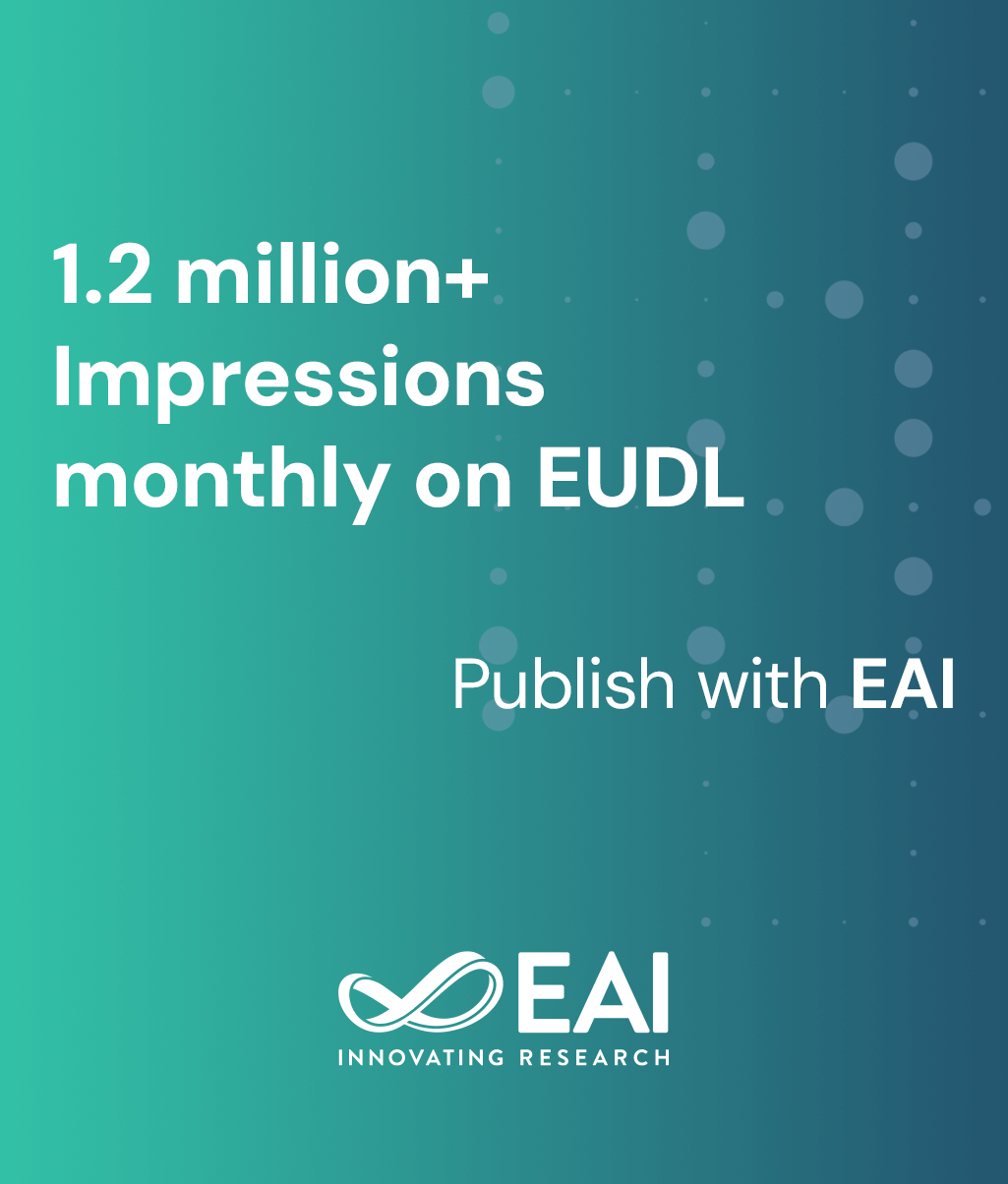
Research Article
Dytective: Diagnosing Risk of Dyslexia with a Game
@INPROCEEDINGS{10.4108/eai.16-5-2016.2263338, author={Luz Rello and Miguel Ballesteros and Abdullah Ali and Miquel Serra and Daniela Alarcón Sánchez and Jeffrey P. Bigham}, title={Dytective: Diagnosing Risk of Dyslexia with a Game}, proceedings={10th EAI International Conference on Pervasive Computing Technologies for Healthcare}, publisher={ACM}, proceedings_a={PERVASIVEHEALTH}, year={2016}, month={6}, keywords={dyslexia; screening; diagnosis; serious games; linguistics}, doi={10.4108/eai.16-5-2016.2263338} }
- Luz Rello
Miguel Ballesteros
Abdullah Ali
Miquel Serra
Daniela Alarcón Sánchez
Jeffrey P. Bigham
Year: 2016
Dytective: Diagnosing Risk of Dyslexia with a Game
PERVASIVEHEALTH
EAI
DOI: 10.4108/eai.16-5-2016.2263338
Abstract
More than 10% of the population has dyslexia, and most are diagnosed only after they fail in school. This work seeks to change this through scalable early detection via machine learning models that predict reading and writing difficulties by watching how people interact with a linguistic web-based game: Dytective. The design of Dytective is based on (i) the empirical linguistic analysis of the errors that people with dyslexia make, (ii) principles of language acquisition, and (iii) specific linguistic skills related to dyslexia. Experiments with 243 children and adults (95 with diagnosed dyslexia) revealed differences in how people with dyslexia read and write. We trained a machine learning model that was able to predict dyslexia with 83% accuracy in a held-out test set with 100 participants. Currently, we are working with schools to put our approach into practice at scale to reduce school failure as a primary way dyslexia is diagnosed.