Sari and Ismaini Zain and Kartika Fithriasari and Amri Muhaimin}, title={BR+ for Addressing Imbalanced Multilabel Data Classification Combined with Resampling Technique}, proceedings={Proceedings of The 6th Asia-Pacific Education And Science Conference, AECon 2020, 19-20 December 2020, Purwokerto, Indonesia}, publisher={EAI}, proceedings_a={AECON}, year={2021}, month={8}, keywords={multilabel imbalanced data br+ smote-nc tomek link random forest}, doi={10.4108/eai.19-12-2020.2309179} }
- Nilam Novita Sari
Ismaini Zain
Kartika Fithriasari
Amri Muhaimin
Year: 2021
BR+ for Addressing Imbalanced Multilabel Data Classification Combined with Resampling Technique
AECON
EAI
DOI: 10.4108/eai.19-12-2020.2309179
Abstract
BR+ is a multilabel method that transforms multilabel into binary single label and assumes label dependency. BR+ can use any different classification method such as random forest. Random forest is an advantageous classification method. But presence of imbalanced classes, random forest will result in poor performance. Hence, handling imbalanced data can be done by applying resampling techniques consisting of SMOTE-NC and T-Link. The dataset used was adolescent risk behavior of drug abuse and premarital sex based on SKAP. The dataset has two labels means there are multilabel problems and the dataset is imbalanced. Thus, the combination of BR+ (Stat) and resampling techniques will be compared in handling multilabel imbalanced data in the classification of adolescent risk behavior using random forest. The results show that the optimum Mtry is 7 and the combination of BR+ (Stat) and T-Link is the best method to handle the multilabel imbalanced data.