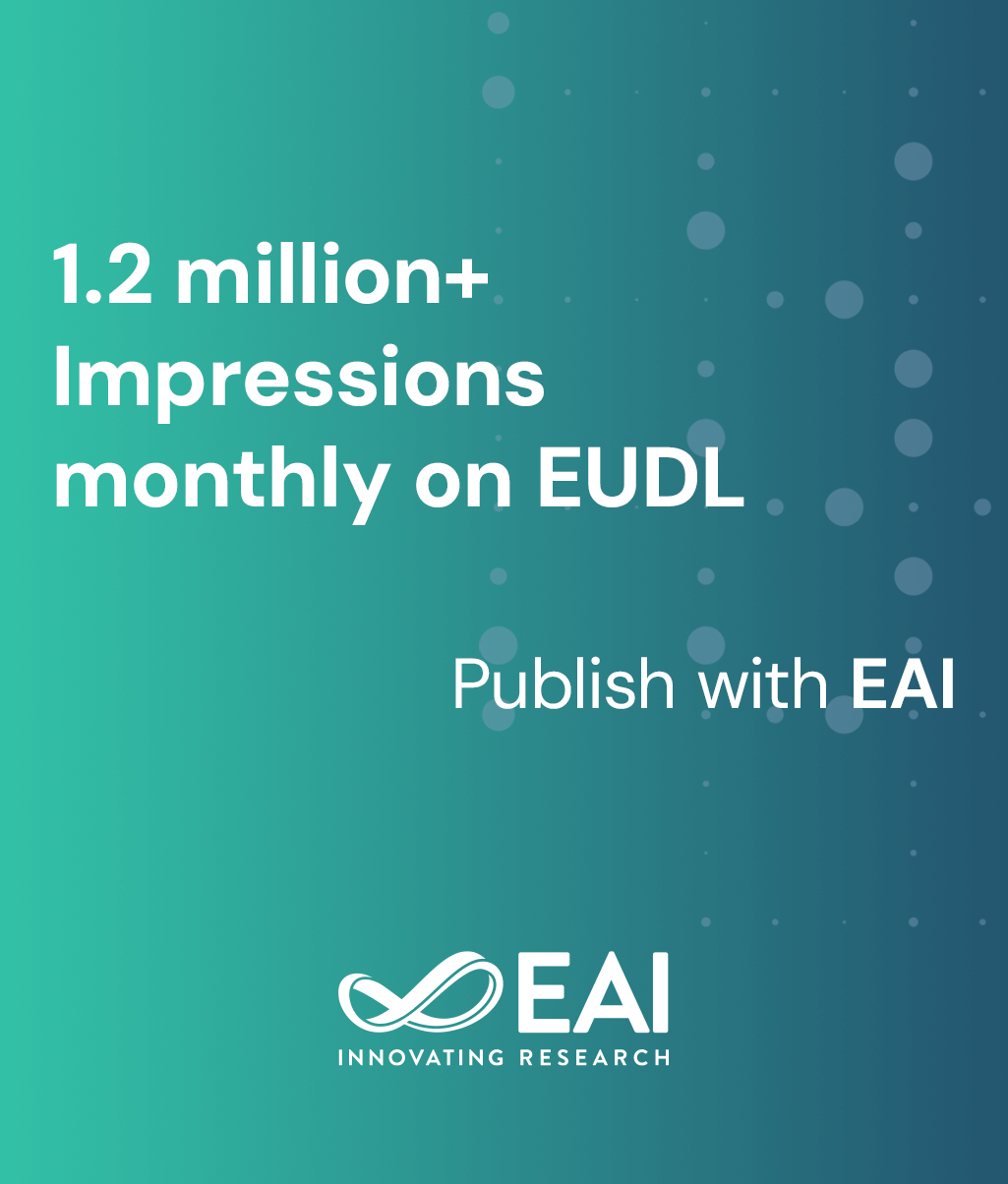
Research Article
A High Performance Glaucoma Screening Technique Using CNN Architecture
@INPROCEEDINGS{10.4108/eai.16-5-2020.2304033, author={J Srinivasa and S Deekshitha and U Kavin Sushil and N S Dhiya and N S Chethan Kumar}, title={A High Performance Glaucoma Screening Technique Using CNN Architecture}, proceedings={Proceedings of the First International Conference on Advanced Scientific Innovation in Science, Engineering and Technology, ICASISET 2020, 16-17 May 2020, Chennai, India}, publisher={EAI}, proceedings_a={ICASISET}, year={2021}, month={1}, keywords={cnn acrima auc precision accuracy specifcity}, doi={10.4108/eai.16-5-2020.2304033} }
- J Srinivasa
S Deekshitha
U Kavin Sushil
N S Dhiya
N S Chethan Kumar
Year: 2021
A High Performance Glaucoma Screening Technique Using CNN Architecture
ICASISET
EAI
DOI: 10.4108/eai.16-5-2020.2304033
Abstract
Glaucoma is a second irreversible eye disease across the world that can lead to blindness, if not treated early. At an early stage, it is very tedious to detect the disease as it does not show any symptoms. So, we build a super-vised method for convolutional neural network to detect the disease as early as possible. In our model ACRIMA data set of 705 images are used where 396 are glaucomatous images and 309 are non-glaucomatous images. The complete data set is divided into 80% for training and 20% for testing. The data set is preprocessed before giving to the CNN model. Our CNN model consists of 8 layers where, 4 convolutional layers for feature extraction and 4 is fully connected layer to classify between glaucomatous and non-glaucomatous imag-es. The best performance is obtained at the learning rate and epochs of 0.0001 and 100.In this work we evaluated the performance of Glaucoma-Deep system by plotting confusion matrix, the sensitivity, specificity, accuracy, precision (PRC) statistical measures and AUC of0.99