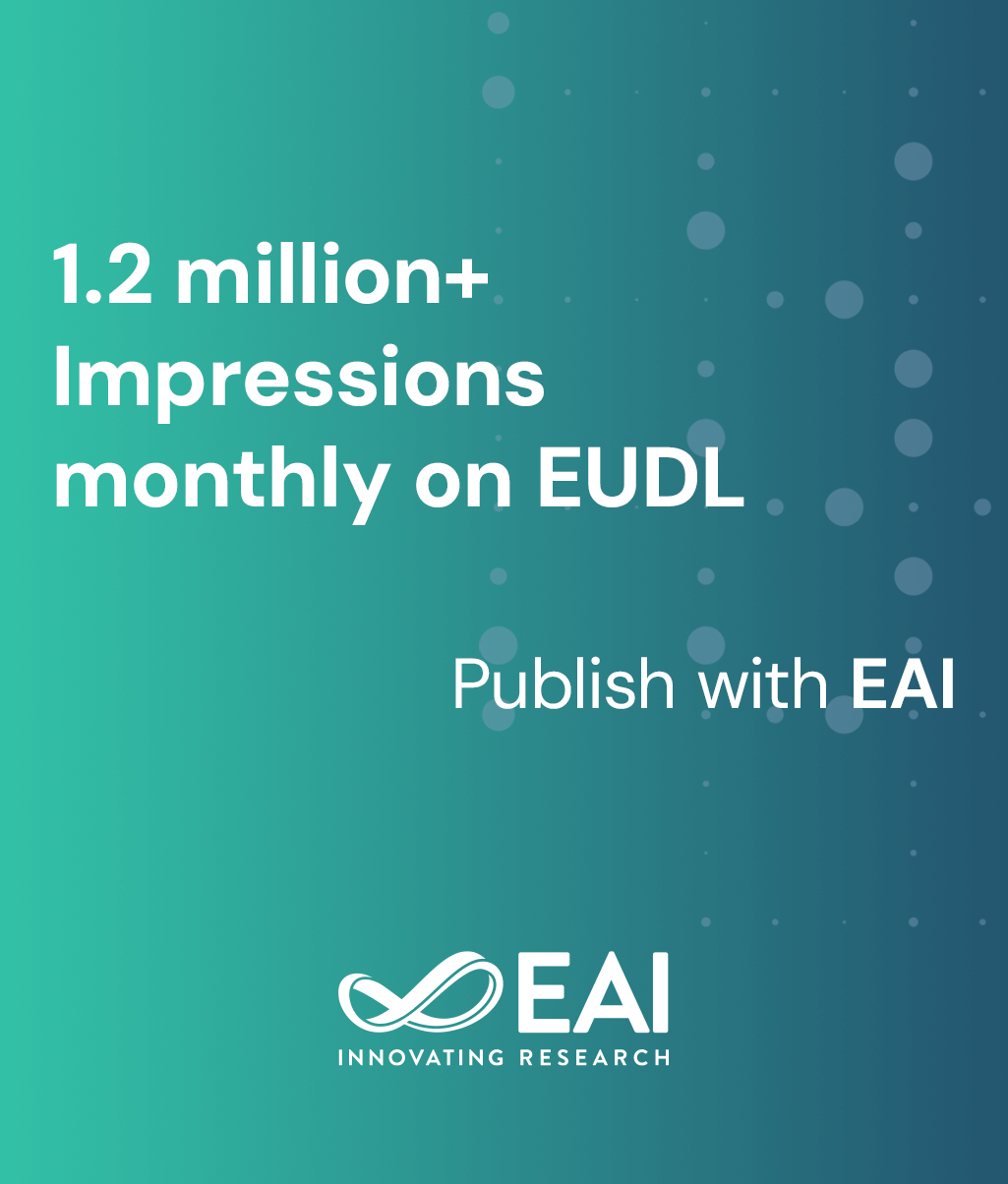
Research Article
Time, Frequency & Complexity Analysis for Recognizing Panic States from Physiologic Time-Series
@INPROCEEDINGS{10.4108/eai.16-5-2016.2263292, author={Jonathan Rubin and Rui Abreu and Shane Ahern and Hoda Eldardiry and Daniel Bobrow}, title={Time, Frequency \& Complexity Analysis for Recognizing Panic States from Physiologic Time-Series}, proceedings={10th EAI International Conference on Pervasive Computing Technologies for Healthcare}, publisher={ACM}, proceedings_a={PERVASIVEHEALTH}, year={2016}, month={6}, keywords={physiological data analysis ecg analysis heart rate variability feature extraction data fusion classification}, doi={10.4108/eai.16-5-2016.2263292} }
- Jonathan Rubin
Rui Abreu
Shane Ahern
Hoda Eldardiry
Daniel Bobrow
Year: 2016
Time, Frequency & Complexity Analysis for Recognizing Panic States from Physiologic Time-Series
PERVASIVEHEALTH
EAI
DOI: 10.4108/eai.16-5-2016.2263292
Abstract
This paper presents results of analysis performed on a physiologic time-series dataset that was collected from a wearable ECG monitoring system worn by individuals who suffer from panic disorder. Models are constructed and evaluated for distinguishing between pathologic and non-pathologic states, including panic (during panic attack), pre-panic (preceding panic attack) and non-panic (outside panic attack window). The models presented use data fusion to combine both traditional time and frequency domain heart rate variability analysis together with nonlinear/complexity analysis. The best performing model is shown to be a random forest classifier that achieves an accuracy of 97.2% and 90.7% for recognizing states of panic and pre-panic, respectively. The models presented have application in pervasive and ubiquitous mobile and wearable health management systems.