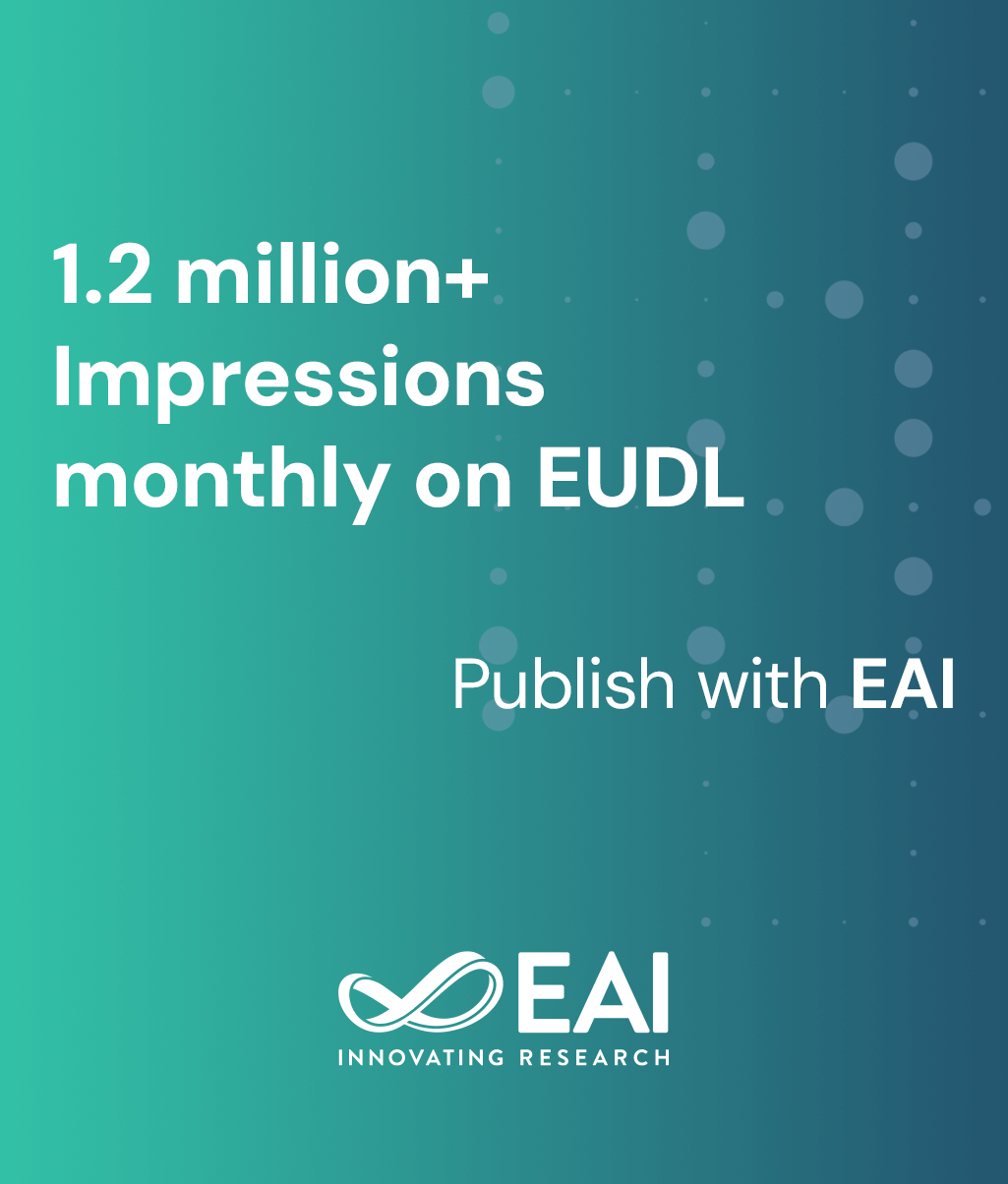
Research Article
Integrated RFE-XGBoost Credit Risk Prediction for SMEs Using Multi-Source Heterogeneous Big Data
@INPROCEEDINGS{10.4108/eai.12-1-2024.2347291, author={Yuwen Zeng and Juan He and Jun Ren and Xingyu Liu}, title={Integrated RFE-XGBoost Credit Risk Prediction for SMEs Using Multi-Source Heterogeneous Big Data}, proceedings={Proceedings of the 3rd International Conference on Big Data Economy and Digital Management, BDEDM 2024, January 12--14, 2024, Ningbo, China}, publisher={EAI}, proceedings_a={BDEDM}, year={2024}, month={6}, keywords={credit risk prediction; multi-source heterogeneous big data; text mining; rfe-xgboost}, doi={10.4108/eai.12-1-2024.2347291} }
- Yuwen Zeng
Juan He
Jun Ren
Xingyu Liu
Year: 2024
Integrated RFE-XGBoost Credit Risk Prediction for SMEs Using Multi-Source Heterogeneous Big Data
BDEDM
EAI
DOI: 10.4108/eai.12-1-2024.2347291
Abstract
This paper addresses the challenge of credit risk prediction in the financing of SMEs, focusing on 716 listed manufacturing SMEs from 2018 to 2022. A predictive model based on RFE and XGBoost was developed by integrating multi-source heterogeneous data, including key indicators such as ESG scores, public sentiment, and litigation records. The results indicate that after applying RFE, the average F1 score of XGBoost improved from 0.8822 to 0.9147, an enhancement of approximately 3.25%. This improvement is about 4.75% higher than the next best model (Random Forest, with an average F1 score of 0.8732). These findings underscore the significant role of multi-source heterogeneous data in credit risk management and provide financial institutions with a more advanced tool for risk assessment.