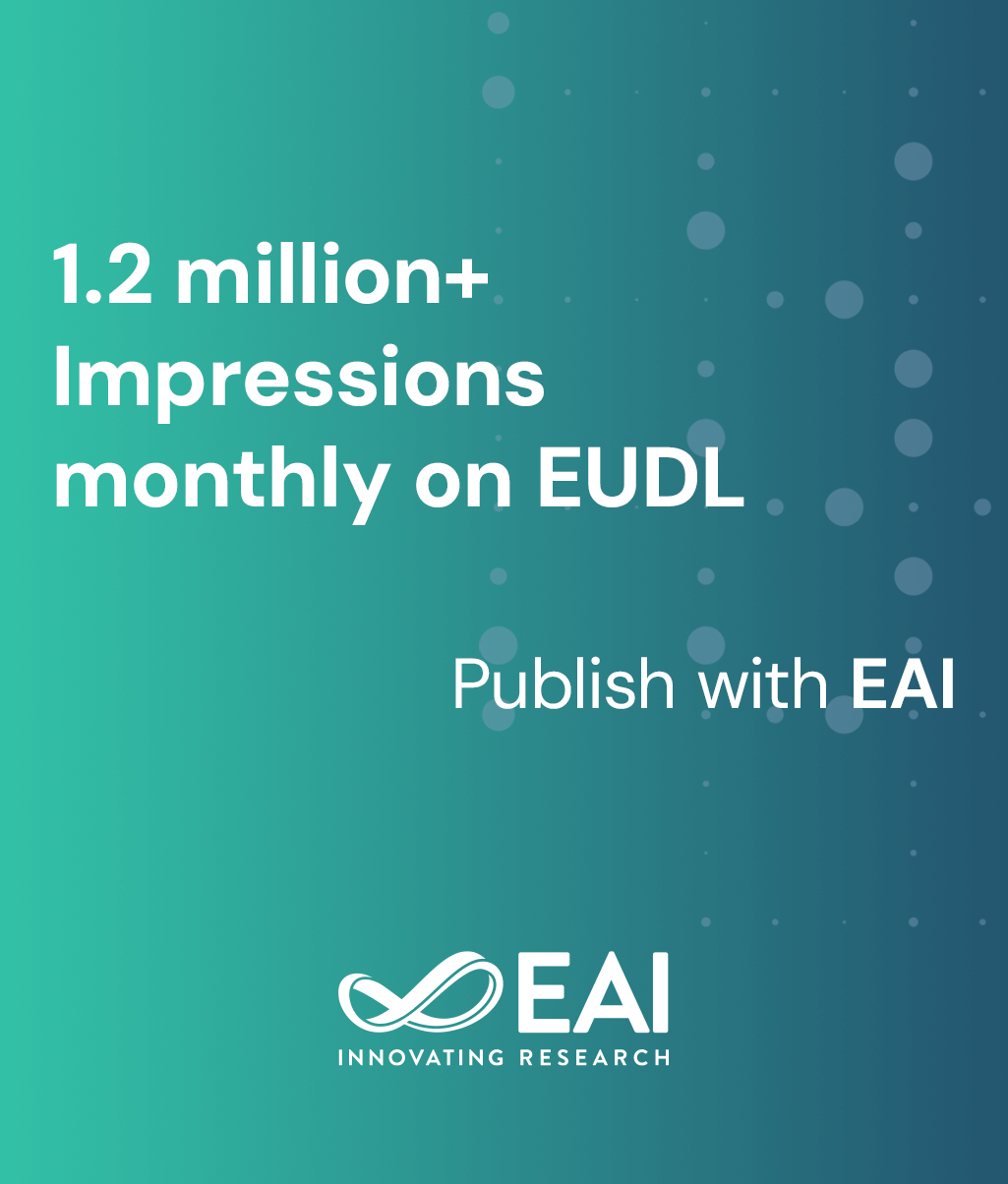
Research Article
The Application of Neural Network in Stock and Futures Forecasting
@INPROCEEDINGS{10.4108/eai.18-11-2022.2326945, author={Xiangru Li and Zeyuan Wang}, title={The Application of Neural Network in Stock and Futures Forecasting}, proceedings={Proceedings of the 4th International Conference on Economic Management and Model Engineering, ICEMME 2022, November 18-20, 2022, Nanjing, China}, publisher={EAI}, proceedings_a={ICEMME}, year={2023}, month={2}, keywords={stock market; price forecast; neural network; rbf; bp}, doi={10.4108/eai.18-11-2022.2326945} }
- Xiangru Li
Zeyuan Wang
Year: 2023
The Application of Neural Network in Stock and Futures Forecasting
ICEMME
EAI
DOI: 10.4108/eai.18-11-2022.2326945
Abstract
The forecasting of stock and futures markets has always been an attractive technical issue in the financial field. This paper introduces the basic principles of RBF and BP neural networks and expounds on their calculation methods in stock and futures market prediction, respectively. RBF neural network uses the three-layer network to build the prediction model of the stock market. Based on preprocessing the sample data, determining the RBF neural network center and training the output layer weight of the RBF network, it can accurately predict the stock price without changing the model and various parameters. BP neural network has been widely used in function approximation, pattern recognition, classification and data compression. It extracts 10 kinds of possibly relevant data from futures transaction data, calculates the correlation coefficient, extracts four key factors, and constructs a prediction model. It improves the calculation accuracy compared with using 10 items of data directly. Meanwhile, the paper also points out two neural networks' improvements in current use and puts forward feasible methods. These results shed light on the application of neural networks in the stock market and provided guidance for the future development and innovation of neural networks.