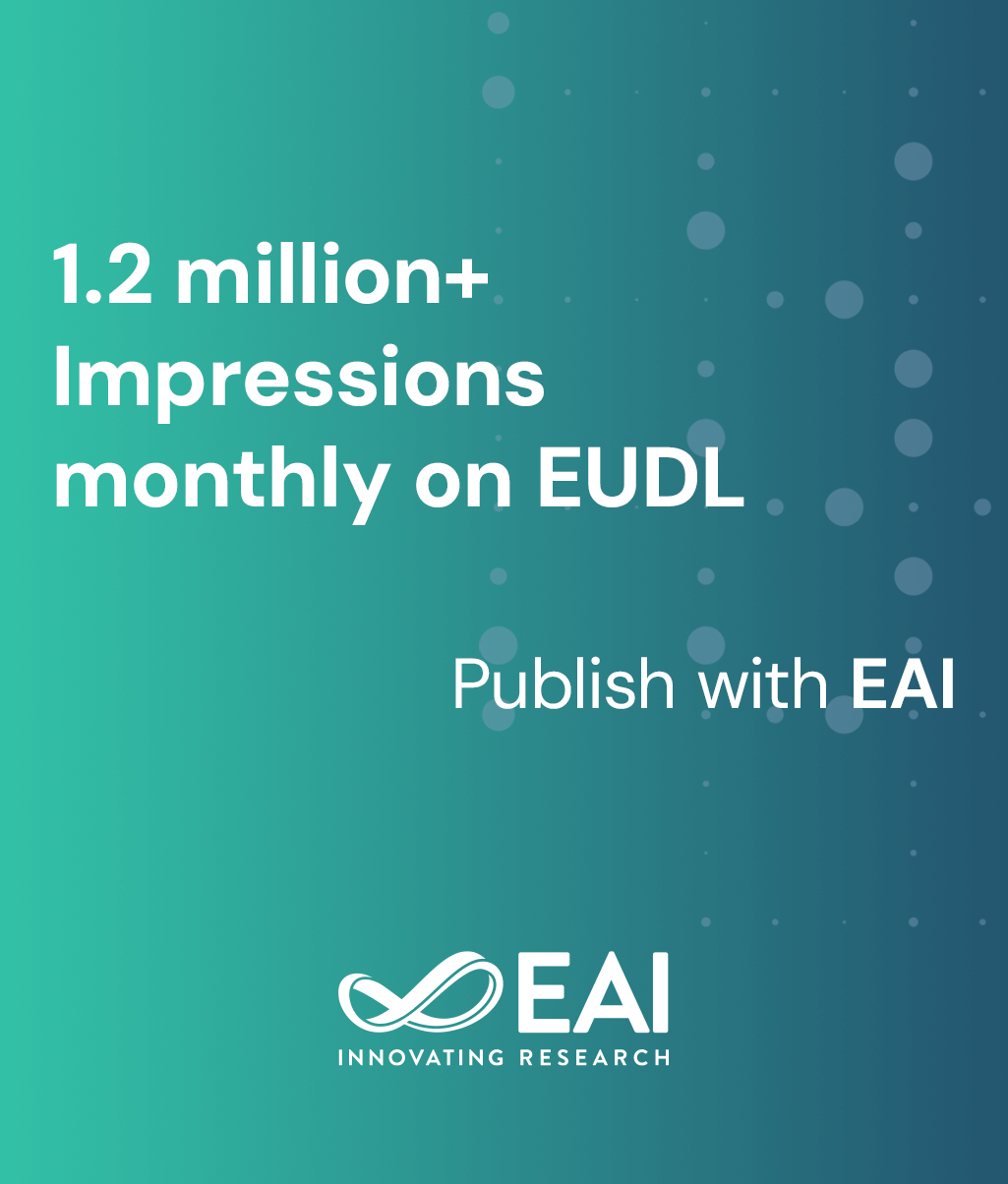
Research Article
Depression Risk Prediction Based on IV-Probit and Transformer for Community Management
@INPROCEEDINGS{10.4108/eai.15-3-2024.2346423, author={Yonghua Yang and Yarui Zhang and Tianyong Chen}, title={Depression Risk Prediction Based on IV-Probit and Transformer for Community Management}, proceedings={Proceedings of the 4th International Conference on Public Management and Intelligent Society, PMIS 2024, 15--17 March 2024, Changsha, China}, publisher={EAI}, proceedings_a={PMIS}, year={2024}, month={6}, keywords={community management offspring quantity gender structure of children mental health of elderly}, doi={10.4108/eai.15-3-2024.2346423} }
- Yonghua Yang
Yarui Zhang
Tianyong Chen
Year: 2024
Depression Risk Prediction Based on IV-Probit and Transformer for Community Management
PMIS
EAI
DOI: 10.4108/eai.15-3-2024.2346423
Abstract
In modern society, community management has gained more attention on children, such as carrying out the three-child policy. On the contrary, the elderly seems be ignored, so this paper researches the correlation between the quantity of children and the well-being of the elderly. Employing data from the China Health and Retirement Longitudinal Study (CHARLS), this research employs an IV-Probit model to elucidate the correlation between the number of offspring and the aged mental well-being, specifically depression symptoms. The result suggests that (1) Chinese people have fertility preference for mix-sex; (2) and parents with more children had a remarkable negative impact on depression rate when endogeneity was not taken into account, but the offspring quantity had a non-significant protective effect on parental mental health when estimated using instrumental variables. In addition, with the aging of society, the mental health problems of the elderly have attracted much attention. This article uses the Transformer model to more accurately capture the diverse factors of depression risk in the elderly and make effective predictions through the advantages of powerful long-range dependency modeling, self-attention mechanism, and multi-head self-attention.