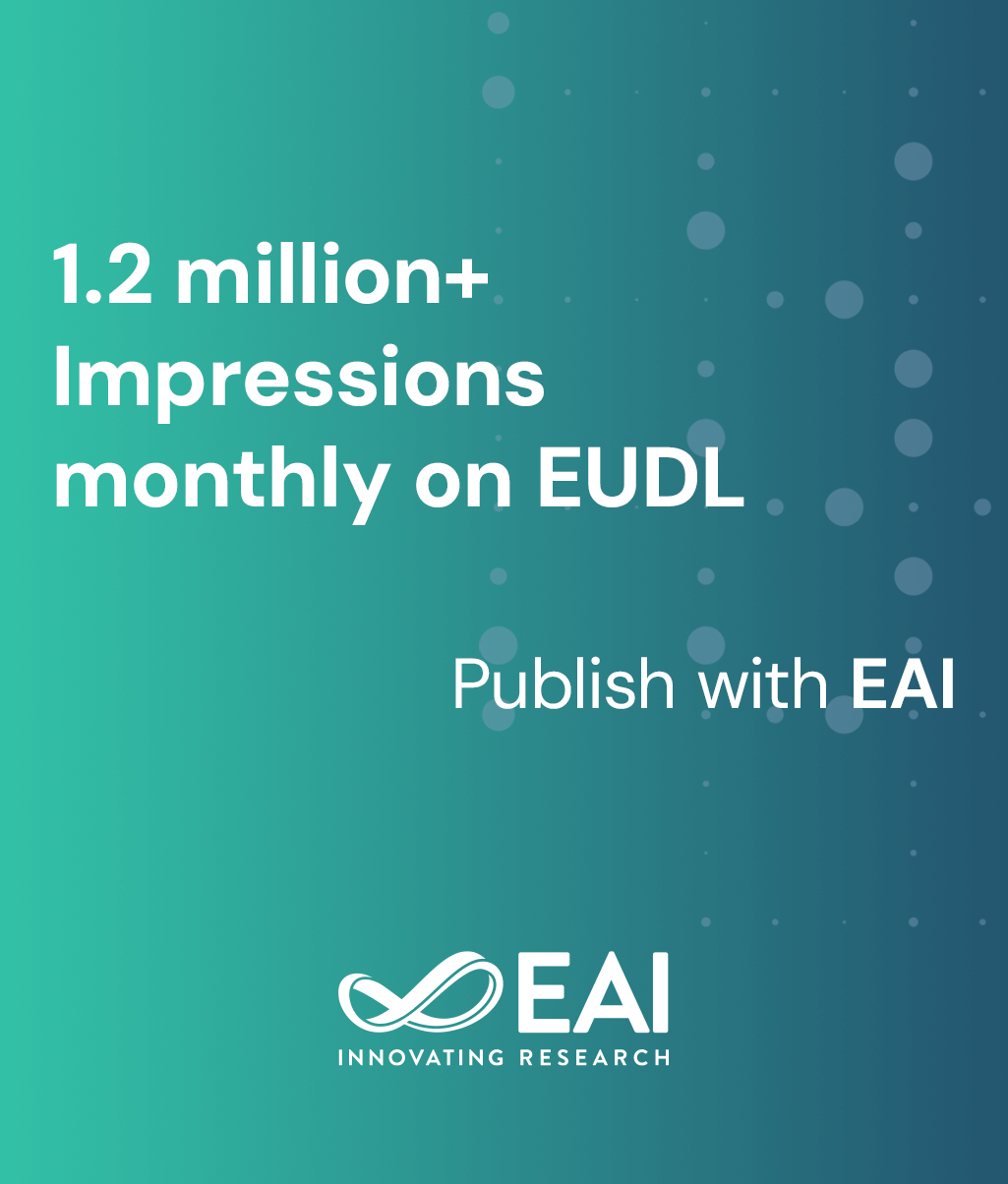
Research Article
Credit Rating Analysis with Decision Tree Method in Housing Loans through the United States
@INPROCEEDINGS{10.4108/eai.17-6-2022.2322597, author={Yuechen Mu}, title={Credit Rating Analysis with Decision Tree Method in Housing Loans through the United States}, proceedings={Proceedings of the International Conference on Information Economy, Data Modeling and Cloud Computing, ICIDC 2022, 17-19 June 2022, Qingdao, China}, publisher={EAI}, proceedings_a={ICIDC}, year={2022}, month={10}, keywords={decision tree; neural network; support vector machines}, doi={10.4108/eai.17-6-2022.2322597} }
- Yuechen Mu
Year: 2022
Credit Rating Analysis with Decision Tree Method in Housing Loans through the United States
ICIDC
EAI
DOI: 10.4108/eai.17-6-2022.2322597
Abstract
With the development of society, the problem of buying houses has become one of the main debt pressures of contemporary people, and housing loans can effectively alleviate this situation. This article tries to use data statistics to discover the preferences of loan groups and find the best classification method for predicting the housing loan people. In order to analyze more accurately, all personal data are sampled from the United States, and their influence on housing loans is judged by comparing individuals' gender, marriage, graduation, employment relationship, and income. This article will use three statistical algorithms for data testing and training, include Decision Tree, Artificial Neural Network, and Support Vector Machines. Through sampling training of seventy percent of the total data, a statistical model is built based on the above three methods. Then the remaining data is used for testing respectively to verify or modify the training model to improve the accuracy of prediction. The final conclusion is that the sampled data cannot be one hundred percent through the evaluation parameters to get the result of the housing loan, the main reason is that the sources of parameters are limited. We can only assess the basic conditions of people, but there are no more sources of information to fully label individuals.