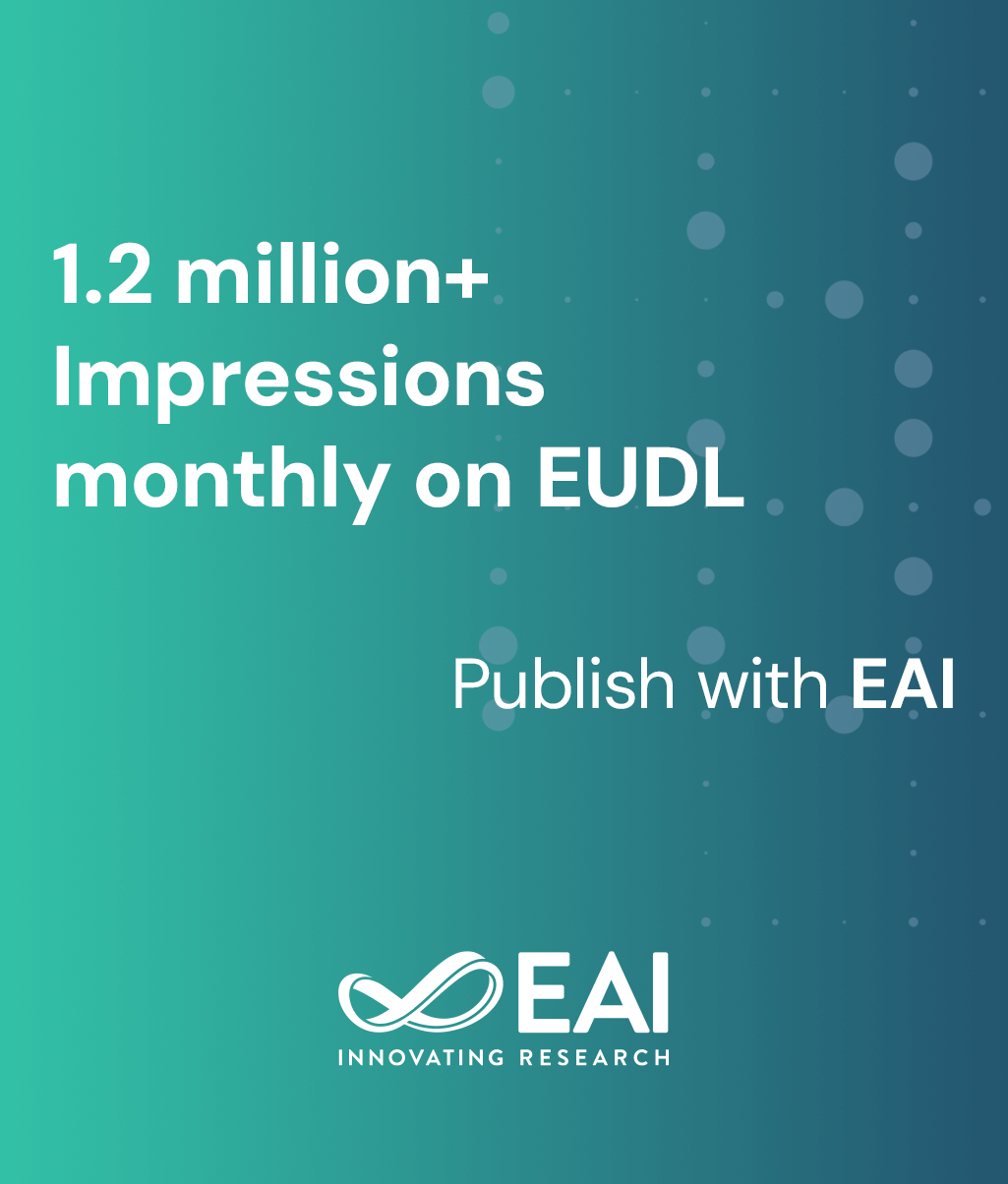
Research Article
Optimal Elbow Angle for Extraction of sEMG and MMG Signals During Dynamic Fatiguing Contractions
@INPROCEEDINGS{10.4108/eai.14-10-2015.2261709, author={Mohamed Al-mulla and Francisco Sepulveda and Bader Al-Bader}, title={Optimal Elbow Angle for Extraction of sEMG and MMG Signals During Dynamic Fatiguing Contractions}, proceedings={5th EAI International Conference on Wireless Mobile Communication and Healthcare - "Transforming healthcare through innovations in mobile and wireless technologies"}, publisher={ACM}, proceedings_a={MOBIHEALTH}, year={2015}, month={12}, keywords={wearble muscle fatigue semg mmg}, doi={10.4108/eai.14-10-2015.2261709} }
- Mohamed Al-mulla
Francisco Sepulveda
Bader Al-Bader
Year: 2015
Optimal Elbow Angle for Extraction of sEMG and MMG Signals During Dynamic Fatiguing Contractions
MOBIHEALTH
ICST
DOI: 10.4108/eai.14-10-2015.2261709
Abstract
Electromyography (sEMG) and Mechanomyography (MMG) signals of the biceps muscle acquired from thirteen subjects undertaking fatiguing dynamic contraction. Both the sEMG and MMG signals were divided into Non-Fatigue and Fatigue segments. A genetic algorithm generated elbow angles which best distinguished (using DBi) between Non-Fatigue and Fatigue contents of the signal. Determining the optimal elbow angle for feature extraction utilised in the evolutionary process was based on 70\% of the conducted trials (both sEMG and MMG). The best individual evolution run was selected based on the optimal elbow angle for separation between the fatigue classes, before testing the classification performance on the remaining on 30\% of the data. The results were compared to eight parameters to measure the classification performance. Results consistently show that the pseudo-wavelet generated the highest classification results of the fatigue content in both signal modalities; however, the sEMG signal performed better than the MMG signals.