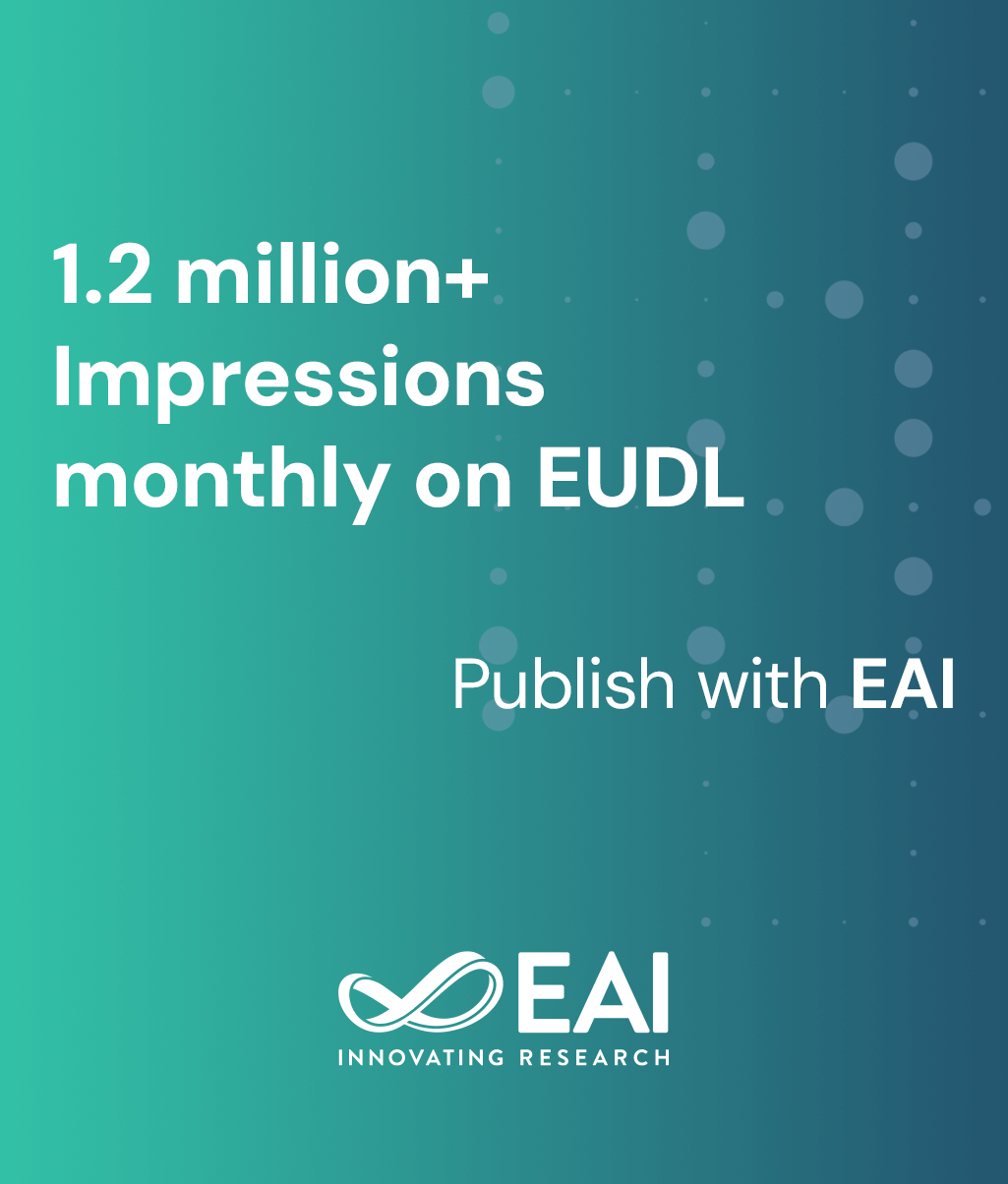
Research Article
VTWM: An Incremental Data Extraction Model Based on Variable Time-Windows
@ARTICLE{10.4108/eai.12-6-2020.166291, author={Weixing Jia and Yang Xu and Jie Liu and Guiling Wang}, title={VTWM: An Incremental Data Extraction Model Based on Variable Time-Windows}, journal={EAI Endorsed Transactions on Collaborative Computing}, volume={4}, number={14}, publisher={EAI}, journal_a={CC}, year={2020}, month={9}, keywords={change data capture, incremental data extraction, timestamp, ETL}, doi={10.4108/eai.12-6-2020.166291} }
- Weixing Jia
Yang Xu
Jie Liu
Guiling Wang
Year: 2020
VTWM: An Incremental Data Extraction Model Based on Variable Time-Windows
CC
EAI
DOI: 10.4108/eai.12-6-2020.166291
Abstract
Continuously extracting and integrating changing data from various heterogeneous systems based on an appropriate data extraction model is the key to data sharing and integration and also the key to building an incremental data warehouse for data analysis. The traditional data capture method based on timestamp changes is plagued with anomalies in the data extraction process, which leads to data extraction failure and affects the efficiency of data extraction. To address the above problems, this paper improves the traditional data capture model based on timestamp increments and proposes VTWM, an incremental data extraction model based on variable time-windows, based on the idea of extracting a small number of duplicate records before removing duplicate values. The model reduces the influence of abnormalities on data extraction, improves the reliability of the traditional data extraction ETL processes, and improves the data extraction efficiency.
Copyright © 2020 Weixing Jia et al., licensed to EAI. This is an open access article distributed under the terms of the Creative Commons Attribution license, which permits unlimited use, distribution and reproduction in any medium so long as the original work is properly cited.