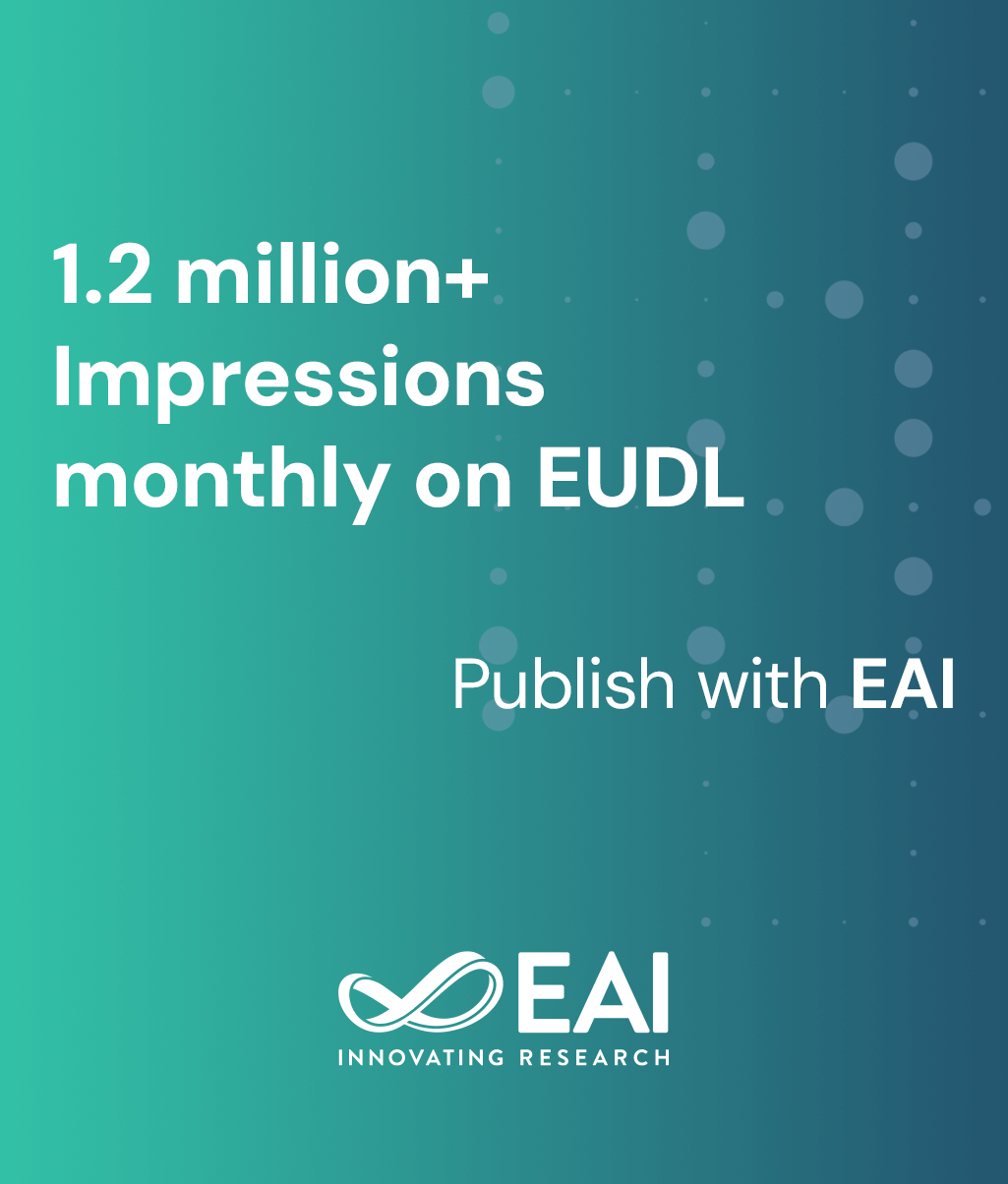
Research Article
A Recommender System Model based on Commodity-Purchase-Cycle Classification
@INPROCEEDINGS{10.4108/eai.18-6-2016.2264232, author={Meina Song and Xue Zhou and Haihong E and Zhonghong Ou}, title={A Recommender System Model based on Commodity-Purchase-Cycle Classification}, proceedings={9th EAI International Conference on Mobile Multimedia Communications}, publisher={ACM}, proceedings_a={MOBIMEDIA}, year={2016}, month={12}, keywords={recommender system itemcf content filtering commodity-purchase-cycle}, doi={10.4108/eai.18-6-2016.2264232} }
- Meina Song
Xue Zhou
Haihong E
Zhonghong Ou
Year: 2016
A Recommender System Model based on Commodity-Purchase-Cycle Classification
MOBIMEDIA
ACM
DOI: 10.4108/eai.18-6-2016.2264232
Abstract
Recommender systems have been widely used in e-commerce platforms, such as Amazon and Taobao. Among the available recommendation algorithms, Item Collaborative Filtering (ItemCF) Algorithm and Content Filtering Algorithm have gained wide adoption because of various strengths. For example, hidden interests can be digged so as to get fresh recommendations, and highly individual recommendations can be made. Despite their strengths and wide adoption, there are still some weaknesses associated with them. One representative weakness is the existence of duplicated, and outdated recommendations due to the lack of purchasing cycles, e.g., weekly or seasonal, of goods. We name such cycles Commodity Purchase Cycle (CPC), and propose a new recommendation algorithm based on CPC in this paper. We leverage CPC attributes to modify the collaborative filtering output rating matrix acquired by the ItemCF Algorithm, and take into consideration both user behaviors and commodity characteristics to make timely recommendations. We utilize a realistic dataset from Taobao to verify the performance of the proposed algorithm. Experimental results demonstrate good performance of CPC algorithm. Specifically, from the perspective of Root Mean Square Error (RMSE), the CPC Algorithm promotes the recommendation accuracy by 15%- 20%, compared with the state-of-the-art ItemCF Algorithm.