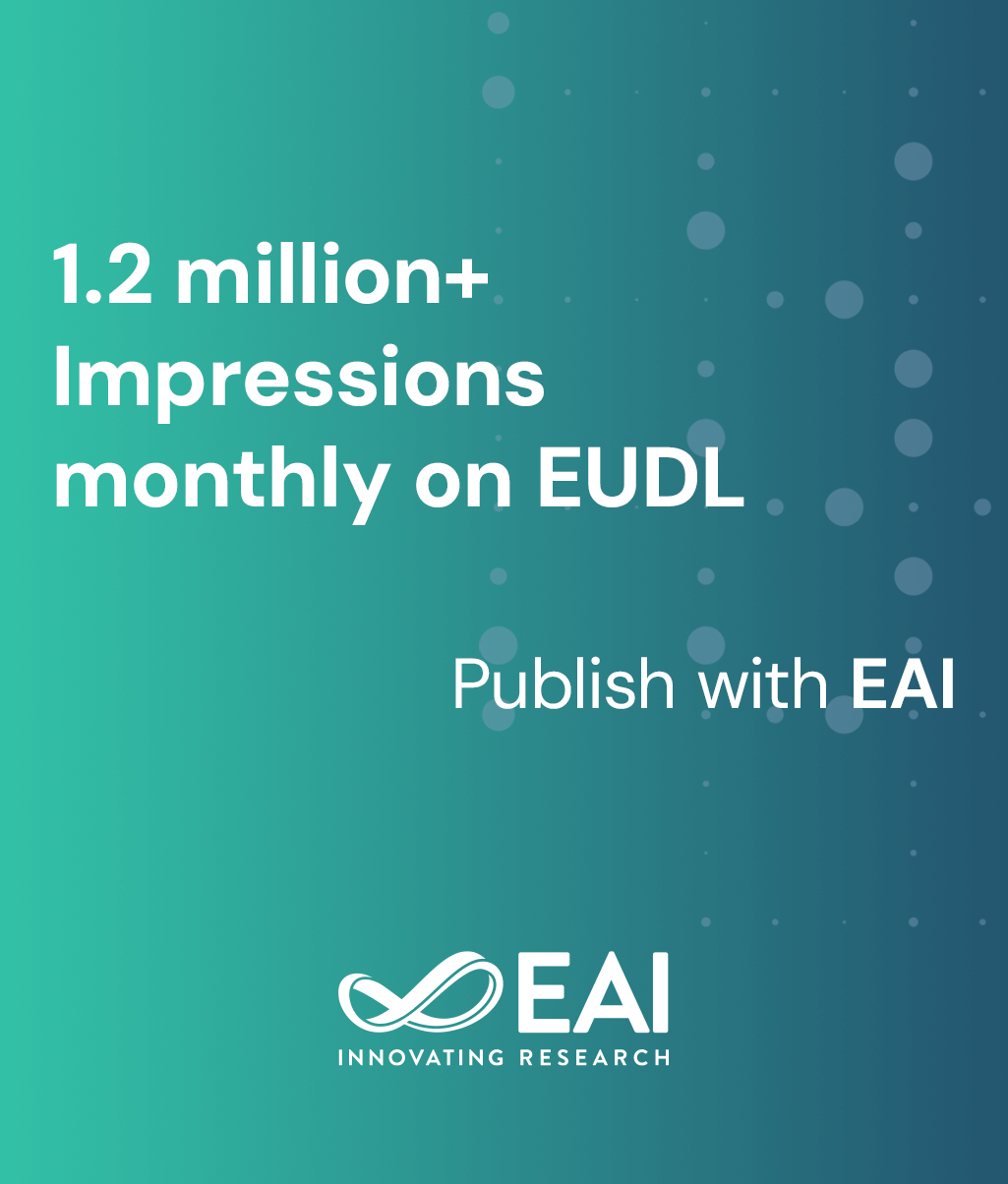
Research Article
Food Watch: Detecting and Characterizing Eating Episodes through Feeding Gestures
@INPROCEEDINGS{10.4108/eai.15-12-2016.2267793, author={Shibo Zhang and Rawan Alharbi and William Stogin and Mohammad Pourhomayoun and Angela Pfammatter and Bonnie Spring and Nabil Alshurafa}, title={Food Watch: Detecting and Characterizing Eating Episodes through Feeding Gestures}, proceedings={11th International Conference on Body Area Networks}, publisher={ACM}, proceedings_a={BODYNETS}, year={2017}, month={4}, keywords={wrist-worn sensors wearables hand-to-mouth gestures overeating inertial sensors}, doi={10.4108/eai.15-12-2016.2267793} }
- Shibo Zhang
Rawan Alharbi
William Stogin
Mohammad Pourhomayoun
Angela Pfammatter
Bonnie Spring
Nabil Alshurafa
Year: 2017
Food Watch: Detecting and Characterizing Eating Episodes through Feeding Gestures
BODYNETS
EAI
DOI: 10.4108/eai.15-12-2016.2267793
Abstract
Obesity, caused primarily by overeating, is a preventable chronic disease yielding staggering healthcare costs. To detect overeating passively, a machine learning framework was designed to detect and accurately count the number of feeding gestures during an eating episode to characterize each eating episode with a feeding gesture count. With the ubiquitous nature of wrist-worn sensors, existing literature has focused on detecting eating-related gestures and eating episodes that are at least five minutes long. In this paper, our objective is to show the potential of commercial smartwatches to be used in detection of eating episodes with short durations confounded by other activities of daily living in order to truly capture all eating episodes in the field. The effect of time-series segmentation and sensing configurations on the accuracy of detecting and characterizing feeding gestures is then analyzed. Finally, the effects of personalized and generalized machine learning models in predicting feeding gestures are compared. Results demonstrate the large within-subject variability of eating, where a generalized user-independent model yields a 75.7\% average F-measure, whereas a personalized user-dependent model yields a 85.7\% average F-measure. This shows the effects of personalized clustering on feeding gesture count, resulting in a root mean square error of 8.4.