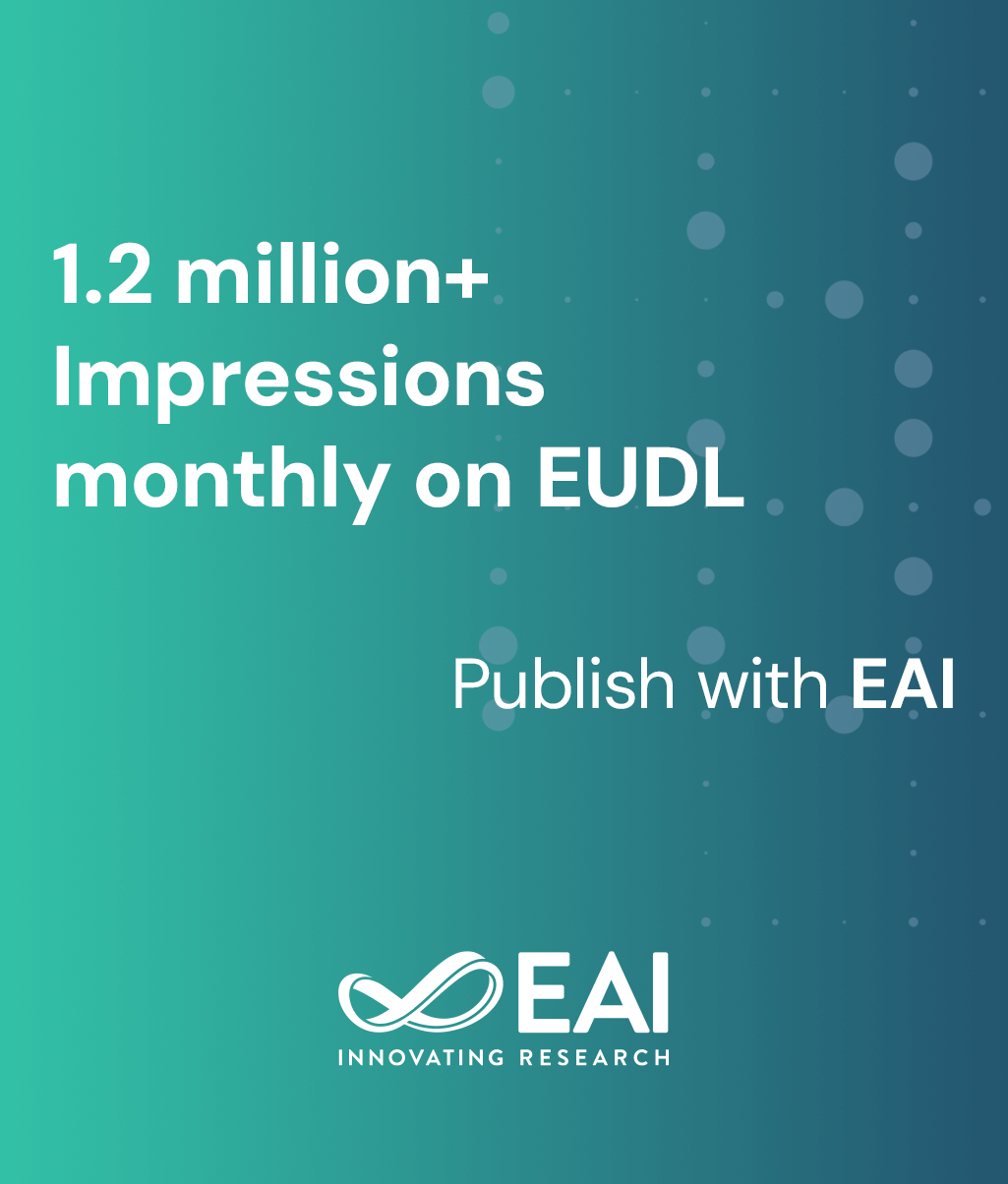
Research Article
Reinforcement Learning in Portfolio Management with Sharpe Ratio Rewarding Based Framework
@INPROCEEDINGS{10.4108/eai.18-11-2022.2327121, author={Zhenqiang Liu}, title={Reinforcement Learning in Portfolio Management with Sharpe Ratio Rewarding Based Framework}, proceedings={Proceedings of the 4th International Conference on Economic Management and Model Engineering, ICEMME 2022, November 18-20, 2022, Nanjing, China}, publisher={EAI}, proceedings_a={ICEMME}, year={2023}, month={2}, keywords={portfolio management; deep q-network (dqn); model-free reinforcement learning; sharpe ratio; mean variance optimization (mvo)}, doi={10.4108/eai.18-11-2022.2327121} }
- Zhenqiang Liu
Year: 2023
Reinforcement Learning in Portfolio Management with Sharpe Ratio Rewarding Based Framework
ICEMME
EAI
DOI: 10.4108/eai.18-11-2022.2327121
Abstract
Portfolio management is a financial operation which aims at maximizing the return or optimizing the Sharpe Ratio. One widely used portfolio management strategy, Mean-Variance Optimization, also known as Modern Portfolio Theory, mainly profits by focusing on finding out the expected return and variance of stocks based on historical data to maximize Sharpe Ratio. Yet, it is not easy and accurate to simply predict future return and variance based on a formula. So, in this paper, two Models-free framework, Sharpe Ratio reward based Deep Q-Network (DQN-S) and Return reward (DQN-R) are proposed to overcome the limitations above. Deep Q-learning was employed to train a neural network to manage a stock portfolio of 10 stocks. Stock price was defined as environment of NN, weight of portfolio was defined as action of neural network agent, and reward was indicated to train the model. Traditional portfolio allocation strategy Mean Variance Optimization (MVO) and Naïve Portfolio Allocation (NPA) were also introduced as benchmark to evaluate the performance of reinforcement learning. Moreover, the extensiveness of DQN-S was discussed. The result shows that the MVO is dominating the NPA with a 5% higher annual return and 0.5 higher of Sharpe ratio, although the MDD is slightly higher, indicating the superiority of Sharpe Ratio oriented strategy.