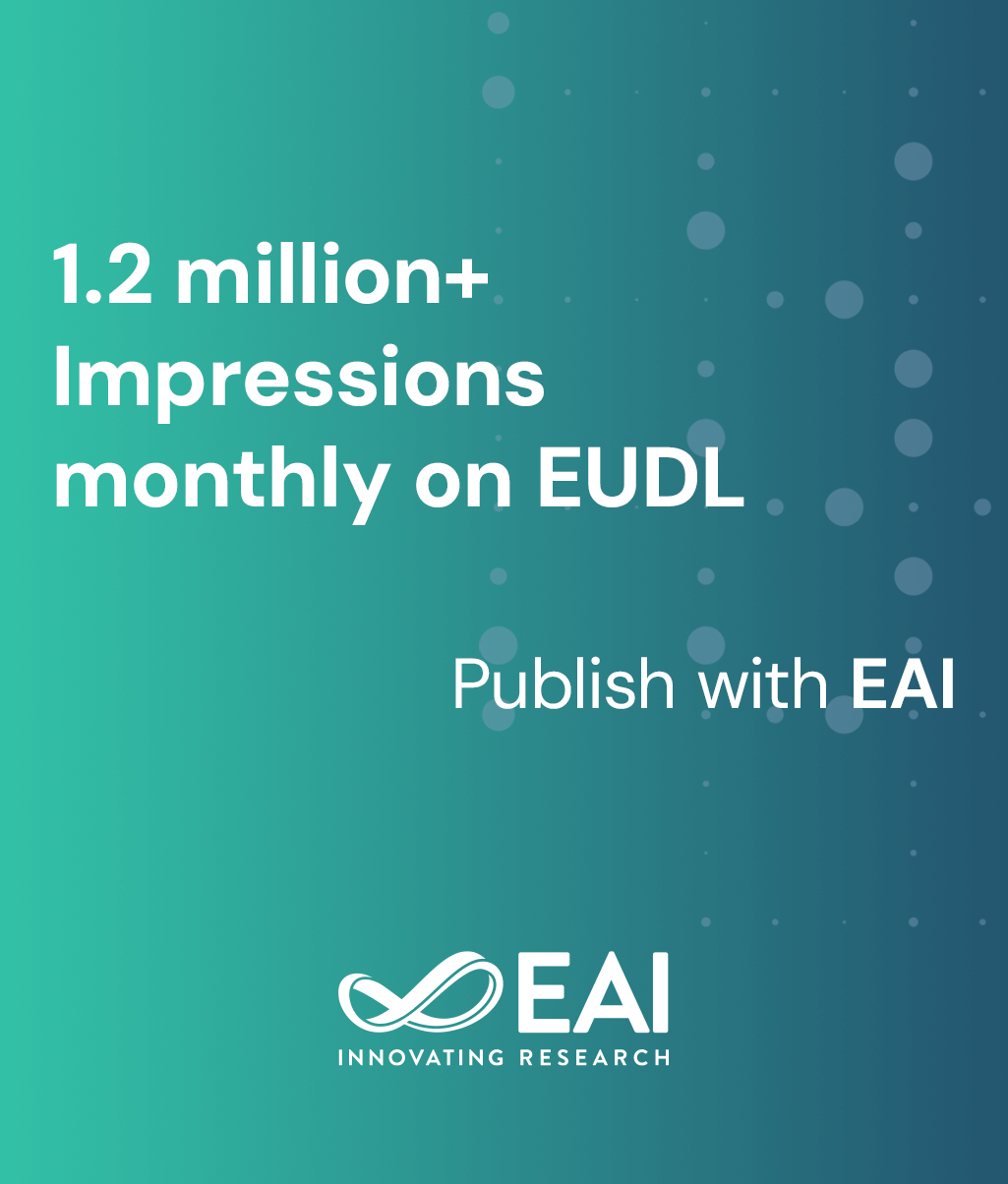
Research Article
Analysis of RAN Slicing for Cellular V2X and Mobile Broadband Services Based on Reinforcement Learning
@ARTICLE{10.4108/eai.13-7-2018.163841, author={Haider Daami R. Albonda and J. P\^{e}rez-Romero}, title={Analysis of RAN Slicing for Cellular V2X and Mobile Broadband Services Based on Reinforcement Learning}, journal={EAI Endorsed Transactions on Wireless Spectrum}, volume={4}, number={13}, publisher={EAI}, journal_a={WS}, year={2020}, month={3}, keywords={Vehicle-to-everything (V2X), reinforcement learning, network slicing, RAN slicing}, doi={10.4108/eai.13-7-2018.163841} }
- Haider Daami R. Albonda
J. Pérez-Romero
Year: 2020
Analysis of RAN Slicing for Cellular V2X and Mobile Broadband Services Based on Reinforcement Learning
WS
EAI
DOI: 10.4108/eai.13-7-2018.163841
Abstract
Radio Access Network (RAN) slicing is one of the key enablers to provide the design flexibility and enable 5G system to support heterogeneous services over a common platform (i.e., by creating a customized slice for each service). In this regard, this paper provides an analysis of a Reinforcement Learning (RL)-based RAN slicing strategy for a heterogeneous network with two generic services of 5G, namely enhanced mobile broadband (eMBB) and vehicle-to-everything (V2X). In particular, this paper investigates the RAN slicing by evaluating the proposed scheme under different algorithm configurations (i.e., number of actions of RL) and parameters in order to analyze the performance in terms of metrics such as RL convergence time and to demonstrate the capability of the algorithm to perform an efficient allocation of resources among slices. In addition, this study compares the results obtained by the proposed solution to those obtained with a Proportional Scheme.
Copyright © 2020 Haider Daami R. Albonda et al., licensed to EAI. This is an open access article distributed under the terms of the Creative Commons Attribution licence (http://creativecommons.org/licenses/by/3.0/), which permits unlimited use, distribution and reproduction in any medium so long as the original work is properly cited.