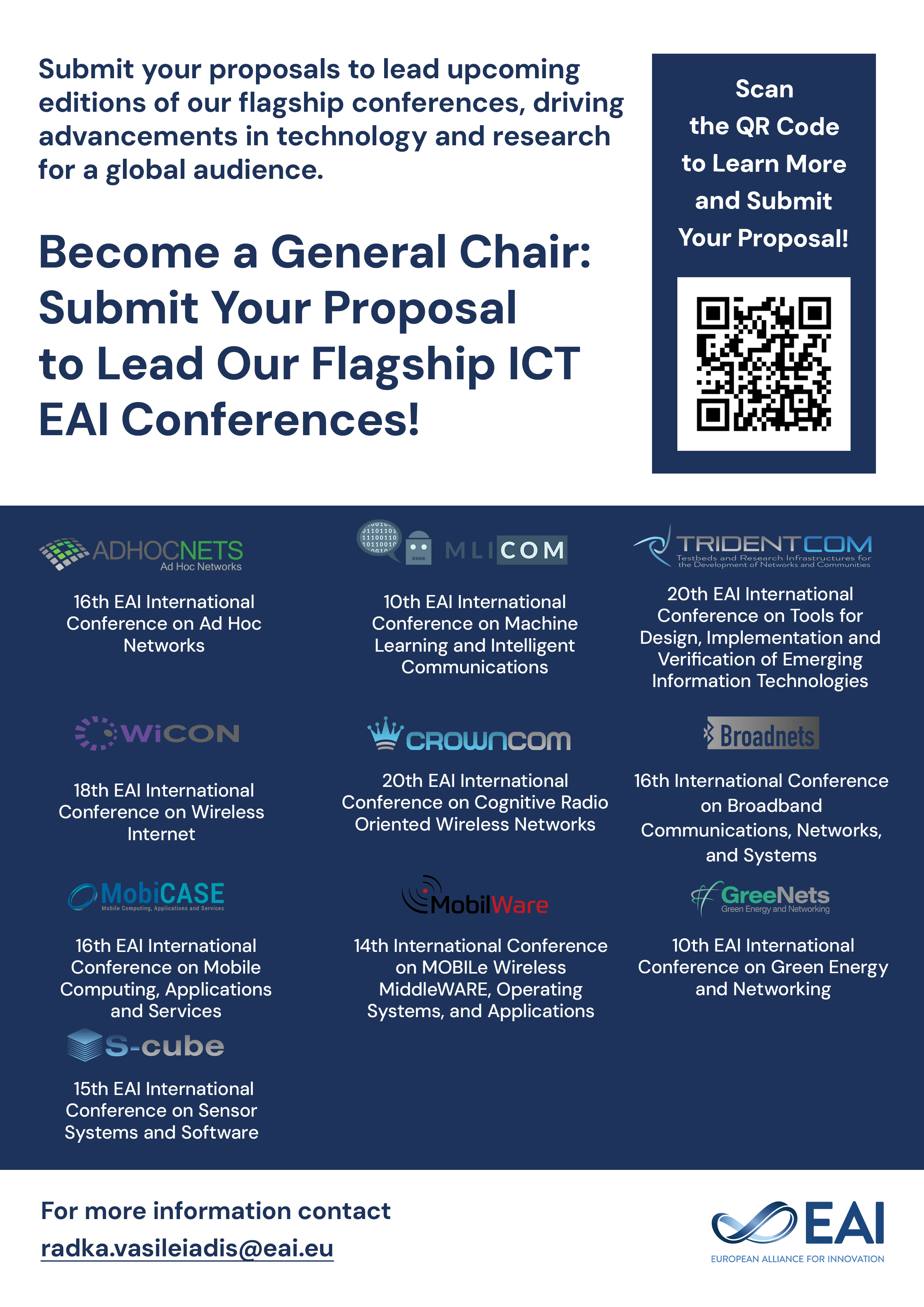
Research Article
An Adaptive Collaborative Filtering Based Approach for Point-of-Interest Recommendations
@INPROCEEDINGS{10.4108/eai.17-6-2022.2322634, author={Yueyu Wang and Leyan Chen and Jiani Chen}, title={An Adaptive Collaborative Filtering Based Approach for Point-of-Interest Recommendations}, proceedings={Proceedings of the International Conference on Information Economy, Data Modeling and Cloud Computing, ICIDC 2022, 17-19 June 2022, Qingdao, China}, publisher={EAI}, proceedings_a={ICIDC}, year={2022}, month={10}, keywords={activity region point-of- interest (poi) recommendation system collaborative filtering (cf)}, doi={10.4108/eai.17-6-2022.2322634} }
- Yueyu Wang
Leyan Chen
Jiani Chen
Year: 2022
An Adaptive Collaborative Filtering Based Approach for Point-of-Interest Recommendations
ICIDC
EAI
DOI: 10.4108/eai.17-6-2022.2322634
Abstract
LBSNs (Location-based Social Networks) provide abundant information for users to browse and explore the places where they are interested in, named POI (Point-of-Interest). However, such large amount of check-in records in LBSNs cause information overload problem and increase difficulty for users to find the really desire POIs. POI recommendation systems can be employed to solve this problem. Most traditional POI recommendation methods are CF (Collaborative Filtering) based and achieve recommendations for a particular user according to check-in records of his similar users. In this paper, we propose an adaptive CF based algorithm to achieve POI recommendations for users, considering their personalized activity regions. Compare with existing algorithm, our algorithm does not construct user-POI matrix by using the entire historical records. Instead, we first explore users’ activity regions and construct more personalized user-POI matrix for each particular user according to corresponding activity regions. Besides, we propose a method to dynamically determine the number of similar users for a certain user, instead of using a fix number for all users, leading to more personalized recommendations. We have implemented our POI recommendation system and compared with state-of-the-art methods by using Foursquare dataset. The experimental results show that our POI recommendation system achieves better performance than all these compared approaches.