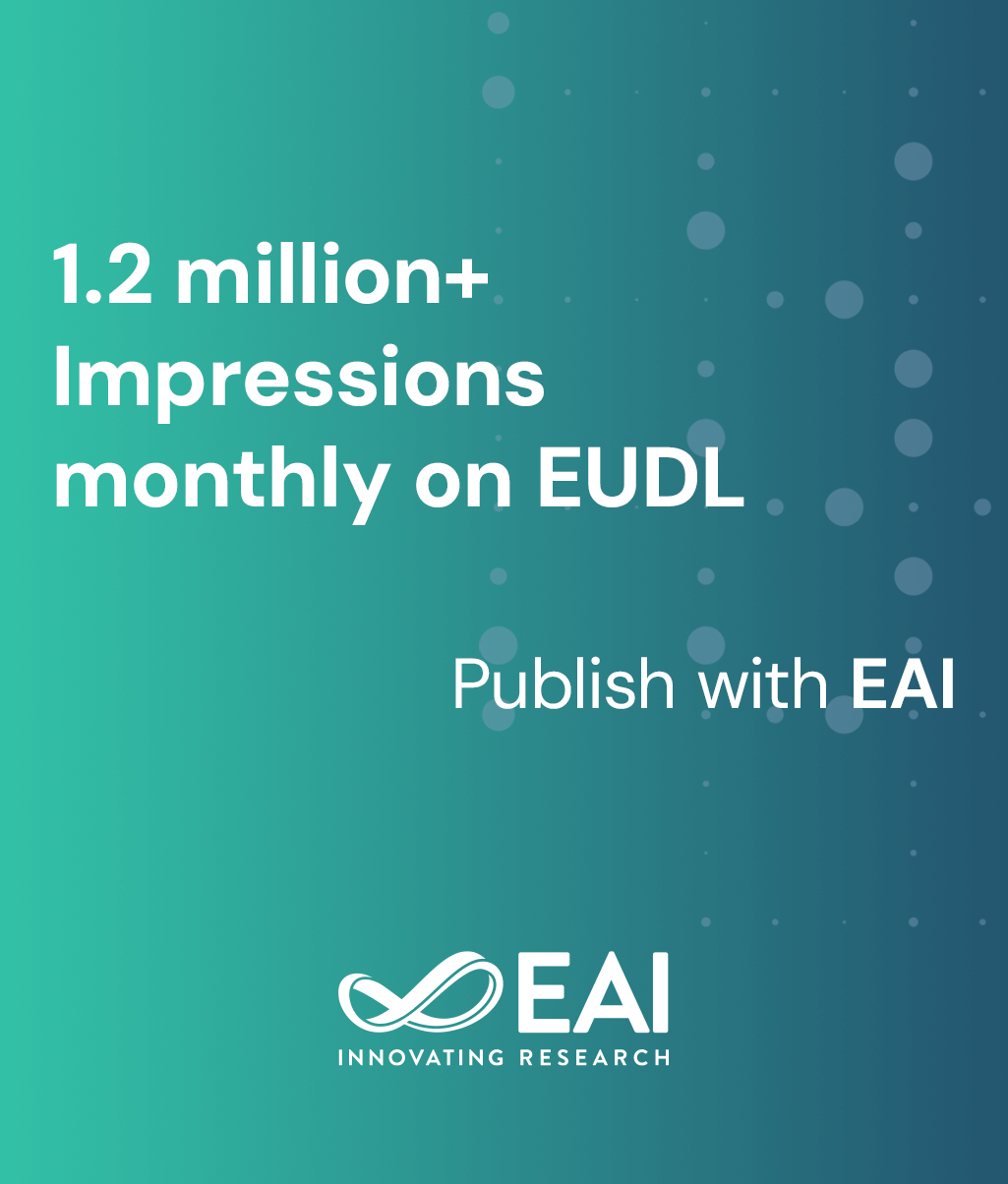
Research Article
AI-driven Generation of News Summaries Leveraging GPT and Pegasus Summarizer for Efficient Information Extraction
@INPROCEEDINGS{10.4108/eai.18-12-2023.2348180, author={Issiaka Faissal Compaore and Rodrigue Kafando and Aminata Sabane and Abdoul Kader Kabore and Tegawende F. Bissyand\^{e}}, title={AI-driven Generation of News Summaries Leveraging GPT and Pegasus Summarizer for Efficient Information Extraction}, proceedings={Proceedings of the 6th Computer Science Research Days, JRI 2023, 18-20 December 2023, Ouagadougou, Burkina Faso}, publisher={EAI}, proceedings_a={JRI}, year={2024}, month={6}, keywords={online press artificial intelligence web scraping transformers abstractive summarization}, doi={10.4108/eai.18-12-2023.2348180} }
- Issiaka Faissal Compaore
Rodrigue Kafando
Aminata Sabane
Abdoul Kader Kabore
Tegawende F. Bissyandé
Year: 2024
AI-driven Generation of News Summaries Leveraging GPT and Pegasus Summarizer for Efficient Information Extraction
JRI
EAI
DOI: 10.4108/eai.18-12-2023.2348180
Abstract
The surge of online information makes it challenging to access relevant news quickly. This necessitates automated methods to effectively extract and summarize information. Our research focuses on designing an online press synthesis tool using advanced AI models. We investigate the feasibility of employing two pre-trained models, GPT-3.5 Turbo 16k and Pegasus Summarizer, to generate high-quality summaries from scraped articles. Our methodology encompasses robust web scraping, model integration, and metric evaluation. Experimental results demonstrate that GPT-3.5 Turbo 16k outperforms in accuracy, achieving a BLEU score of 16.39% and a ROUGE score of 0.66%. The turner007/pegasus-summarizer model also performs well, with a BLEU score of 15.45% and a ROUGE score of 0.45%. We identify areas for improvement, such as enriching the database with expert-authored summaries and adopting a dynamic approach to news adaptation. Additionally, we explore a unified models approach for further refinement.