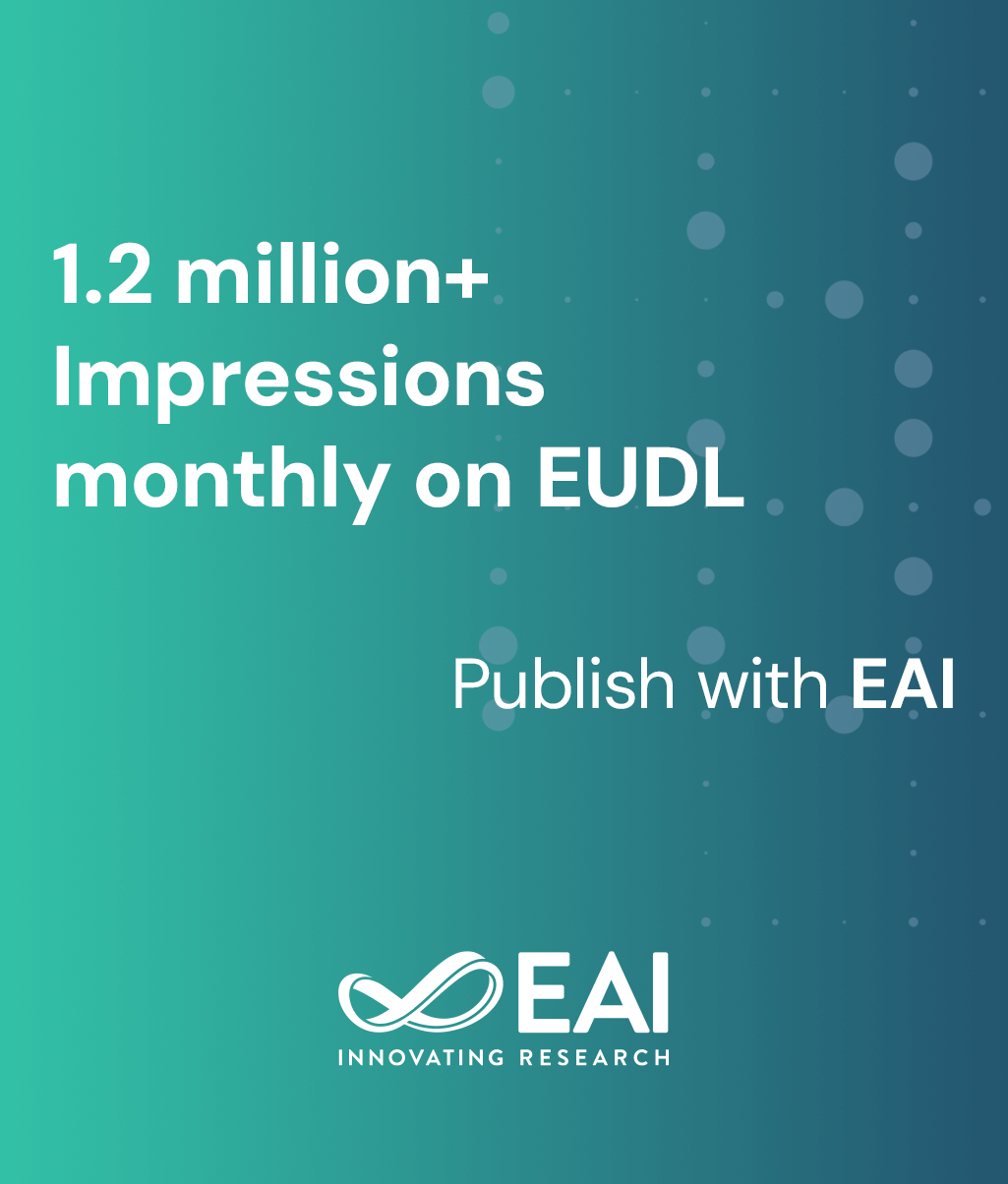
Research Article
An Implementation of ECODB Algorithm to Identify Outliers on the Data of National Exam Scores, Integrity Index, and Accreditation Level of Senior High Schools in Yogyakarta
@INPROCEEDINGS{10.4108/eai.19-10-2018.2282539, author={Angela Mediatrix Melly and Paulina H. Prima Rosa}, title={An Implementation of ECODB Algorithm to Identify Outliers on the Data of National Exam Scores, Integrity Index, and Accreditation Level of Senior High Schools in Yogyakarta}, proceedings={Proceedings of the 1st International Conference on Science and Technology for an Internet of Things, 20 October 2018, Yogyakarta, Indonesia}, publisher={EAI}, proceedings_a={ICSTI}, year={2019}, month={4}, keywords={data mining outlier identification enhanced class outlier distance based algorithm national exam integrity index accreditation level}, doi={10.4108/eai.19-10-2018.2282539} }
- Angela Mediatrix Melly
Paulina H. Prima Rosa
Year: 2019
An Implementation of ECODB Algorithm to Identify Outliers on the Data of National Exam Scores, Integrity Index, and Accreditation Level of Senior High Schools in Yogyakarta
ICSTI
EAI
DOI: 10.4108/eai.19-10-2018.2282539
Abstract
Outlier detection is one field of research in data mining. One of the outlier detection algorithms is the Enhanced Class Outlier Distance Based (ECODB) algorithm. In this paper, the ECODB algorithm is used to identify outliers on national exam results, integrity index and accreditation level of senior high schools in the Yogyakarta province year 2015. An experiment by varying number of outlier and varying number of nearest neighbor were done to identify the value of N and K that result on smallest Class Outlier Factor (COF). The identified N and K were then used to find out schools that are classified as outliers. Further decriptive analysis of the schools were then performed, afterward.
Copyright © 2018–2025 EAI