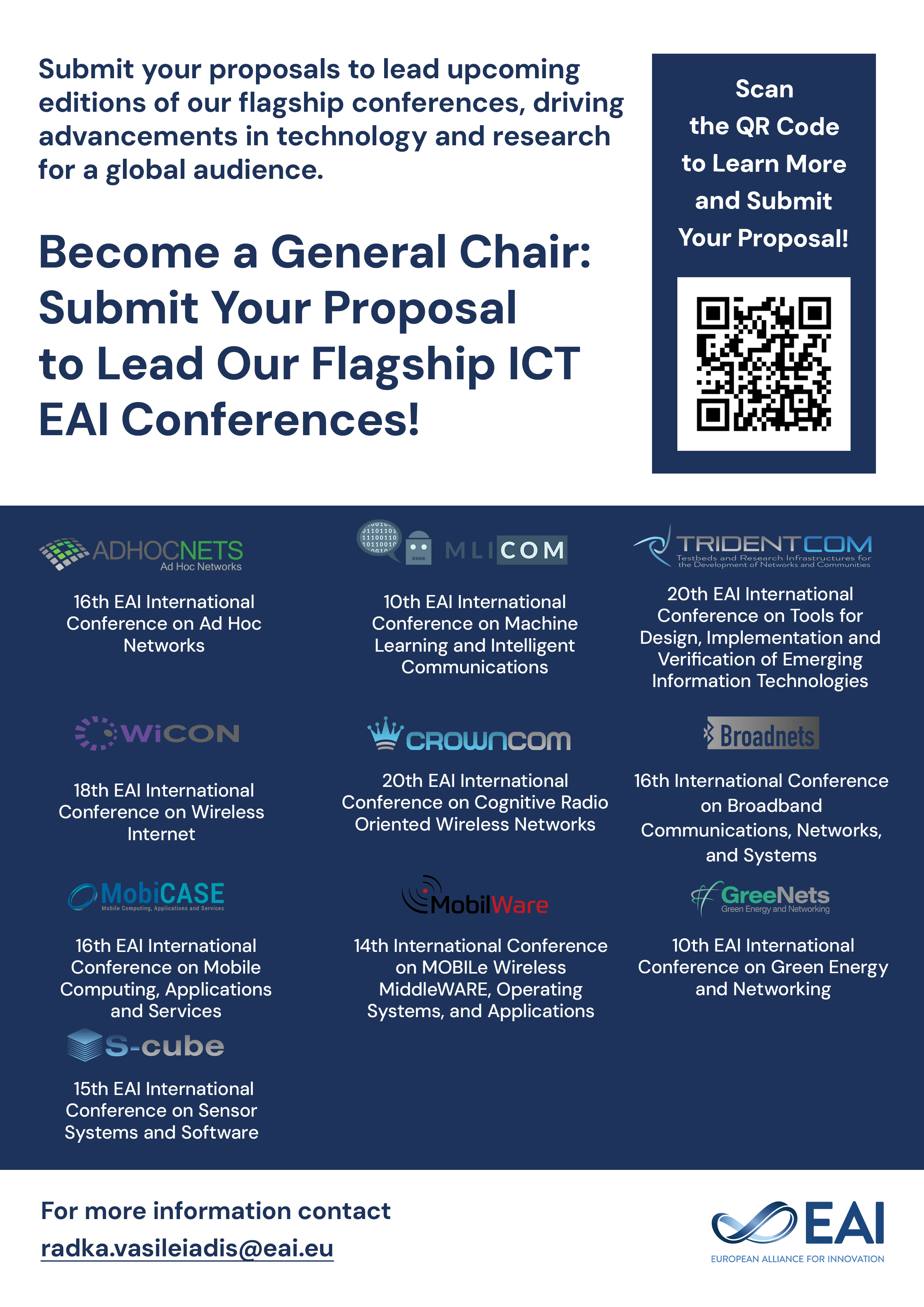
Research Article
Advancing credit scoring models: integrating explainable AI for fair and transparent financial decision-making
@INPROCEEDINGS{10.4108/eai.15-3-2024.2346182, author={Dingyuan Liu and Feng Feng}, title={Advancing credit scoring models: integrating explainable AI for fair and transparent financial decision-making}, proceedings={Proceedings of the 5th International Conference on E-Commerce and Internet Technology, ECIT 2024, March 15--17, 2024, Changsha, China}, publisher={EAI}, proceedings_a={ECIT}, year={2024}, month={5}, keywords={internet finance credit risk economic security machine learning xai}, doi={10.4108/eai.15-3-2024.2346182} }
- Dingyuan Liu
Feng Feng
Year: 2024
Advancing credit scoring models: integrating explainable AI for fair and transparent financial decision-making
ECIT
EAI
DOI: 10.4108/eai.15-3-2024.2346182
Abstract
In the context of the big data era, internet finance is flourishing. However, it is also confronted with an increasing number of credit risk challenges, posing significant threats to economic security. The global nature of the internet means the impact and scope of individual and corporate credit defaults have widened, making it crucial to mitigate credit risk for stable economic development. Today, with the relative maturity of machine learning technology, several popular credit scoring technological methods have emerged, which this article summarizes. However, given the heightened demands, especially in terms of interpretability, placed by many financial institutions and organizations in recent years, previous methods or models cannot be straightforwardly applied and need to be explained in conjunction with Explainable AI (XAI). This paper also proposes a fusion model that simultaneously considers both the accuracy of credit default prediction and interpretability, which can adapt to the current economic environment.