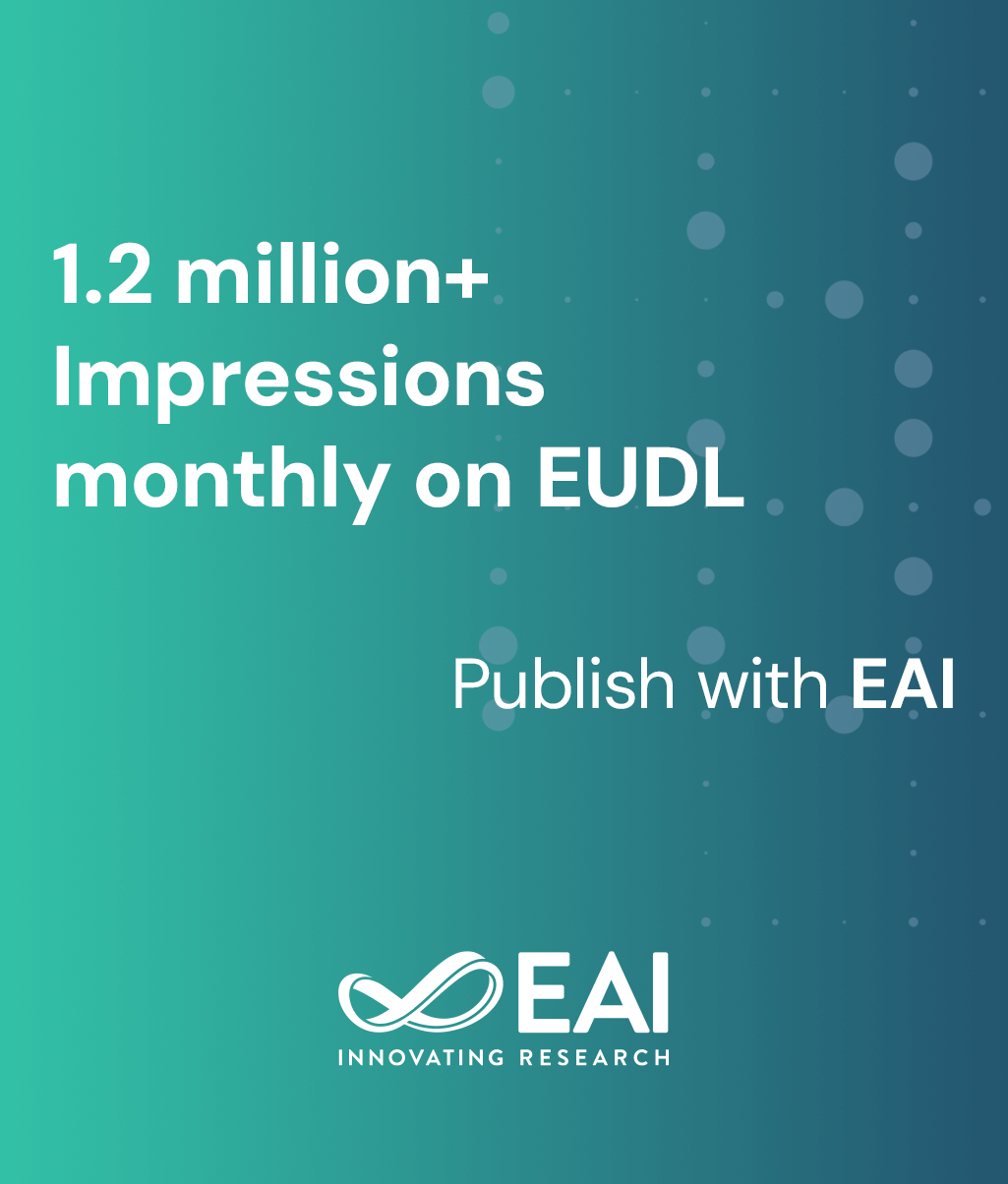
Research Article
Feature Selection in MicroArray Datasets using Manta Ray Foraging Optimization Technique
@INPROCEEDINGS{10.4108/eai.16-12-2022.2326167, author={Devendra K. Tayal and Ramya Neelakantan and Khushi Bhargava}, title={Feature Selection in MicroArray Datasets using Manta Ray Foraging Optimization Technique}, proceedings={Proceedings of the International Conference on Application of AI and Statistical Decision Making for the Business World, ICASDMBW 2022, 16-17 December 2022, Rukmini Devi Institute of Advanced Studies, Delhi, India}, publisher={EAI}, proceedings_a={ICASDMBW}, year={2023}, month={2}, keywords={feature selection (fs) nature inspired algorithms (nia) microarray dataset genetic data manta ray foraging optimization (mrfo)}, doi={10.4108/eai.16-12-2022.2326167} }
- Devendra K. Tayal
Ramya Neelakantan
Khushi Bhargava
Year: 2023
Feature Selection in MicroArray Datasets using Manta Ray Foraging Optimization Technique
ICASDMBW
EAI
DOI: 10.4108/eai.16-12-2022.2326167
Abstract
In this document, we apply the Manta Ray Foraging Optimization technique for Feature Selection in the genetic dataset to identify the relevant features from a high dimensional feature space. MRFO is one of the recent meta-heuristic optimization techniques which has been used for Feature Selection. presence of cancerous tumors in the patient. [10]. This document analyzes the preexisting algorithms developed in the space of Feature Selection using the MRFO algorithm in the GEM dataset. The MRFO method uses three unique search techniques in three different ways. The local search ability of the algorithm is highly aided by the chain foraging behavior; the global search ability is determined by the manta ray cyclone foraging behavior; and the local search ability and convergence rate are greatly improved by the somersault foraging behavior. This document, two separate learning classifiers were used: K-Nearest Neighbors (KNN) classifier and the Random Forest Classifier were used for calculating the fitness