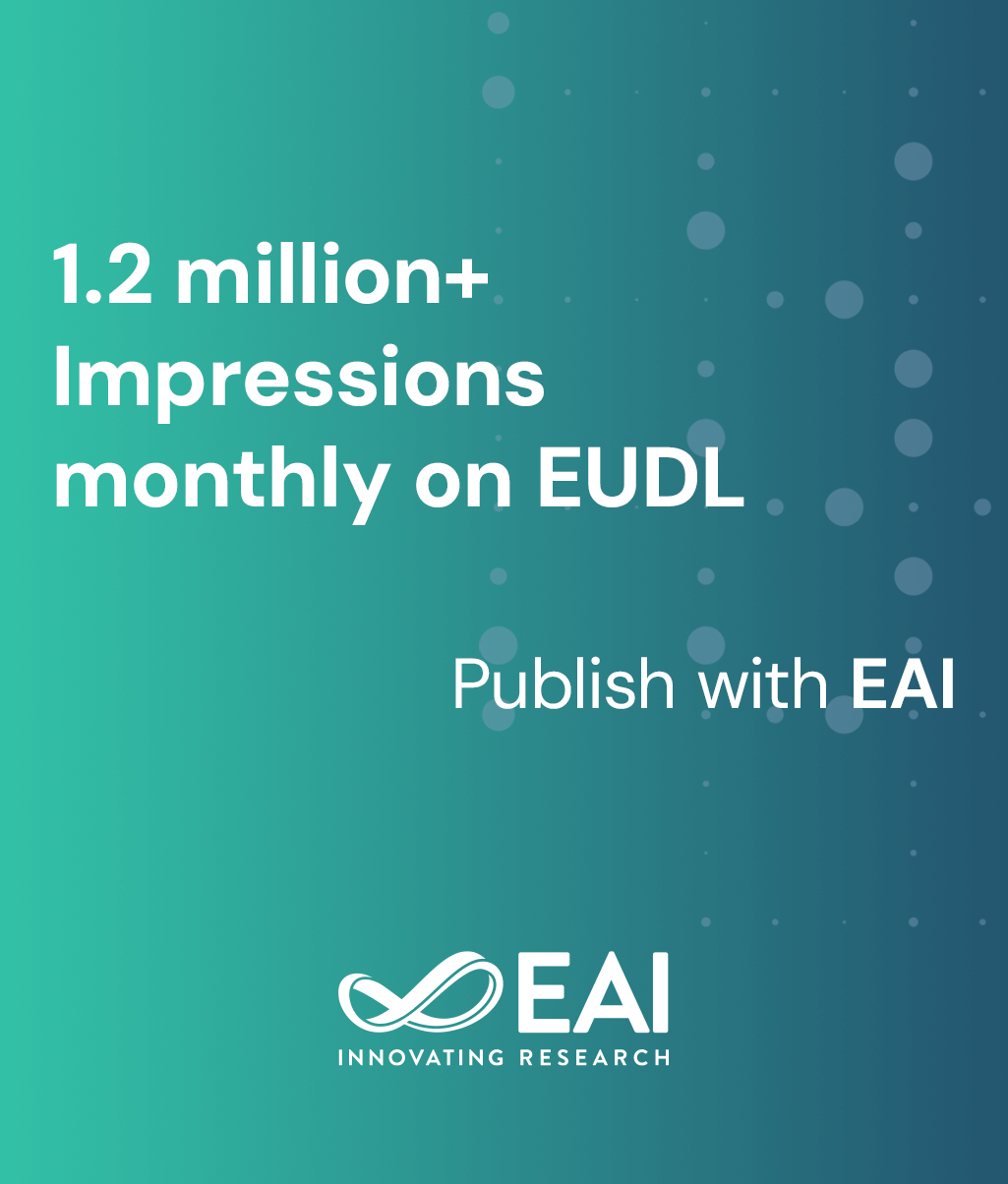
Research Article
The Progress of Stock Price Forecasting Model Based on AI Techniques: ARIMA, Neural Networks and LSTM
@INPROCEEDINGS{10.4108/eai.18-11-2022.2327116, author={Tianhao Gao and Yuwen Huo and Mengmeng Yu}, title={The Progress of Stock Price Forecasting Model Based on AI Techniques: ARIMA, Neural Networks and LSTM }, proceedings={Proceedings of the 4th International Conference on Economic Management and Model Engineering, ICEMME 2022, November 18-20, 2022, Nanjing, China}, publisher={EAI}, proceedings_a={ICEMME}, year={2023}, month={2}, keywords={price forecasting; arima; machine learning; neural networks; lstm}, doi={10.4108/eai.18-11-2022.2327116} }
- Tianhao Gao
Yuwen Huo
Mengmeng Yu
Year: 2023
The Progress of Stock Price Forecasting Model Based on AI Techniques: ARIMA, Neural Networks and LSTM
ICEMME
EAI
DOI: 10.4108/eai.18-11-2022.2327116
Abstract
In contemporary society, under great demand for effective investing, stock price forecasting is always a hot topic in financial research. On this basis, this study investigates the price forecasting models based on three common used measures and approaches, i.e., ARIMA, Neural networks and LSTM models regarding information retrieval and literature review methods. Specifically, for Artificial neural networks, we present a traditional three-layer model consisting of input, hidden and output layers with six variables. As for ARIMA, we mainly determine the main application scope of ARIMA through the brief comparison between ARIMA and other models in stock forecasting and the advantages and disadvantages of ARIMA. With regard to LSTM, we discuss the study of its various doors and functions and the analysis of its advantages and disadvantages. According to our analysis, among the three selected scenarios, LSTM shows pretty well performances among various approaches. Overall, these results shed light on future stock predicting model improvement.