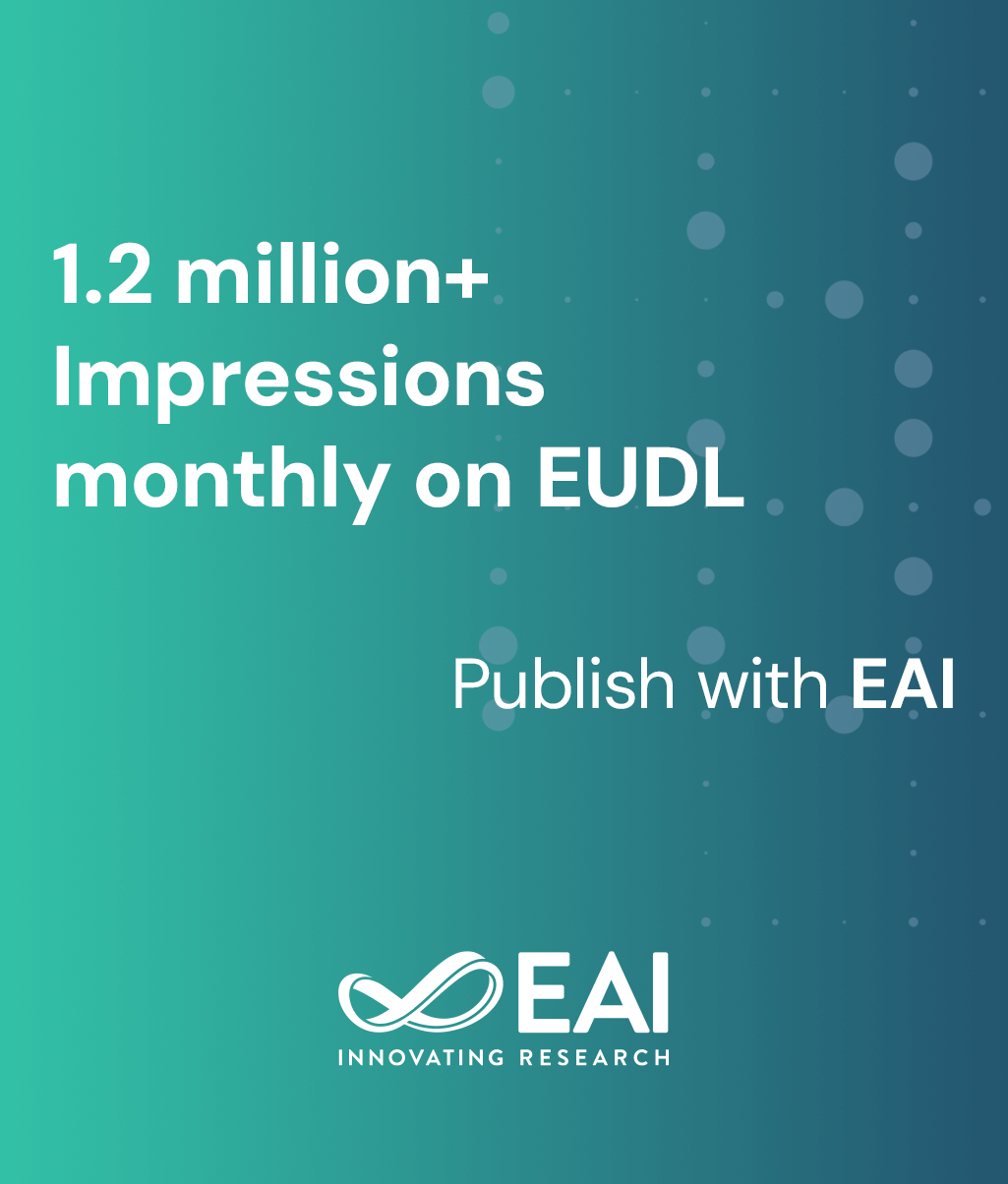
Research Article
Stock Price Prediction Based on Decision Trees, CNN and LSTM
@INPROCEEDINGS{10.4108/eai.18-11-2022.2327160, author={Ruotong Li and Moying Ma and Nan Tang}, title={Stock Price Prediction Based on Decision Trees, CNN and LSTM}, proceedings={Proceedings of the 4th International Conference on Economic Management and Model Engineering, ICEMME 2022, November 18-20, 2022, Nanjing, China}, publisher={EAI}, proceedings_a={ICEMME}, year={2023}, month={2}, keywords={stock price; forecasting; decision trees; cnn; lstm}, doi={10.4108/eai.18-11-2022.2327160} }
- Ruotong Li
Moying Ma
Nan Tang
Year: 2023
Stock Price Prediction Based on Decision Trees, CNN and LSTM
ICEMME
EAI
DOI: 10.4108/eai.18-11-2022.2327160
Abstract
Stock price forecasting plays an important role in quantitative transaction and financial analysis. In this paper, three well-known machine learning approaches, decision tree, LSTM, and CNN, are implemented in order to realize stock price prediction. After introducing each model's background, principle, and method, the historical closing price of China Merchants Bank stock is used as the training set data. After the establishment of the three models, the comparison between the predicted value and the real value on the test set is demonstrated. According to the results, the prediction feasibility is verified to a certain extent. Furthermore, it is concluded that the forecast effect in the short term is better than that in the long term. These results shed light on the effectiveness limitations of machine learning used in quantitative trading.