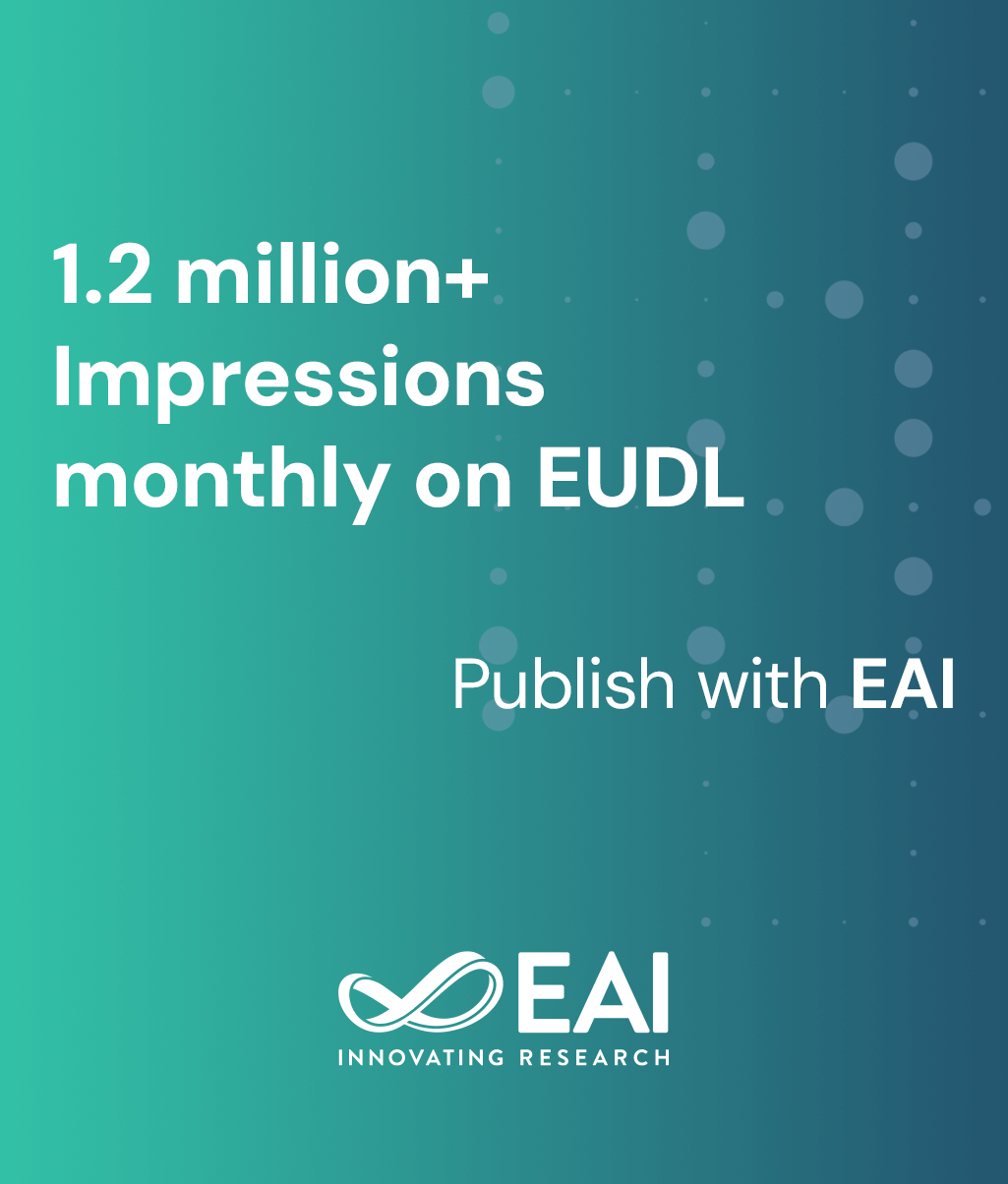
Research Article
Applying Machine Learning Techniques to Understand User Behaviors When Phishing Attacks Occur
@ARTICLE{10.4108/eai.13-7-2018.162809, author={Yi Li and Kaiqi Xiong and Xiangyang Li}, title={Applying Machine Learning Techniques to Understand User Behaviors When Phishing Attacks Occur}, journal={EAI Endorsed Transactions on Security and Safety}, volume={6}, number={21}, publisher={EAI}, journal_a={SESA}, year={2019}, month={8}, keywords={User behavior, phishing emails, machine learning, security attacks}, doi={10.4108/eai.13-7-2018.162809} }
- Yi Li
Kaiqi Xiong
Xiangyang Li
Year: 2019
Applying Machine Learning Techniques to Understand User Behaviors When Phishing Attacks Occur
SESA
EAI
DOI: 10.4108/eai.13-7-2018.162809
Abstract
Emails have been widely used in our daily life. It is important to understand user behaviors regarding email security situation assessments. However, there are very challenging and limited studies on email user behaviors. To study user security-related behaviors, we design and investigate an email test platform to understand how users behave differently when they read emails, some of which are phishing. Specifically, we conduct two experimental studies, where participants take part in our experiments on site in a lab contained environment and online through Amazon Mechanical Turk that are referred to on-site study and online study, respectively. In the two experimental studies, we design questionnaires for the two studies and use a set of emails including phishing emails from the real world with some necessary modifications for personal information protection. Furthermore, we develop necessary software tools to collect experimental data include participants’ basic background information, time measurement, mouse movement, and their answers to survey questions. Based on the collected data, we investigate what factors, such as intervention, phishing types, and an incentive mechanism, play a key role in user behaviors when phishing attacks occur. The difficulty of such investigation is due to the qualitative analysis of user behaviors and the limited number of data in the on-site study. For these reasons, we develop an approach to quantify user behavior metrics and reduce the number of user attributes by evaluating the significance of each attribute and analyzing the correlation of attributes. Moreover, we propose a machine learning framework, which contains attribute reduction, to find a critical point that classifies the performance of a participant into either ‘good’ or ‘bad’ through 10-fold cross-validation with randomly selected attributes cross-validation models. The proposed machine learning model can be used to predict the performance of a user based on the user profile. Our data analysis shows that intervention and an incentive mechanism play a significant role while phishing type I is more harmful to users compared to the other two types. The findings of this research can be used to help a user identify a phishing attack and prevent the user from being a victim of such an attack.
Copyright © 2019 Yi Li et al., licensed to EAI. This is an open access article distributed under the terms of the Creative Commons Attribution license (http://creativecommons.org/licenses/by/3.0/), which permits unlimited use, distribution and reproduction in any medium so long as the original work is properly cited.