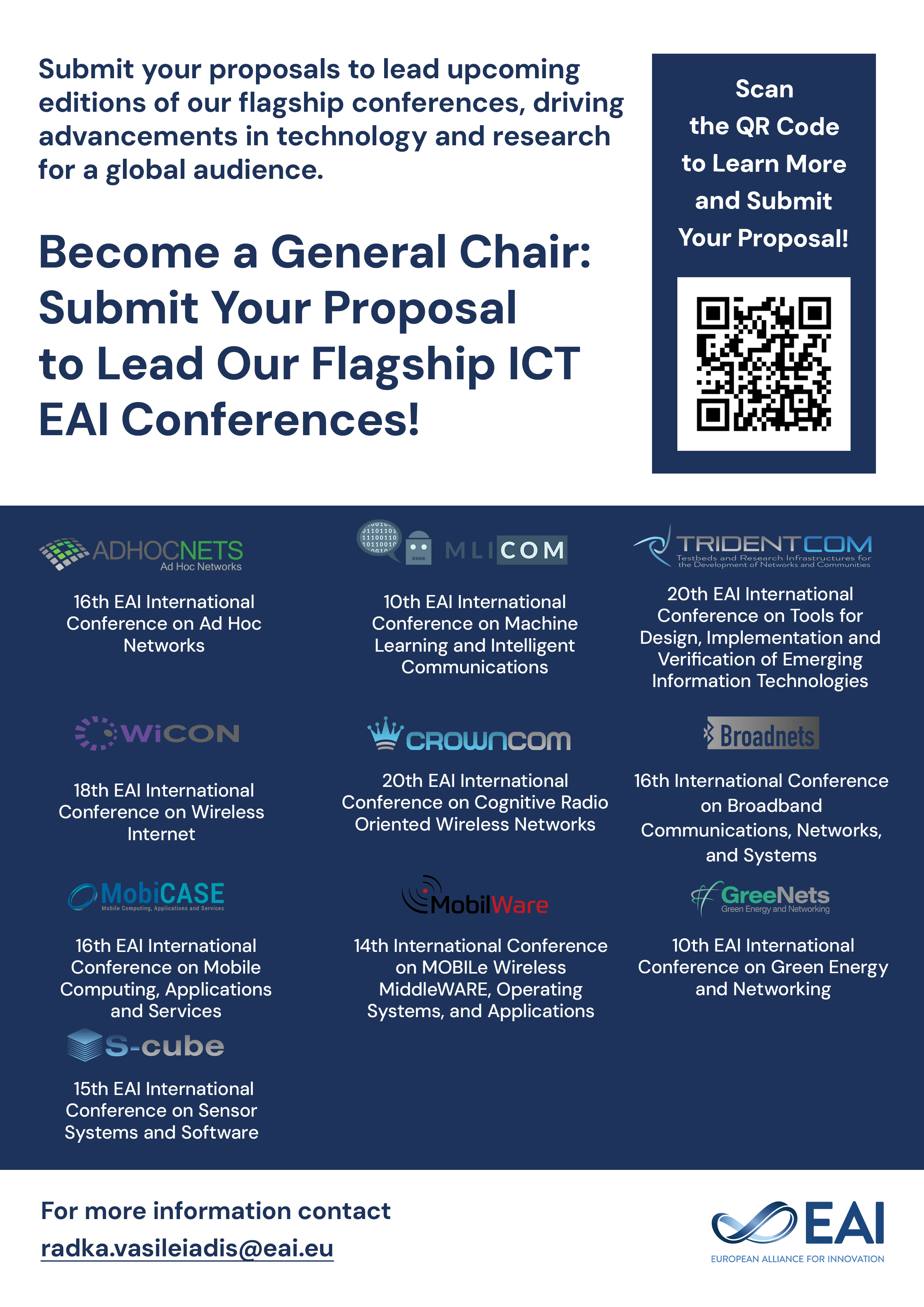
Research Article
Generalized Deep Neural Network and its Application in Financial Time Series Forecasting
@INPROCEEDINGS{10.4108/eai.17-6-2022.2322846, author={Ningrui Du}, title={Generalized Deep Neural Network and its Application in Financial Time Series Forecasting}, proceedings={Proceedings of the International Conference on Information Economy, Data Modeling and Cloud Computing, ICIDC 2022, 17-19 June 2022, Qingdao, China}, publisher={EAI}, proceedings_a={ICIDC}, year={2022}, month={10}, keywords={generalized deep neural networks financial time series forecasting research}, doi={10.4108/eai.17-6-2022.2322846} }
- Ningrui Du
Year: 2022
Generalized Deep Neural Network and its Application in Financial Time Series Forecasting
ICIDC
EAI
DOI: 10.4108/eai.17-6-2022.2322846
Abstract
Generalized representation is a very important concept in deep learning, which can treat multiple data as random sequences. The different types and parameters are somehow intrinsically related to each other and independent of each other. The generalized representation model has a very important role in financial time series, and the generalized analysis method is a kind of deep learning based on data mining, which can solve a large number of complex problems encountered in financial time series forecasting. Since traditional neural networks have the advantages of high complexity, real-time and good stability, their applications have a wide development prospect in many fields, but the current research hotspots mainly focus on how to improve the quality and accuracy of the model, and the research on generalized deep neural networks is still relatively small. Therefore, this paper investigates the modeling and prediction performance of generalized deep neural network models based on the generalized analysis method.