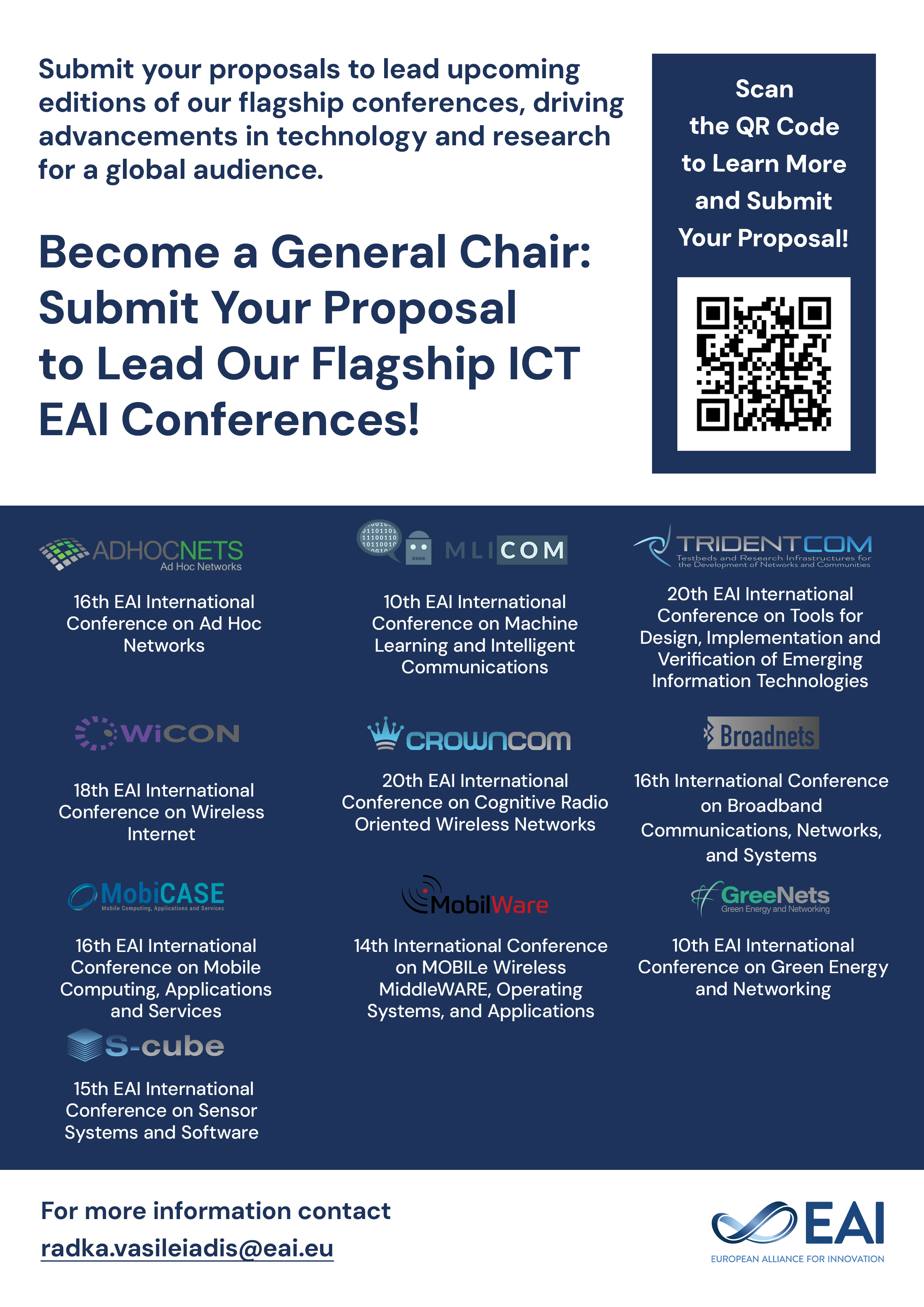
Research Article
Employing U-NET and RBCNN to Build an Automatic Lung Cancer Segmentation and Classification System
@INPROCEEDINGS{10.4108/eai.17-11-2023.2342832, author={P Dhivya and P Yamini}, title={Employing U-NET and RBCNN to Build an Automatic Lung Cancer Segmentation and Classification System}, proceedings={Proceedings of the First International Conference on Science, Engineering and Technology Practices for Sustainable Development, ICSETPSD 2023, 17th-18th November 2023, Coimbatore, Tamilnadu, India}, publisher={EAI}, proceedings_a={ICSETPSD}, year={2024}, month={1}, keywords={computer assisted system lung cancer detection lung nodule segmentation feature extraction rbcnn classification}, doi={10.4108/eai.17-11-2023.2342832} }
- P Dhivya
P Yamini
Year: 2024
Employing U-NET and RBCNN to Build an Automatic Lung Cancer Segmentation and Classification System
ICSETPSD
EAI
DOI: 10.4108/eai.17-11-2023.2342832
Abstract
The most common cancer-related cause of death globally is lung cancer. The key to effective lung cancer treatment and higher survival rates is early diagnosis. Converting a radiologist's diagnosing procedure to computer assisted results in more accurate results and an earlier diagnosis. The difficulty is that building a effective model for segmentation and classification. In this paper, we suggest a system for detecting lung cancer that makes use of a number of methods for precise and effective diagnosis. To enhance picture quality, our method pre-processes CT scan images using a Gaussian filter and contrast stretching. For the purpose of determining the borders of lung nodules with high precision, the U-Net architecture with the Adam optimizer is used. Then, a Gaussian mixture model (GMM) with EM optimisation and pixel padding is used to extract features. The rotational-based CNN (RBCNN) classifier successfully categorises the nodules as benign and malignant using these form variables as inputs