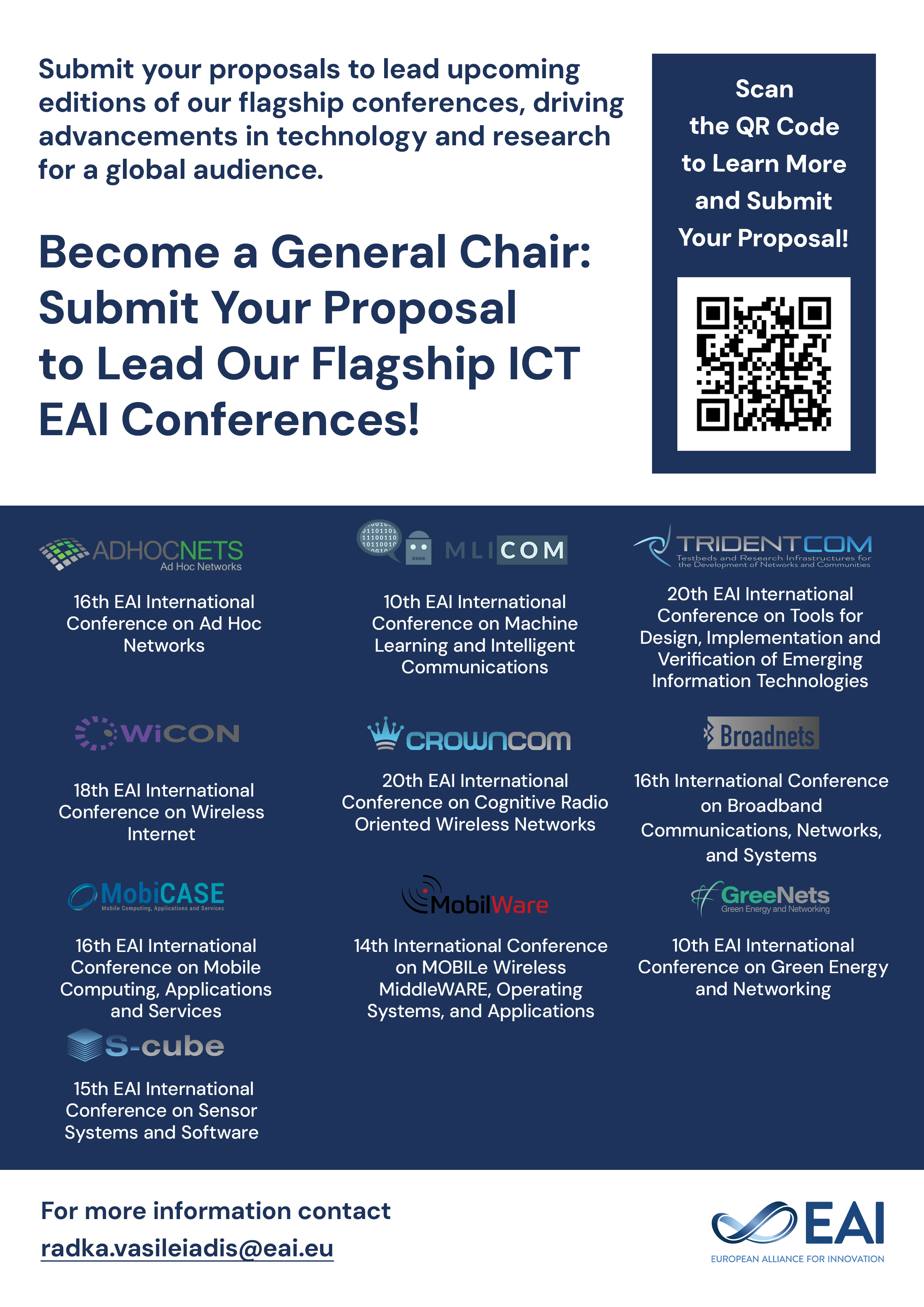
Research Article
House Price Prediction Based on Machine Learning --Taking Beijing House Price Data as an Example
@INPROCEEDINGS{10.4108/eai.17-11-2023.2342672, author={Yuhong Xu}, title={House Price Prediction Based on Machine Learning --Taking Beijing House Price Data as an Example}, proceedings={Proceedings of the 5th International Conference on Economic Management and Model Engineering, ICEMME 2023, November 17--19, 2023, Beijing, China}, publisher={EAI}, proceedings_a={ICEMME}, year={2024}, month={2}, keywords={machine learning feature engineering house prices prediction}, doi={10.4108/eai.17-11-2023.2342672} }
- Yuhong Xu
Year: 2024
House Price Prediction Based on Machine Learning --Taking Beijing House Price Data as an Example
ICEMME
EAI
DOI: 10.4108/eai.17-11-2023.2342672
Abstract
House price prediction has significant practical value in the real estate market, as it provides critical decision support for specific needs of buyers, sellers, and investors. However, accurate forecasting remains challenging due to the complex and multi-factorial nature of housing prices. This study investigates the application of machine learning algorithms for house price forecasting to enhance predictive accuracy. To achieve this aim, we examine several classical machine learning models including decision trees, linear regression, k-nearest neighbors, random forests, AdaBoost, Bagging, gradient boosting, and Extra Tree. Using housing price data from Beijing containing attributes such as transaction time, age of the house, and the average community price, we employ feature engineering to process the data.