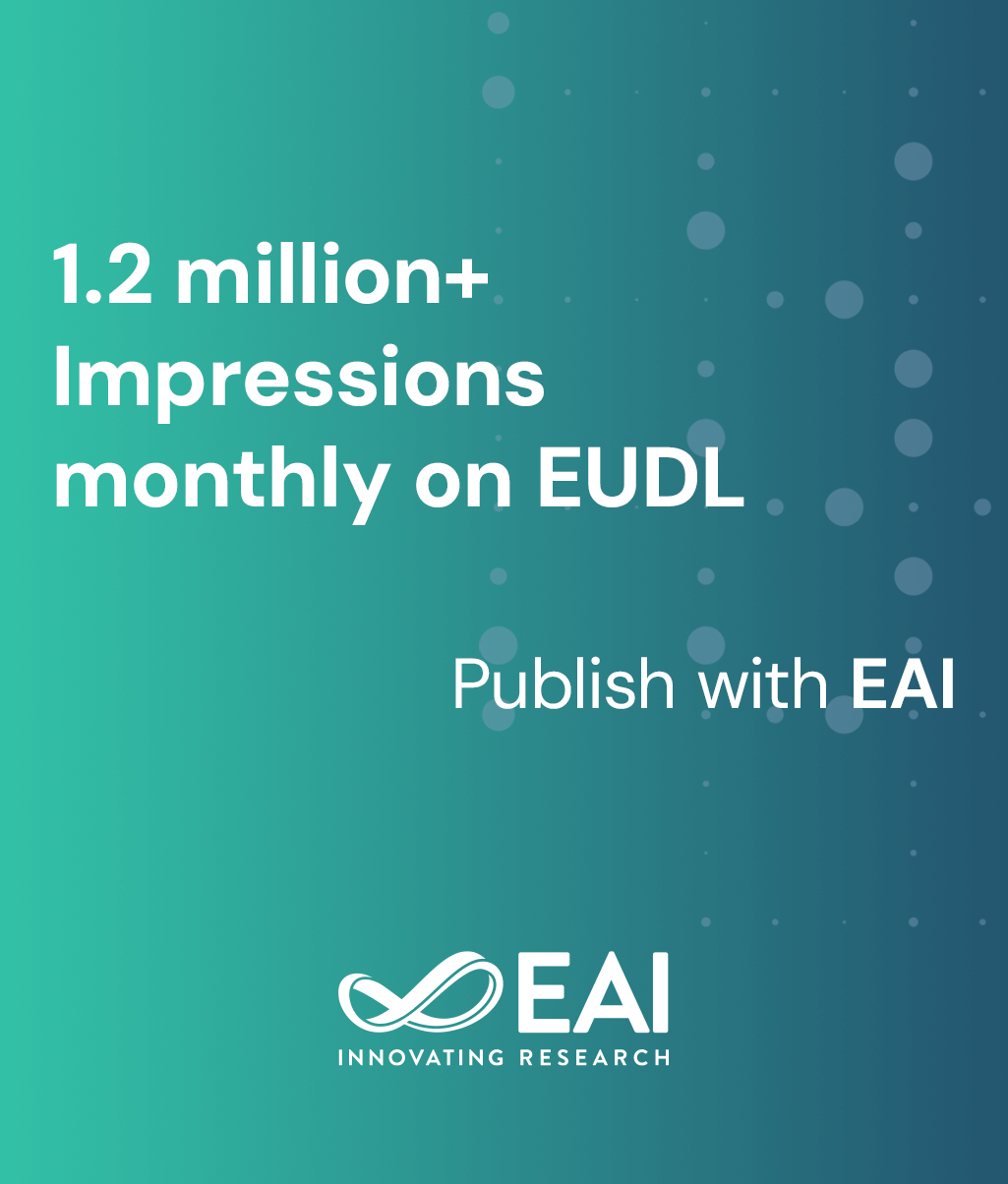
Research Article
Assessment of Nail Images for Preliminary disease detection and classification based on CNN: The New Horizon in Disease Detection in Humans
@INPROCEEDINGS{10.4108/eai.16-4-2022.2318168, author={Shweta Marulkar and Bhawna Narain}, title={Assessment of Nail Images for Preliminary disease detection and classification based on CNN: The New Horizon in Disease Detection in Humans}, proceedings={Proceedings of The International Conference on Emerging Trends in Artificial Intelligence and Smart Systems, THEETAS 2022, 16-17 April 2022, Jabalpur, India}, publisher={EAI}, proceedings_a={THEETAS}, year={2022}, month={6}, keywords={digital image processing nail features analysis disease prediction convolutional neural network (cnn) inceptionv3 inceptionresnetv2 mobilenetv2 efficientnetb0}, doi={10.4108/eai.16-4-2022.2318168} }
- Shweta Marulkar
Bhawna Narain
Year: 2022
Assessment of Nail Images for Preliminary disease detection and classification based on CNN: The New Horizon in Disease Detection in Humans
THEETAS
EAI
DOI: 10.4108/eai.16-4-2022.2318168
Abstract
Digital image processing has wide scope in globe such as military, medical, robotics, forensic science etc. Now a day for such type of applications feature extraction of digital image is important part of processing. Pattern recognition requires the classification of medical imagery. Classification improves the efficiency and accuracy. For the classification process, image preprocessing, fragmentation, and feature extraction might be used. Preliminary disease detection rate depends on all the steps but classification has its own importance in pattern recognition. A Convolutional Neural Network (CNN) model for preliminary disease identification and categorization in humans is presented in this research. The dataset contains 750 images of nails with four symptoms of diseases. We built a CNN model to automate feature extraction and categorization. Color information is frequently employed in the early stages of disease prediction. The filters are applied to three channels based on RGB components in our model. Experiments have said that the proposed approach accurately predicts diseases.