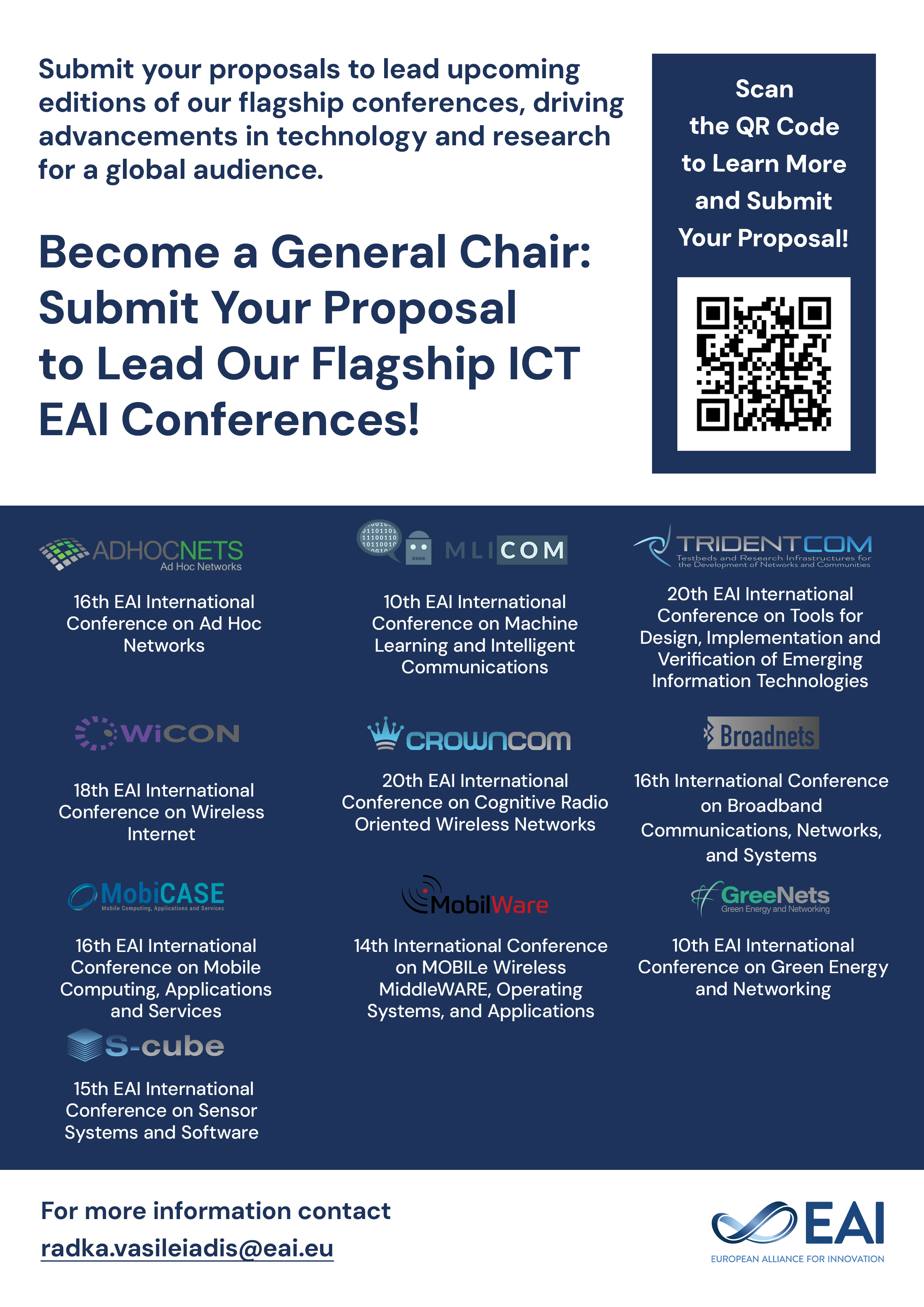
Research Article
Volatility Prediction Based on the Multifactorial Linear Model
@INPROCEEDINGS{10.4108/eai.18-11-2022.2327125, author={Tingyu Dai and Jiawen Song and Shenghui Wang}, title={Volatility Prediction Based on the Multifactorial Linear Model}, proceedings={Proceedings of the 4th International Conference on Economic Management and Model Engineering, ICEMME 2022, November 18-20, 2022, Nanjing, China}, publisher={EAI}, proceedings_a={ICEMME}, year={2023}, month={2}, keywords={volatility prediction; multifactorial model; linear model; ols; ridge; lasso}, doi={10.4108/eai.18-11-2022.2327125} }
- Tingyu Dai
Jiawen Song
Shenghui Wang
Year: 2023
Volatility Prediction Based on the Multifactorial Linear Model
ICEMME
EAI
DOI: 10.4108/eai.18-11-2022.2327125
Abstract
Volatility prediction serves as an important role in financial analysis, which could assist the investors in their financial decisions with the appropriate techniques of prediction on volatility. Meanwhile, volatility models play key roles in the academic literature for testing the fundamental trade-off between risk and return of financial assets and investigating the causes and consequences of the volatility dynamics in the economy. The paper investigates the feasibility of predicting volatility based on the multifactorial linear model. Specifically, 10 highly related factors, selected from systematically scanning, are utilized to predict 10 minutes, 30 minutes, 60 minutes, and 240 minutes of return volatility from the CSI 300 index futures. Three linear regression models including OLS, Lasso, and Ridge are constructed to predict volatility. According to the results, it is verified the prediction method is valid, and the overall goodness of fit is approximately 20%. In addition, each frequency has reported similar effects as expected. Based on the analysis, it is noted that the lower the frequency of the volatility, the better the result will be. These results shed light on volatility forecasting based on multifactorial models.