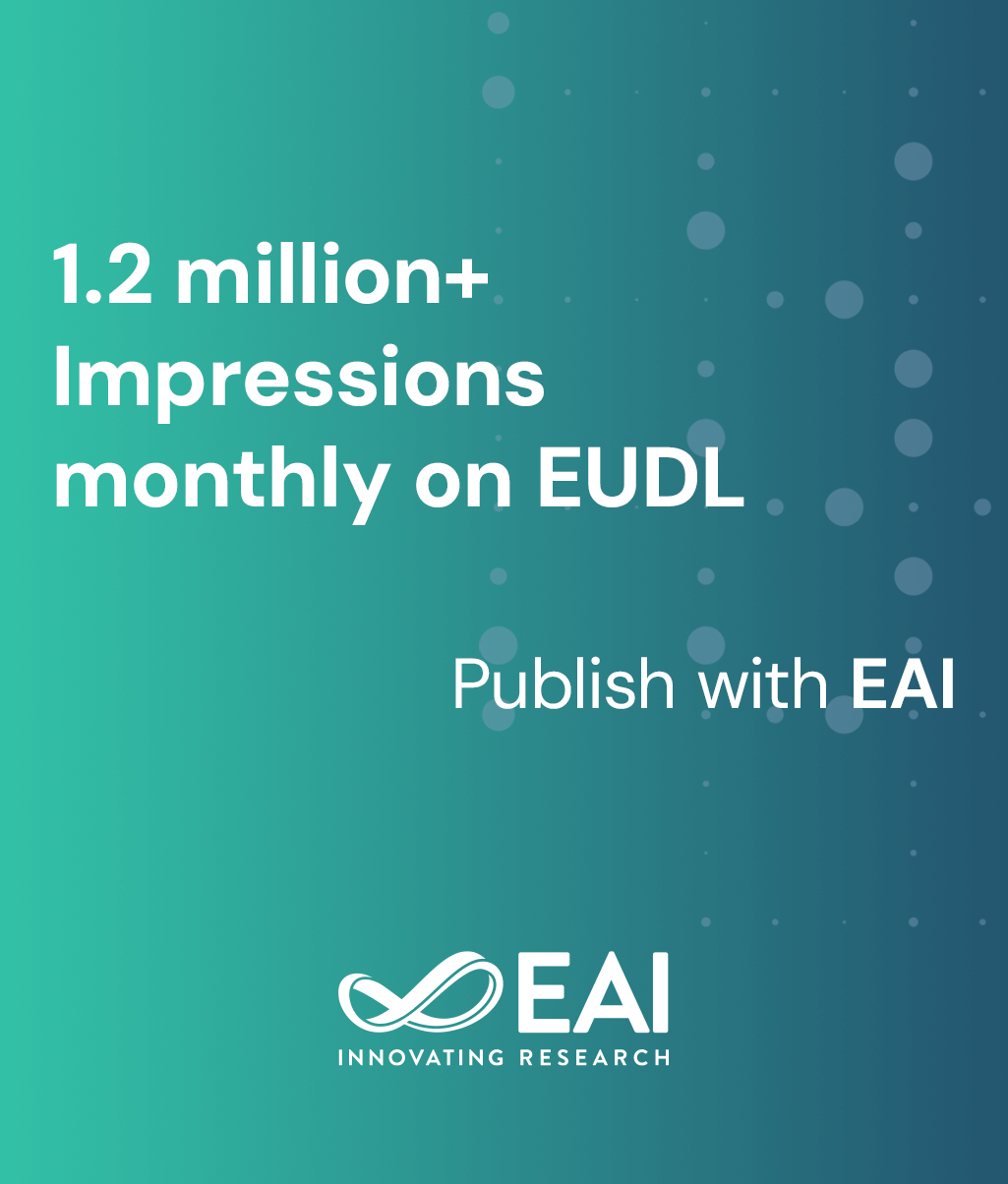
Research Article
Predicting Diabetes Mellitus and Analysing Risk-Factors Correlation
@ARTICLE{10.4108/eai.13-7-2018.164173, author={Md. Faisal Faruque and Asaduzzaman Asaduzzaman and Syed Md. Minhaz Hossain and Md. Hasan Furhad and Iqbal H. Sarker}, title={Predicting Diabetes Mellitus and Analysing Risk-Factors Correlation}, journal={EAI Endorsed Transactions on Pervasive Health and Technology}, volume={5}, number={20}, publisher={EAI}, journal_a={PHAT}, year={2019}, month={11}, keywords={health informatics, machine learning, diabetes, classification, e-health services}, doi={10.4108/eai.13-7-2018.164173} }
- Md. Faisal Faruque
Asaduzzaman Asaduzzaman
Syed Md. Minhaz Hossain
Md. Hasan Furhad
Iqbal H. Sarker
Year: 2019
Predicting Diabetes Mellitus and Analysing Risk-Factors Correlation
PHAT
EAI
DOI: 10.4108/eai.13-7-2018.164173
Abstract
INTRODUCTION: Diabetes mellitus is a common disease of the human body caused by a group of metabolic disorders where the sugar levels exceed a prolonged period, and that is very high than the usual time. It not only affects different organs of the human body but also harms a large number of the body system, in particular the blood veins and nerves.
OBJECTIVES: Early predictions of this phenomenon can help us to control the disease and also to save human life. For achieving the goal, this research work mainly explores various risk factors such as kidney complications, blood pressure, hearing loss, and skin complications related to this disease using machine learning techniques and make a decision.
METHODS: Machine learning techniques provide an efficient result to extract knowledge by constructing predicting models from diagnostic medical datasets collected from 200 diabetic patients from the Medical Centre Chittagong, Bangladesh using 16 attributes. Obtaining knowledge from such data can be useful to predict diabetes. In this work, we perform four popular machine learning algorithms, such as Support Vector Machine (SVM), Naive Bayes (NB), K-Nearest Neighbour (KNN) and C4.5 Decision Tree (DT), on adult population dataset to predict Diabetes Mellitus.
RESULTS: C4.5 Decision Tree performs better than other algorithms for predicting diabetes with 73.5% accuracy, 72% F-measure, and 0.69 of AUC (area under ROC curve). Besides, we determine the correlation between different risk factors of Diabetes Mellitus. The highest correlation is 0.81 for blood pressure (Hypertension) complications with diabetes.
CONCLUSION: In this study, both positive and negative correlation has been established between the various risk factors and diabetes. There is a positive correlation for predicting kidney complications (Nephropathy) and blood pressure (Hypertension) complications and a negative correlation at predicting hearing loss and skin complications (diabetes dermopathy) from diabetic patients. It helps a patient to be aware of the risk factors related to diabetes.
Copyright © 2019 Md. Faisal Faruque et al., licensed to EAI. This is an open access article distributed under the terms of the Creative Commons Attribution licence (http://creativecommons.org/licenses/by/3.0/), which permits unlimited use, distribution, and reproduction in any medium so long as the original work is properly cited.