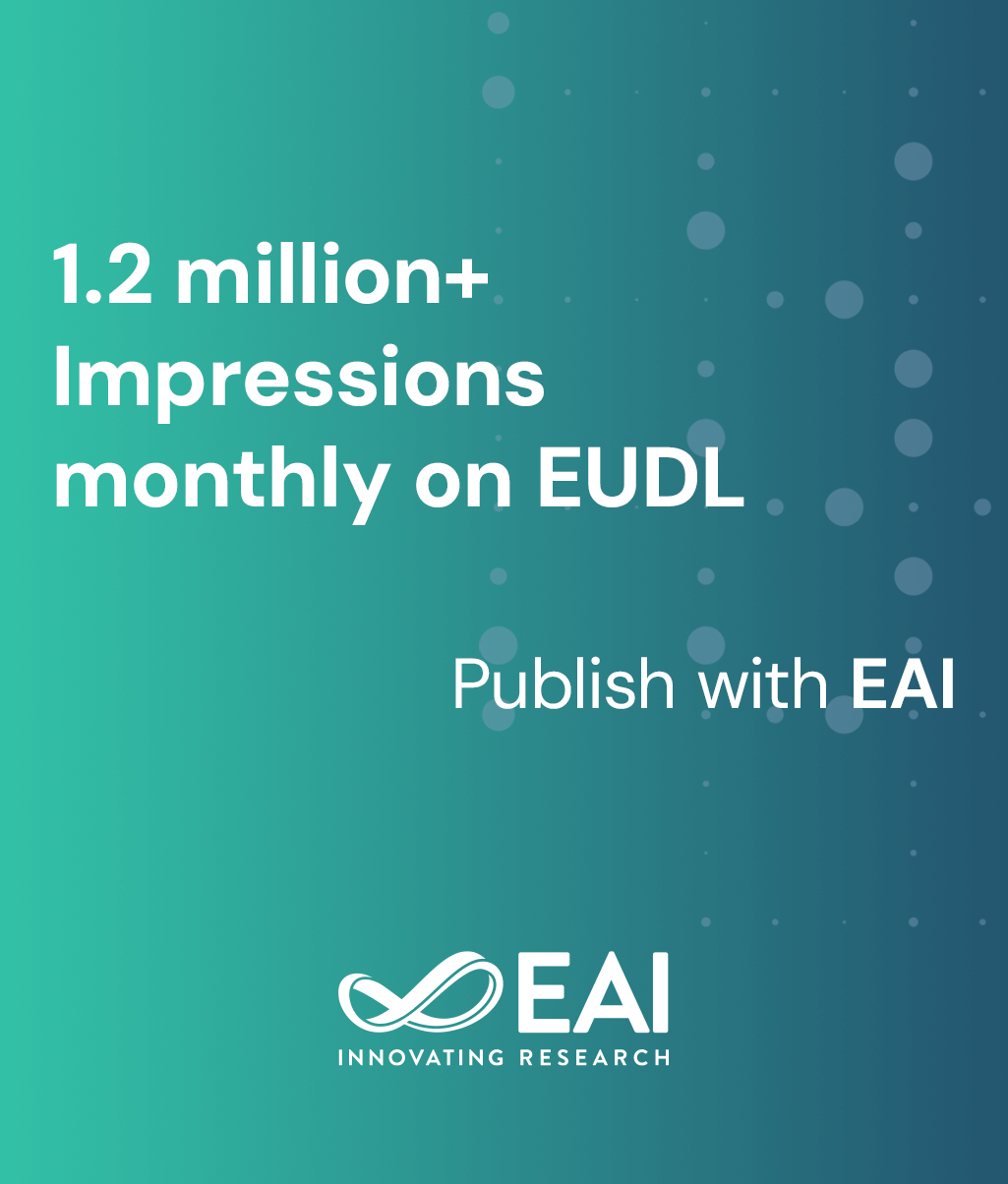
Research Article
PCA Based Hybrid Hyperplane Margin Clustering and Regression for Indoor WLAN Localization
@INPROCEEDINGS{10.4108/eai.15-8-2015.2260714, author={Lingxia Li and Ming Xiang and Mu Zhou and Zengshan Tian}, title={PCA Based Hybrid Hyperplane Margin Clustering and Regression for Indoor WLAN Localization}, proceedings={10th EAI International Conference on Communications and Networking in China}, publisher={IEEE}, proceedings_a={CHINACOM}, year={2015}, month={9}, keywords={wlan localization support vector machine support vector regression hyperplane margin pca}, doi={10.4108/eai.15-8-2015.2260714} }
- Lingxia Li
Ming Xiang
Mu Zhou
Zengshan Tian
Year: 2015
PCA Based Hybrid Hyperplane Margin Clustering and Regression for Indoor WLAN Localization
CHINACOM
IEEE
DOI: 10.4108/eai.15-8-2015.2260714
Abstract
Based on the Principal Component Analysis (PCA), a novel hybrid Support Vector Machine (SVM) Clustering and Regression (SVMCR) approach used for indoor Wireless Local Area Network (WLAN) localization is proposed in this paper. First of all, we rely on the SVM Clustering (SVMC) to conduct the classification for the sake of narrowing down the search space of fingerprints, as well as reducing the computation overhead. Second, the Received Signal Strength (RSS) is processed by using the PCA to extract the RSS features for localization. Finally, we use the Support Vector Regression (SVR) approach to characterize the relations of the RSS distributions and physical locations to achieve the accurate localization. Experimental results in a realistic indoor WLAN test-bed prove that the proposed approach not only reduces the computation and storage overhead, but also provides the high localization accuracy.