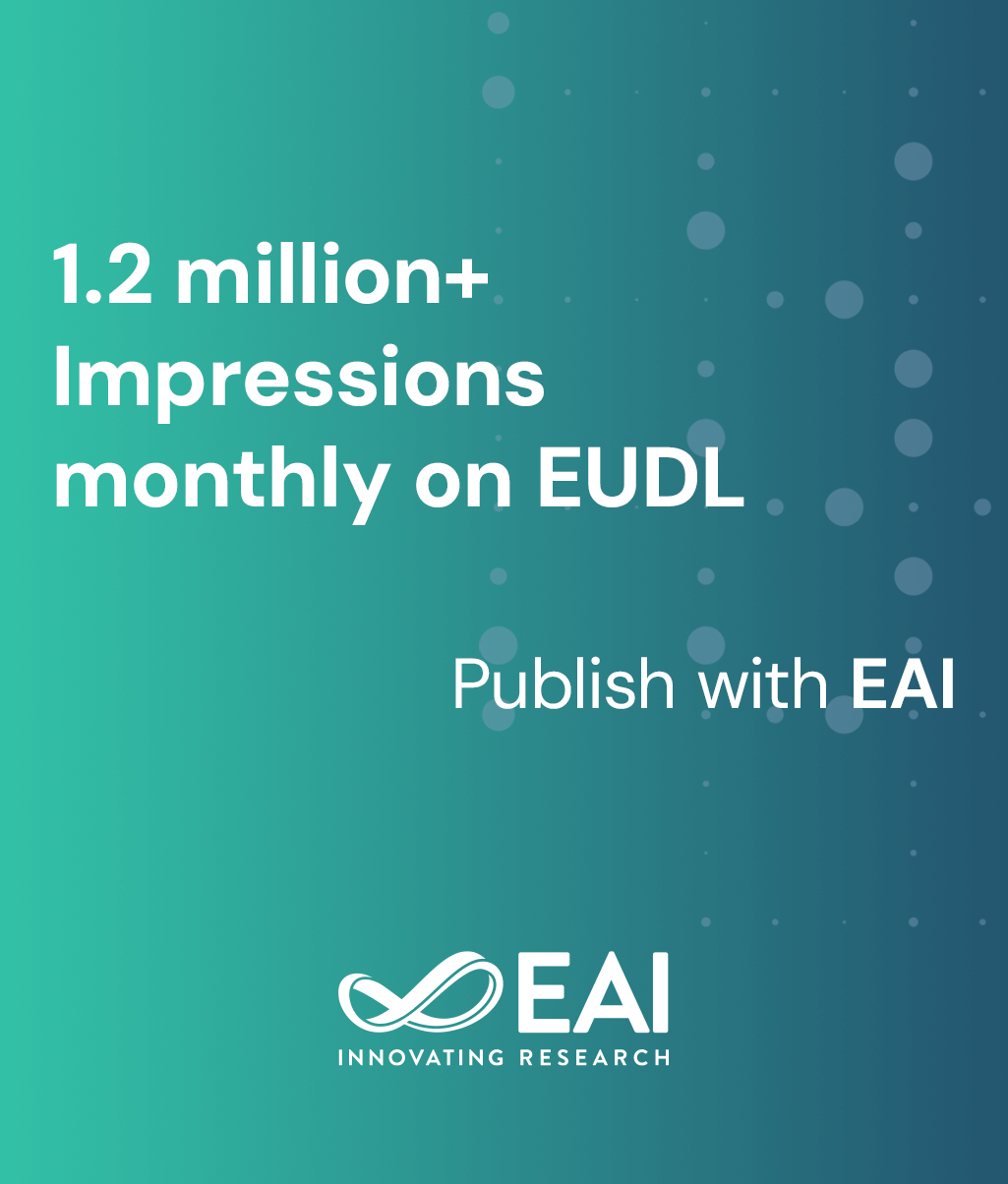
Research Article
Complex human action recognition on daily living environments using wearable inertial sensors
@INPROCEEDINGS{10.4108/eai.16-5-2016.2263332, author={Irvin Hussein L\^{o}pez-Nava and Ang\^{e}lica Mu\`{o}oz-Mel\^{e}ndez}, title={Complex human action recognition on daily living environments using wearable inertial sensors}, proceedings={10th EAI International Conference on Pervasive Computing Technologies for Healthcare}, publisher={ACM}, proceedings_a={PERVASIVEHEALTH}, year={2016}, month={6}, keywords={action recognition; action classification; action variability analysis; inertial sensors; wearable sensors}, doi={10.4108/eai.16-5-2016.2263332} }
- Irvin Hussein López-Nava
Angélica Muñoz-Meléndez
Year: 2016
Complex human action recognition on daily living environments using wearable inertial sensors
PERVASIVEHEALTH
EAI
DOI: 10.4108/eai.16-5-2016.2263332
Abstract
The aim of this study is to evaluate how similar a set of human actions are when they are performed under controlled conditions versus the same set of actions when they are performed under uncontrolled conditions. In this work, we measure and analyze five human complex actions using wearable sensors in both, structured and daily living environments. Three wearable inertial sensor units were used in this study and they were worn by three healthy young subjects on three points of their upper limbs. The complex actions involved in this study are: grooming, cooking, eating, doing housework, and mouth care. Dynamic Time Warping algorithm was used to measure the intra and inter test variability of actions in both environments. Additionally, the results of the application of three supervised classification techniques are compared in terms of true positive rate TPR, true negative rate TNR and F-measure metrics. The classification models were based on time-domain and frequency-domain features extracted from orientation signals. According to the analysis cooking and eating are the actions with highest and lowest variability, respectively. Concerning the classification results, Naïve Bayes and Logistic Regression obtain a TPR of 0.911 using relevant attributes. Our results provide valuable information to measure the similarity of a set of complex actions in daily living environments and to classify them.