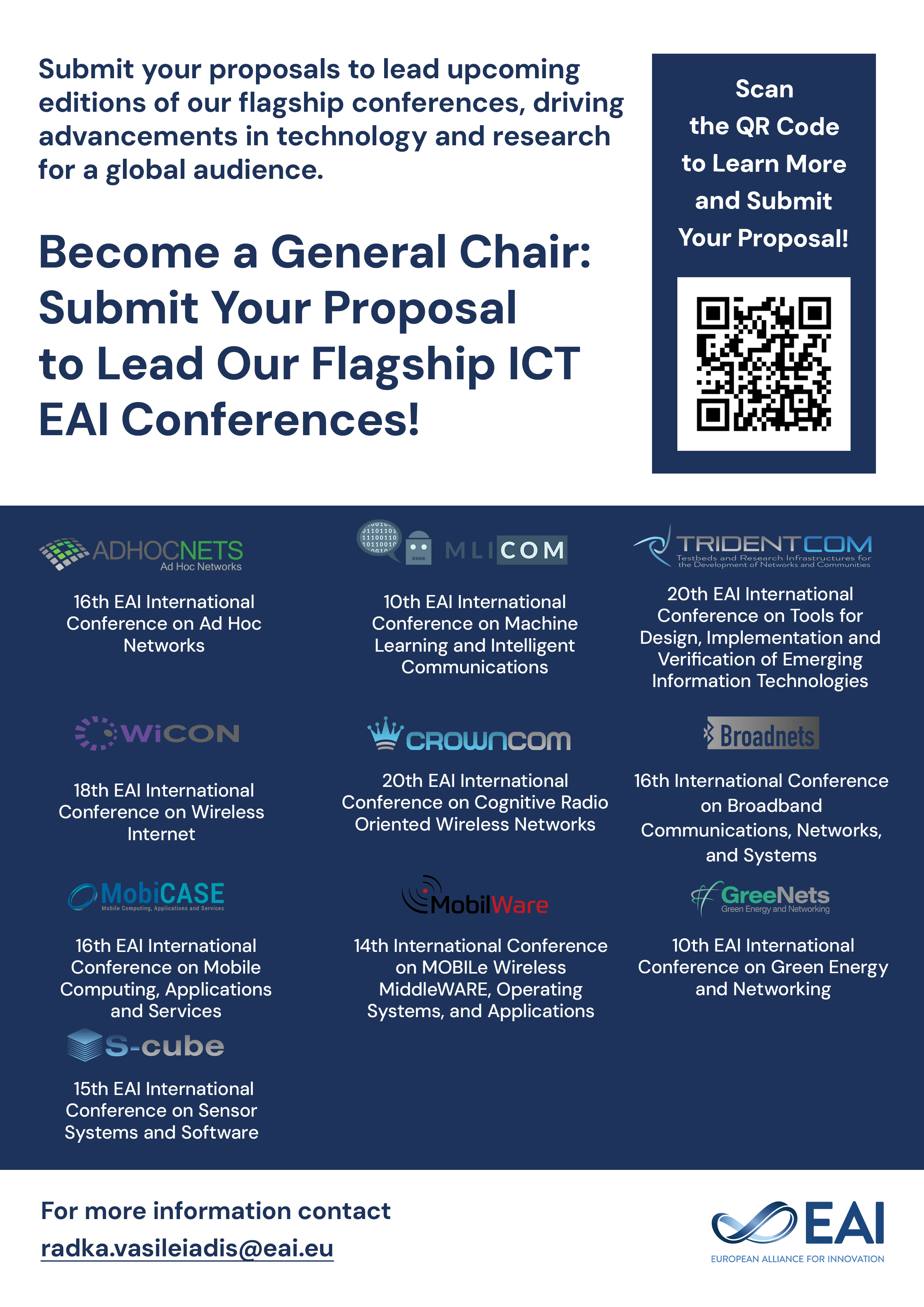
Research Article
Network-based Analysis and Classification of Malware using Behavioral Artifacts Ordering
@ARTICLE{10.4108/eai.13-7-2018.156002, author={Aziz Mohaisen and Omar Alrawi and Jeman Park and Joongheon Kim and DaeHun Nyang and Manar Mohaisen}, title={Network-based Analysis and Classification of Malware using Behavioral Artifacts Ordering}, journal={EAI Endorsed Transactions on Security and Safety}, volume={5}, number={16}, publisher={EAI}, journal_a={SESA}, year={2018}, month={12}, keywords={Malware, behavior-based analysis, classification, machine learning, n-grams}, doi={10.4108/eai.13-7-2018.156002} }
- Aziz Mohaisen
Omar Alrawi
Jeman Park
Joongheon Kim
DaeHun Nyang
Manar Mohaisen
Year: 2018
Network-based Analysis and Classification of Malware using Behavioral Artifacts Ordering
SESA
EAI
DOI: 10.4108/eai.13-7-2018.156002
Abstract
Using runtime execution artifacts to identify malware and its associated “family” is an established technique in the security domain. Many papers in the literature rely on explicit features derived from network, file system, or registry interaction. While effective, the use of these fine-granularity data points makes these techniques computationally expensive. Moreover, the signatures and heuristics are often circumvented by subsequent malware authors. In this work, we propose Chatter, a system that is concerned only with the order in which high-level system events take place. Individual events are mapped onto an alphabet and execution traces are captured via terse concatenations of those letters. Then, leveraging an analyst labeled corpus of malware, n-gram document classification techniques are applied to produce a classifier predicting malware family. This paper describes that technique and its proof-of-concept evaluation. In its prototype form only network events are considered and eleven malware families are used. We show the technique achieves 83%-94% accuracy in isolation and makes non-trivial performance improvements when integrated with a baseline classifier of combined order features to reach an accuracy of up to 98.8%.
Copyright © 2018 Aziz Mohaisen et al., licensed to EAI. This is an open access article distributed under the terms of the Creative Commons Attribution license (http://creativecommons.org/licenses/by/3.0/), which permits unlimited use, distribution and reproduction in any medium so long as the original work is properly cited.