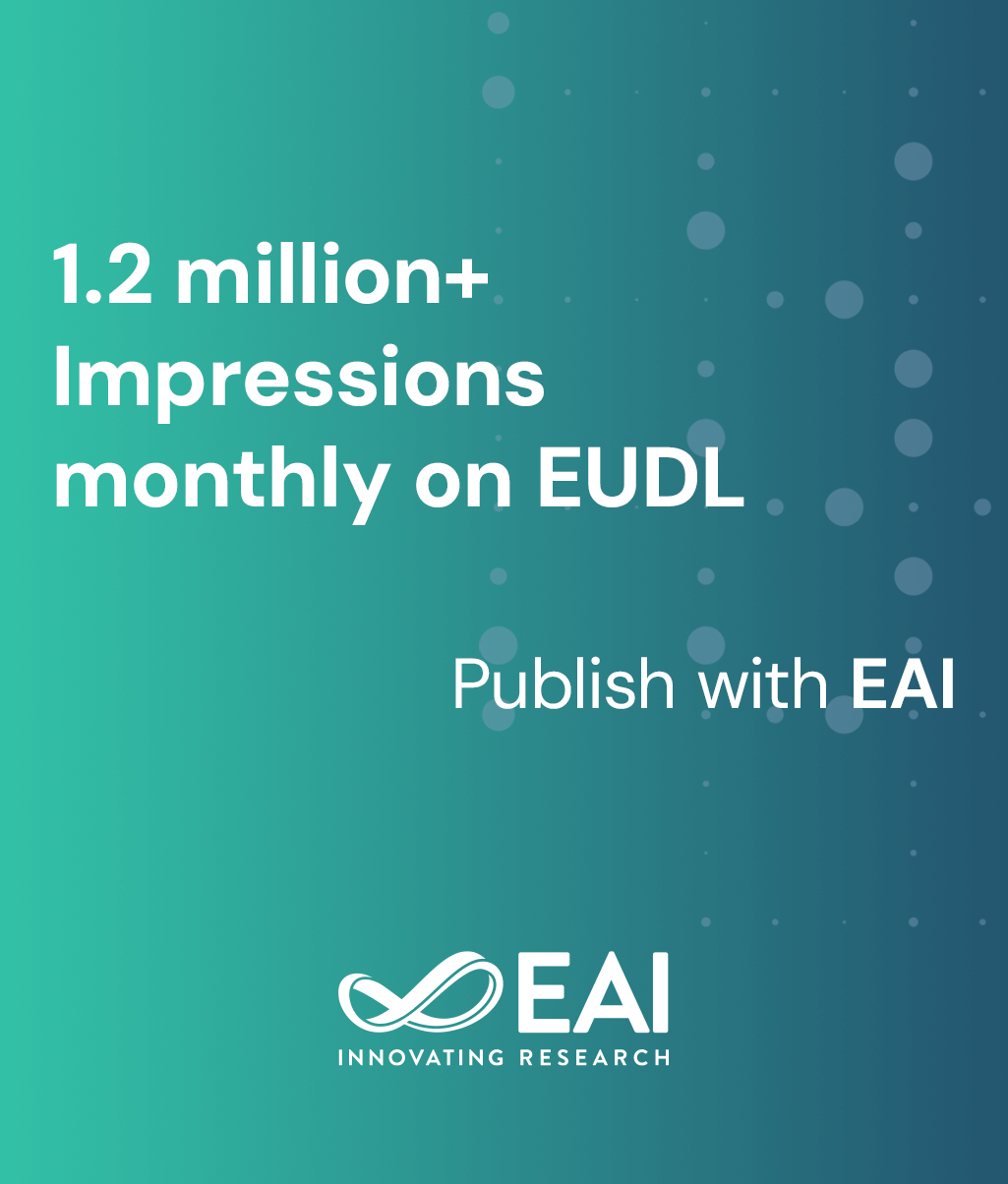
Research Article
Research on sales forecasting of online products based on machine learning methods--Taking Meituan photo studio as an example
@INPROCEEDINGS{10.4108/eai.17-1-2025.2355320, author={Yiran You}, title={Research on sales forecasting of online products based on machine learning methods--Taking Meituan photo studio as an example }, proceedings={Proceedings of the 4th International Conference on Computing Innovation and Applied Physics, CONF-CIAP 2025, 17-23 January 2025, Eskişehir, Turkey}, publisher={EAI}, proceedings_a={CONF-CIAP}, year={2025}, month={4}, keywords={online platform sales prediction random forest regression shap analysis interaction features}, doi={10.4108/eai.17-1-2025.2355320} }
- Yiran You
Year: 2025
Research on sales forecasting of online products based on machine learning methods--Taking Meituan photo studio as an example
CONF-CIAP
EAI
DOI: 10.4108/eai.17-1-2025.2355320
Abstract
With the rapid development of the digital economy, the way consumers purchase goods and enjoy services has gradually shifted to various online platforms. As a leading comprehensive platform, Meituan has accumulated massive market data in the field of photo services. In this paper, based on the Colab environment, eXtreme Gradient Boosting (XGBoost) and Random forest (RF) in the field of machine learning are used to introduce SHapley Additive exPlanations (SHAP) analytics to model and analyze its sales data and predict future sales. The model was optimized using grid search and stochastic search, combined with several metrics such as R-Square (R²), Median Absolute Error (MdAE), and Log Mean Absolute Percentage Error (Log MAPE) for a comprehensive assessment of the model effectiveness. The results show that RF outperforms XGBoost in both initial and optimized models. In particular, with the introduction of interaction features, RF can effectively capture complex nonlinear relationships and significantly improve the accuracy of sales volume prediction, while XGBoost performs poorly in the face of data imbalance and extreme values, with large prediction errors. This study provides an important reference for merchants to optimize their marketing strategies and improve user experience, which has important theoretical value and practical significance.