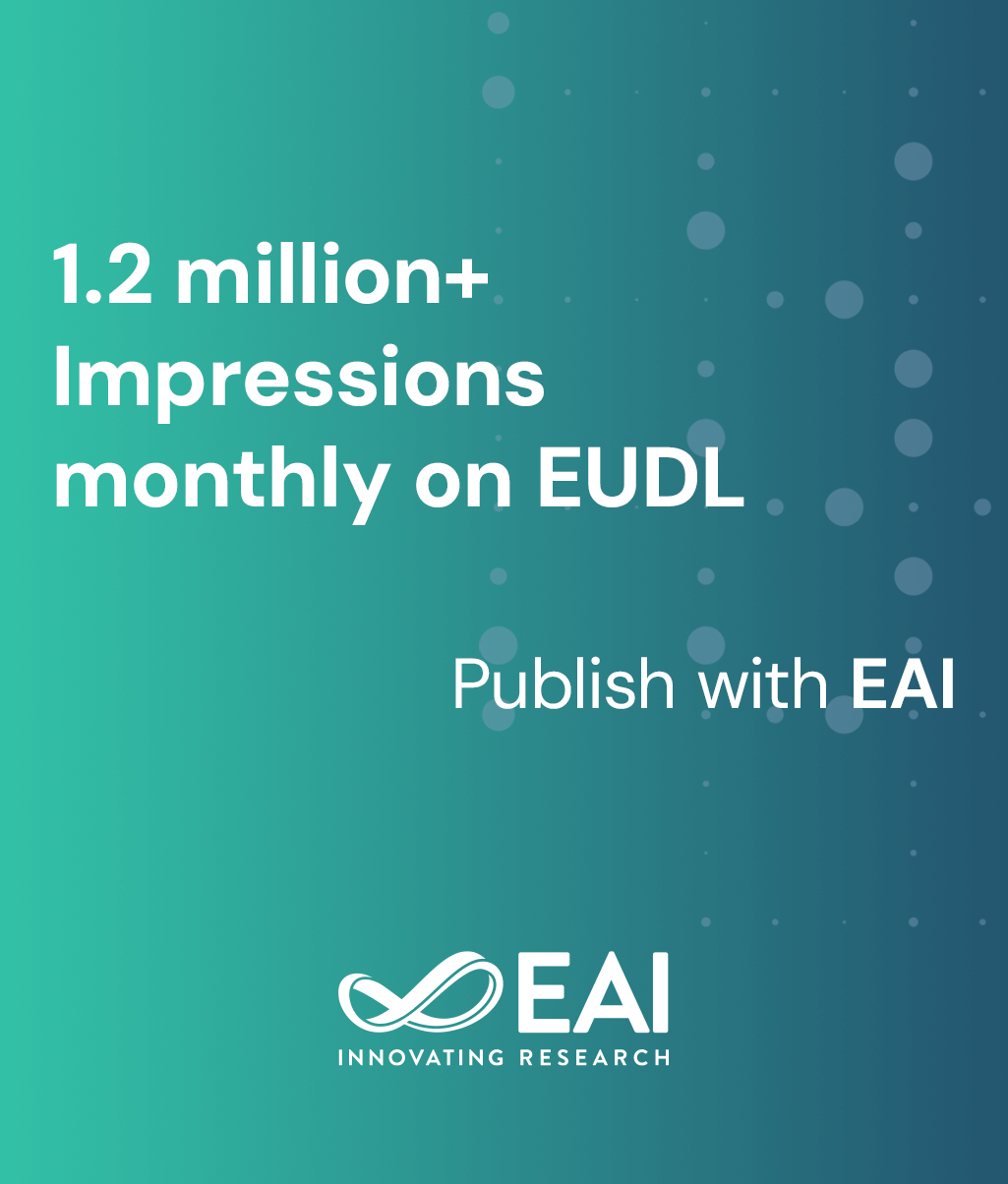
Research Article
Deciphering Lending Behaviors in Peer-to-Peer Platforms: An Integrated Analysis of Emotion, Topic Modeling, and User-defined Occupational Data
@INPROCEEDINGS{10.4108/eai.15-12-2023.2345291, author={Yunxuan Zhang}, title={Deciphering Lending Behaviors in Peer-to-Peer Platforms: An Integrated Analysis of Emotion, Topic Modeling, and User-defined Occupational Data}, proceedings={Proceedings of the 3rd International Conference on Public Management and Big Data Analysis, PMBDA 2023, December 15--17, 2023, Nanjing, China}, publisher={EAI}, proceedings_a={PMBDA}, year={2024}, month={5}, keywords={peer-to-peer (p2p) lending self-disclosure sentiment analysis}, doi={10.4108/eai.15-12-2023.2345291} }
- Yunxuan Zhang
Year: 2024
Deciphering Lending Behaviors in Peer-to-Peer Platforms: An Integrated Analysis of Emotion, Topic Modeling, and User-defined Occupational Data
PMBDA
EAI
DOI: 10.4108/eai.15-12-2023.2345291
Abstract
This research investigates the influence of borrower-generated content on Peer-to-Peer (P2P) lending outcomes, specifically focusing on the sentiment and thematic content of loan descriptions. The central hypothesis posits that these elements of self-disclosure profoundly influence lending determinations and patterns. Utilizing advanced machine learning techniques, variables such as loan descriptions, occupations, loan amounts, interest rates, and loan statuses are examined. Findings highlight strong correlations between sentiment, thematic quality of loan descriptions, and funding success, accentuating the pivotal role of self-reported occupation. These insights illuminate previously uncharted currents within P2P lending dynamics, emphasizing the importance of sentiment analysis, theme selection, and occupational transparency. The research constitutes a sturdy foundation for prospective investigations in this rapidly evolving field.