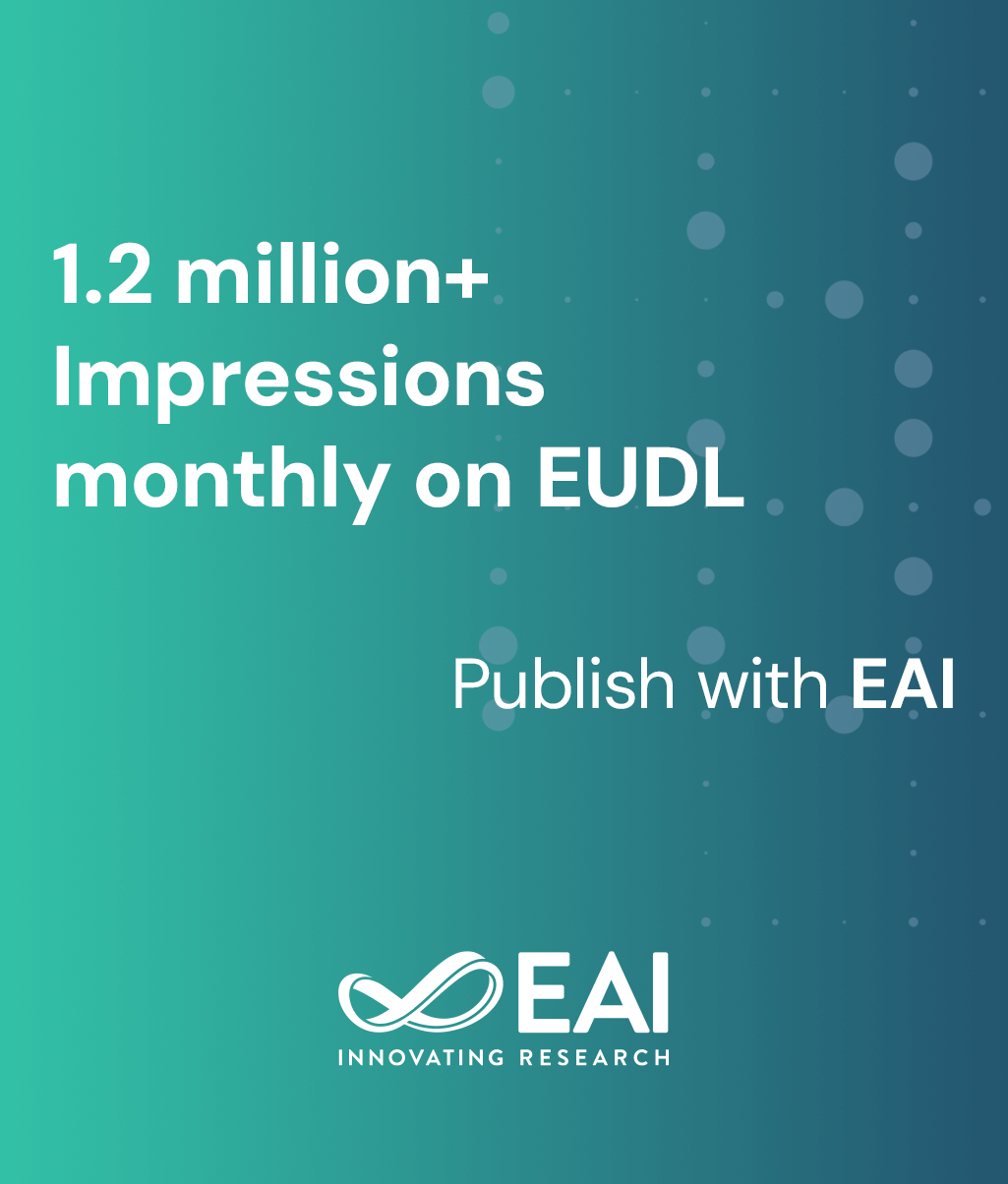
Research Article
Research on the Construction of Hybrid Data Graph Model for Smart Grid Dispatching Based on Multidimensional Data Mining
@INPROCEEDINGS{10.4108/eai.15-12-2023.2345304, author={Jiangang Lu and Ruifeng Zhao and Huijuan Tan and Yue Dai and Chen Wang}, title={Research on the Construction of Hybrid Data Graph Model for Smart Grid Dispatching Based on Multidimensional Data Mining}, proceedings={Proceedings of the 3rd International Conference on Public Management and Big Data Analysis, PMBDA 2023, December 15--17, 2023, Nanjing, China}, publisher={EAI}, proceedings_a={PMBDA}, year={2024}, month={5}, keywords={graph databases; knowledge graphs; multidimensional data mining; smart grids;}, doi={10.4108/eai.15-12-2023.2345304} }
- Jiangang Lu
Ruifeng Zhao
Huijuan Tan
Yue Dai
Chen Wang
Year: 2024
Research on the Construction of Hybrid Data Graph Model for Smart Grid Dispatching Based on Multidimensional Data Mining
PMBDA
EAI
DOI: 10.4108/eai.15-12-2023.2345304
Abstract
The database is more and more unable to meet the grid's demand for fast access and analysis of big data, and the large amount of data has brought greater difficulty in data analysis and processing, and also faces problems such as large grid scheduling, difficult load prediction, and fault detection. In order to ensure the smooth operation of grid scheduling, a research on the construction of a hybrid data graph model for smart grid scheduling based on multidimensional data mining is proposed. This study extracts useful features from raw data collected from various data sources, selects the graph convolutional neural network hybrid data graph model, constructs the data graph model based on the physical topological relationship of the grid and continuously optimizes and updates the model. After the simulation test, this model is able to handle a large amount of data, through the study of smart grid scheduling hybrid data graph model construction can effectively solve the problems such as the difficulty of analyzing and processing a large amount of data.