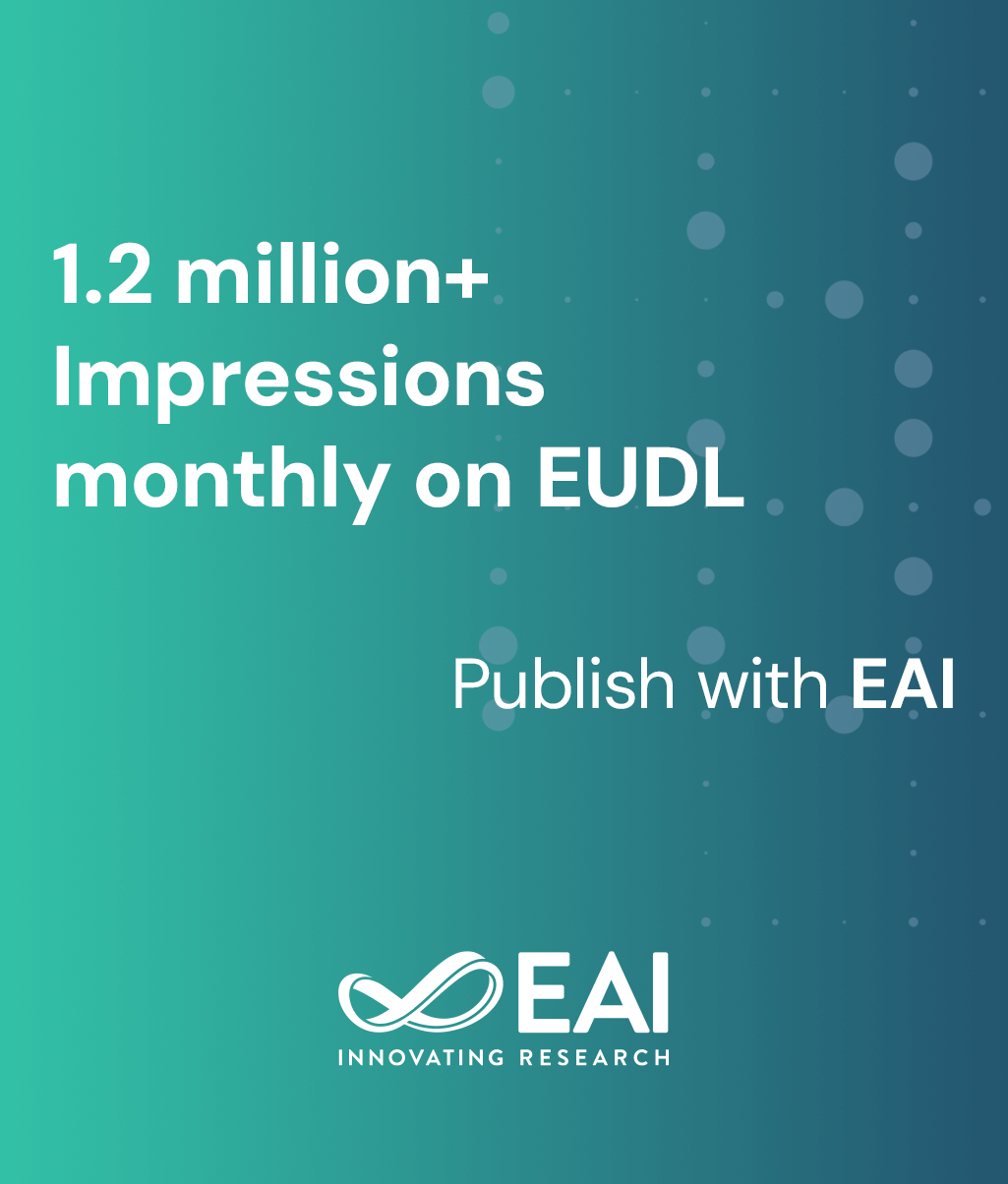
Research Article
Activity Prediction of Construction Enterprise based on Semi-supervised Learning
@INPROCEEDINGS{10.4108/eai.12-1-2024.2347236, author={Jinhao Zhong and Zhen Yu and Xinyu Yao}, title={Activity Prediction of Construction Enterprise based on Semi-supervised Learning}, proceedings={Proceedings of the 3rd International Conference on Big Data Economy and Digital Management, BDEDM 2024, January 12--14, 2024, Ningbo, China}, publisher={EAI}, proceedings_a={BDEDM}, year={2024}, month={6}, keywords={construction enterprise activity; self-organizing mapping network; gaussian mixture model; random forest}, doi={10.4108/eai.12-1-2024.2347236} }
- Jinhao Zhong
Zhen Yu
Xinyu Yao
Year: 2024
Activity Prediction of Construction Enterprise based on Semi-supervised Learning
BDEDM
EAI
DOI: 10.4108/eai.12-1-2024.2347236
Abstract
In this paper, the idea of semi-supervised learning is used to propose a method for predicting the activity of construction enterprises based on the SOM-GMM-RF model. This prediction method can help enterprises understand their activity and operation status and make reasonable decisions. The goal is to address the issue that the cost of data annotation is too high in this study, and the prediction effect of the classification model is unsuitable under the condition of only a small amount of annotated data. According to the experimental data, the random forest model trained using the methodology in this study achieves an accuracy of 93.33%, which is 4.29% greater than the random forest model trained with a labeled training set. Additionally, the model has the best prediction effect in the experiment compared to other classification algorithms. The experiment reveals that the method in this paper can effectively use unlabeled data to improve the prediction effect of the model, even when only a small amount of data is labeled. This finding is important for research on predicting construction enterprise activity.