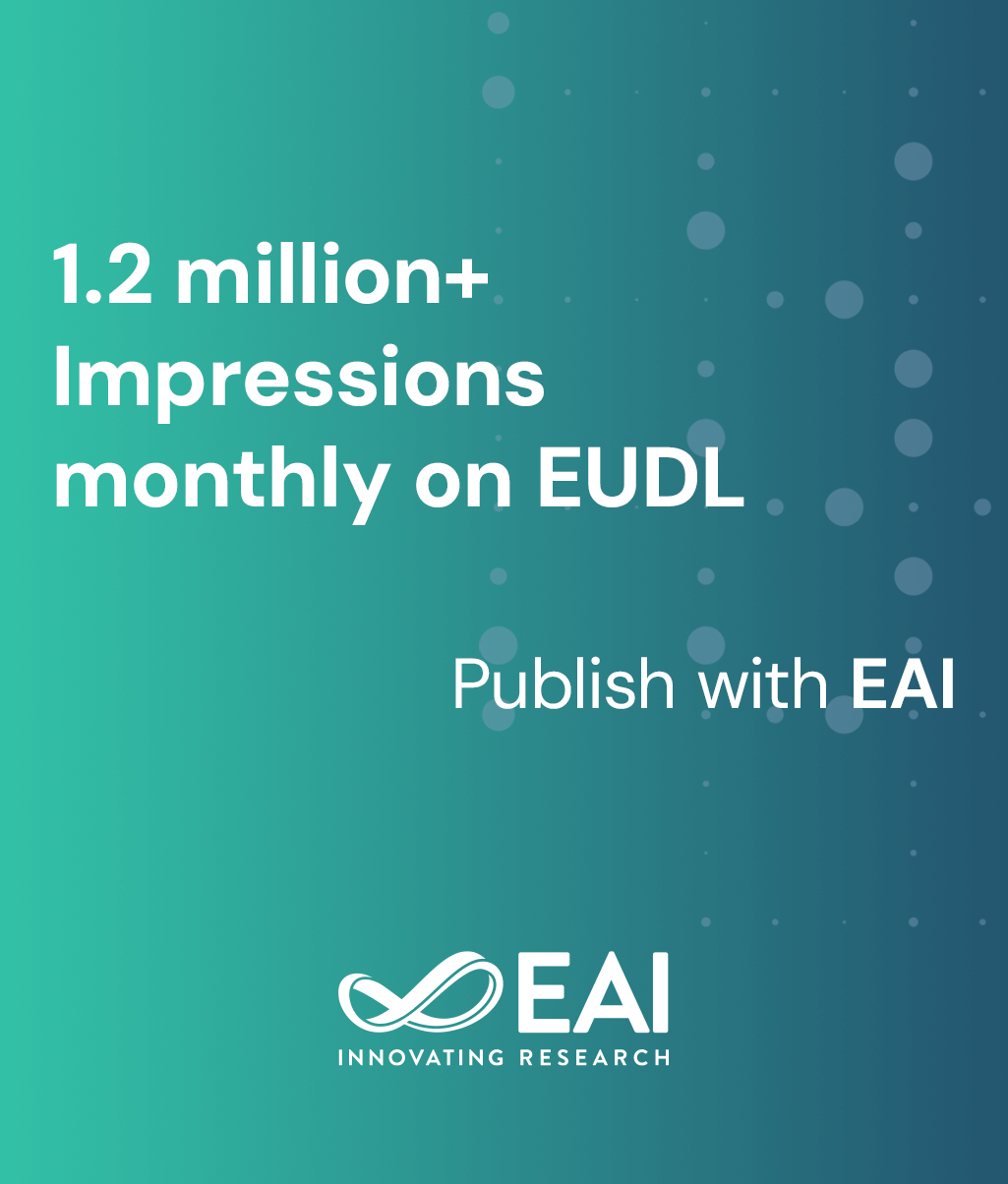
Research Article
Identifying the Signature of Suicidality : A Machine Learning Approach
@INPROCEEDINGS{10.4108/eai.16-4-2022.2318160, author={Md Naimur Rahaman and Sudipto Chaki and Md Shovon Biswas and Milon Biswas and Shamim Ahmed and Md. Julkar Nayeen Mahi and Nuruzzaman Faruqui}, title={Identifying the Signature of Suicidality : A Machine Learning Approach}, proceedings={Proceedings of The International Conference on Emerging Trends in Artificial Intelligence and Smart Systems, THEETAS 2022, 16-17 April 2022, Jabalpur, India}, publisher={EAI}, proceedings_a={THEETAS}, year={2022}, month={6}, keywords={suicide prediction machine learning svm feature engineering}, doi={10.4108/eai.16-4-2022.2318160} }
- Md Naimur Rahaman
Sudipto Chaki
Md Shovon Biswas
Milon Biswas
Shamim Ahmed
Md. Julkar Nayeen Mahi
Nuruzzaman Faruqui
Year: 2022
Identifying the Signature of Suicidality : A Machine Learning Approach
THEETAS
EAI
DOI: 10.4108/eai.16-4-2022.2318160
Abstract
Suicide is a serious problem in today’s world. It would be useful to detect it sooner to lower the death rate. This research proposes a machine learning-based prediction model in this respect. This research uses four traditional machine learning techniques to look at suicidality in various groups of people. To achieve higher levels of accuracy, we have included feature engineering in our suggested model. To accomplish so, a Select-K-Best feature scoring system has been developed. The developed procedure assists in selecting the top 12 scoring characteristics while improving the performance accuracy of the suggested model. In this experimental setup, Random Forest, XGBoost, SVM, and LightGBM prediction algorithms has been used for accuracy prediction. Finally, accuracy levels are measured by fitting our model to the test dataset and found that the XGBoost technique has an accuracy of 89%, which is greater than other implemented ML models.