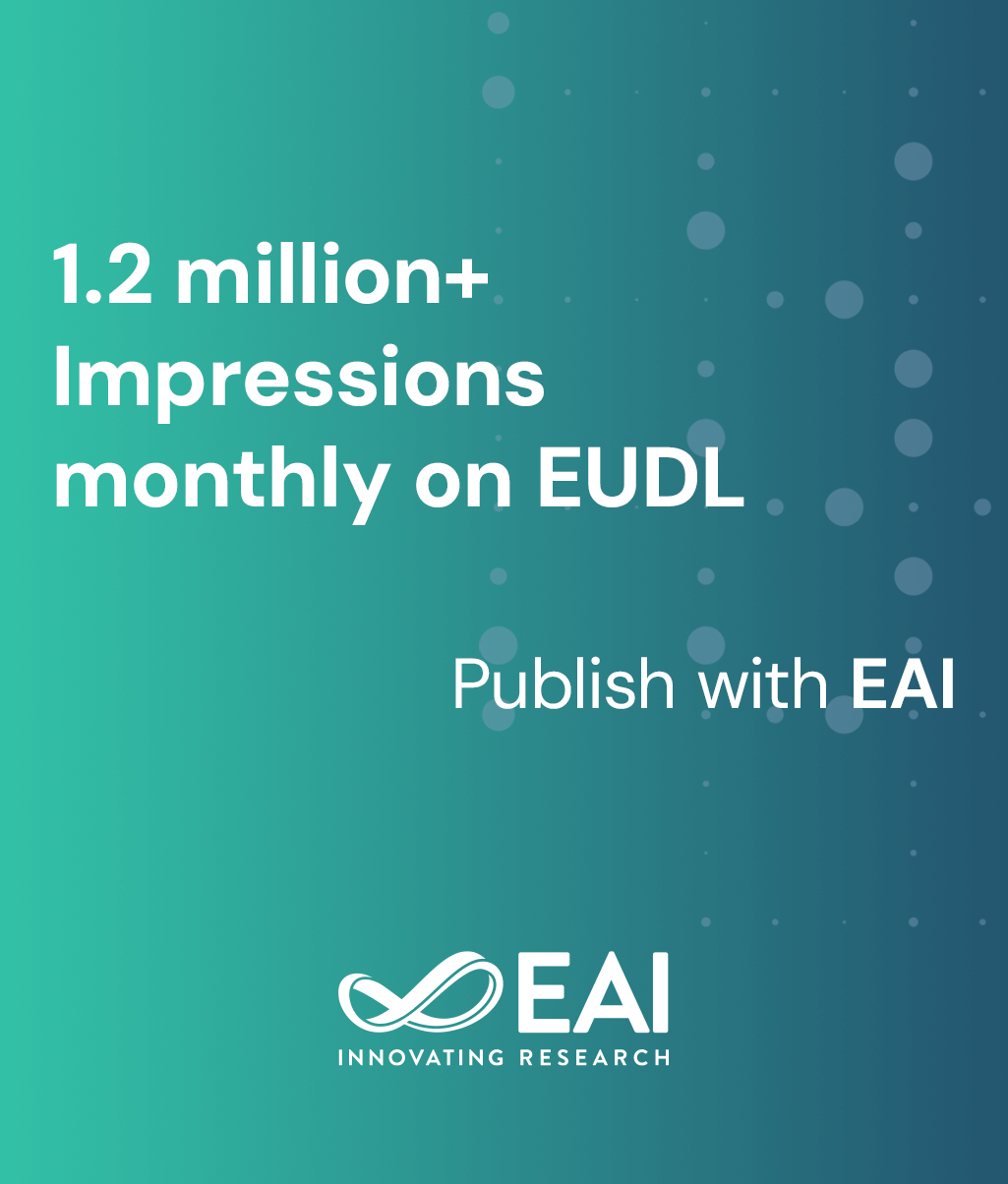
Research Article
Eigenwalk: a Novel Feature for Walk Classification and Fall Prediction
@INPROCEEDINGS{10.4108/eai.15-12-2016.2267645, author={masoud hemmatpour and Renato Ferrero and Bartolomeo Montrucchio and Maurizio Rebaudengo}, title={Eigenwalk: a Novel Feature for Walk Classification and Fall Prediction}, proceedings={11th International Conference on Body Area Networks}, publisher={ACM}, proceedings_a={BODYNETS}, year={2017}, month={4}, keywords={fall prevention health care accelerometer gyroscope smartphone}, doi={10.4108/eai.15-12-2016.2267645} }
- masoud hemmatpour
Renato Ferrero
Bartolomeo Montrucchio
Maurizio Rebaudengo
Year: 2017
Eigenwalk: a Novel Feature for Walk Classification and Fall Prediction
BODYNETS
EAI
DOI: 10.4108/eai.15-12-2016.2267645
Abstract
Predicting a fall is one of the most promising approach to avoid it. Different studies strive to classify abnormal and normal walks in order to predict a fall before its occurrence. This study introduces eigenwalk, a novel feature based on the principal components of the accelerometer and gyroscope signals. This feature, in conjunction with a random forest classifier, is able to distinguish walk patterns and to estimate a fall risk. As the accelerometer and the gyroscope embedded in a smartphone are recognized to be precise enough for fall avoidance systems, they have been exploited in an experimental analysis in order to compare the proposed approach with the most recent ones. The results have shown that the new feature in combination with the random forest classification outperforms state-of-the-art approaches, by improving the accuracy up to 98.6%.