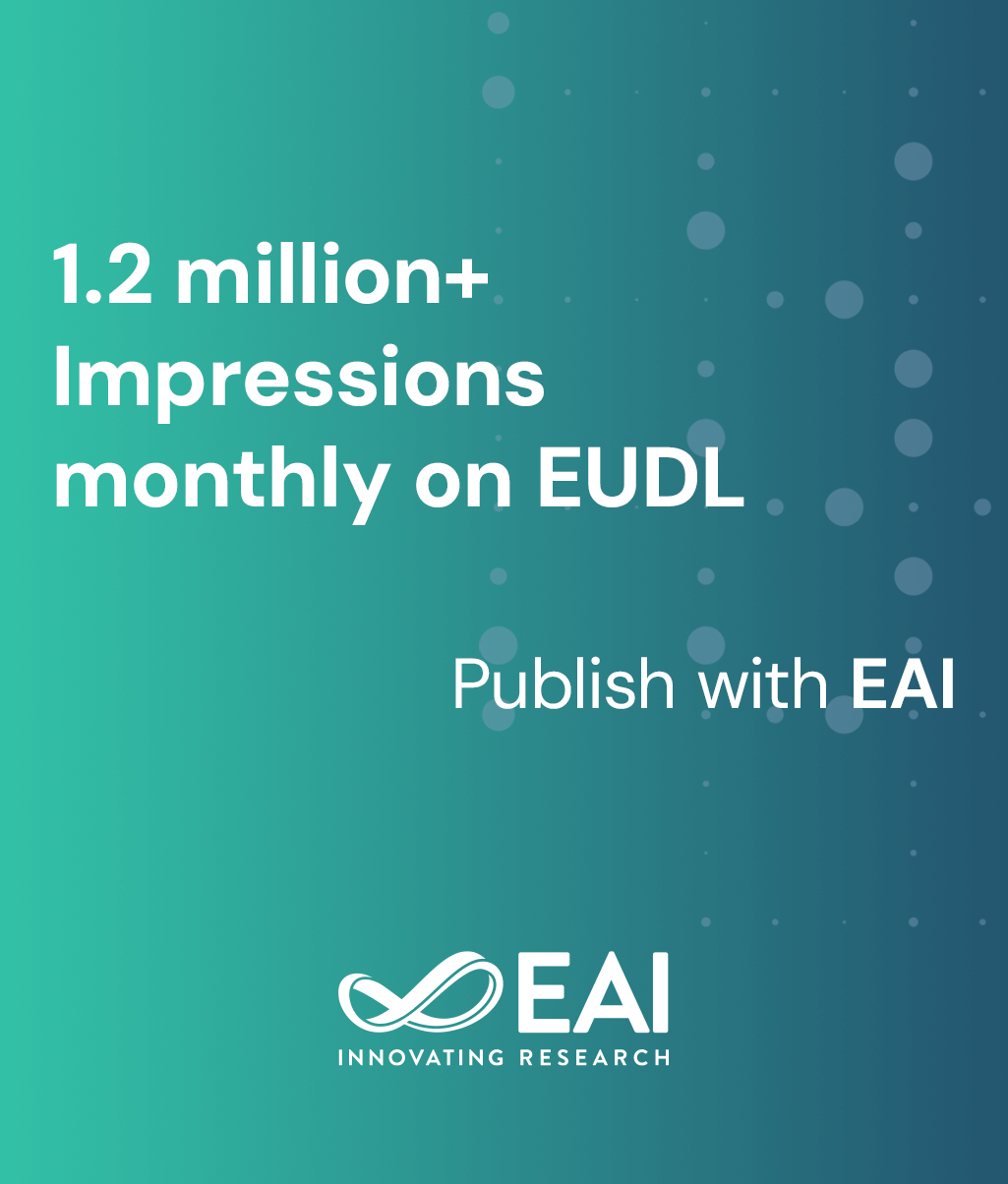
Research Article
A Pervasive Sensing Approach to Automatic Assessment of Trunk Coordination Using Mobile Devices
@ARTICLE{10.4108/eai.13-7-2018.159604, author={Zilu Liang and Yasuyuki Yoshida and Nami Iino and Takuichi Nishimura and Mario Alberto Chapa-Martell and Satoshi Nishimura}, title={A Pervasive Sensing Approach to Automatic Assessment of Trunk Coordination Using Mobile Devices}, journal={EAI Endorsed Transactions on Pervasive Health and Technology}, volume={4}, number={15}, publisher={EAI}, journal_a={PHAT}, year={2018}, month={7}, keywords={trunk coordination, health promotion, pervasive computing, inertia measurement units (IMU), accelerometer, gyroscope, machine learning}, doi={10.4108/eai.13-7-2018.159604} }
- Zilu Liang
Yasuyuki Yoshida
Nami Iino
Takuichi Nishimura
Mario Alberto Chapa-Martell
Satoshi Nishimura
Year: 2018
A Pervasive Sensing Approach to Automatic Assessment of Trunk Coordination Using Mobile Devices
PHAT
EAI
DOI: 10.4108/eai.13-7-2018.159604
Abstract
Assessing trunk coordination has many potential applications in health promotion. However, traditional bio-mechanical approaches are not suited for daily use as they require expensive devices and manual analysis. This study aimed to develop an approach for automatic classification of good and poor trunk coordination using widely available mobile devices. We investigated different combinations of sensor locations (i.e. chest and pelvis), sensing modalities (i.e. accelerometer and gyroscope) and classification techniques (i.e. SVM, KNN, and decision tree). Results showed that using both sensing modalities at chest and pelvis with SVM produced the best classification accuracy: 96% for chest rotation and 100% for pelvis rotation. In practice, however, using one device with both sensing modalities (i.e. accelerometer and gyroscope) will achieve a better trade-off between feasibility and accuracy. In this case, the device should be fixed on the chest. KNN should be selected as the classification technique for chest rotation (best accuracy 95%), and SVM should be selected as the classification technique for pelvis rotation (best accuracy 79%). Post hoc analysis found that poor coordination during chest rotation was associated to weak cross-correlation of angular velocity between chest and pelvis in the frontal plane, while poor coordination during pelvis rotation was associated to weak correlations of angular velocity between the three orthogonal components at chest. This study demonstrated how simple mobile devices can capture relevant motion data and extract key features that help construct computational models for automatic assessment of trunk coordination.
Copyright © 2018 Zilu Liang et al., licensed to EAI. This is an open access article distributed under the terms of the Creative Commons Attribution licence (http://creativecommons.org/licenses/by/3.0/), which permits unlimited use, distribution and reproduction in any medium so long as the original work is properly cited.